Claim Your Discount Today
Get 10% off on all Statistics homework at statisticshomeworkhelp.com! Whether it’s Probability, Regression Analysis, or Hypothesis Testing, our experts are ready to help you excel. Don’t miss out—grab this offer today! Our dedicated team ensures accurate solutions and timely delivery, boosting your grades and confidence. Hurry, this limited-time discount won’t last forever!
We Accept
Sample Distributional Analysis Homework Completed by Our Experts
We provide sample homework that reflect the quality of our work. These examples cover a variety of distributional analysis topics, such as binomial and normal distributions, to give you an idea of what to expect when you choose us. You’ll see firsthand how we approach each problem with detailed solutions and clear explanations.
Data Analysis
Data Analysis
Data Analysis
Get Affordable Distributional Analysis Homework Help Online by Statistics Experts
At StatisticsHomeworkHelper.com, we ensure that our distributional analysis homework help is accessible and affordable for all students. Our pricing structure is designed to meet the varying needs of students by offering flexible rates based on factors such as the complexity of the homework, the deadline, and any special requirements. By doing this, we allow you to only pay for what you need, with no hidden costs. Our goal is to provide top-notch, cost-effective solutions to help students excel in their distributional analysis courses. Below is an overview of our pricing model:
Homework Type | Complexity Level | Price Range ($) |
---|---|---|
Basic Descriptive Distribution Analysis | Low | 50 - 100 |
Normal Distribution Applications | Medium | 100 - 150 |
Poisson and Exponential Distribution | High | 150 - 200 |
Binomial Distribution Case Studies | Medium | 100 - 150 |
Advanced Multivariate Distribution Analysis | High | 150 - 200 |
Custom Data Distribution Modeling | Medium | 100 - 150 |
Inferential Statistical Distribution | Low | 50 - 100 |
Large-Sample Distribution Techniques | High | 150 - 200 |
- What is Distributional Analysis in Statistics?
- Can Your Experts Do My Distributional Analysis Homework on Any Topic?
- Why Should I Avail Your Online Help with Distributional Analysis Homework?
- How Our Brilliant Distributional Analysis Homework Helpers Ensure Plagiarism-Free Solutions
What is Distributional Analysis in Statistics?
In statistics, distributional analysis is the study of the distribution of data to understand its characteristics and patterns. This includes analyzing the shape, spread, central tendency, and deviations of data to draw insights about the underlying population. Distributional analysis is crucial for making informed decisions, predictions, and hypotheses in various fields like economics, engineering, and medicine.
Here are the key aspects of distributional analysis:
- Descriptive Statistics: This involves summarizing data using measures like mean, median, variance, and standard deviation to provide an overall picture of the distribution.
- Probability Distributions: Various probability models like Normal, Binomial, and Poisson distributions are used to fit data and assess how well the data conforms to a theoretical model.
- Outlier Detection: Distributional analysis helps identify extreme values or outliers that may significantly impact the results of your statistical analysis.
- Hypothesis Testing: It enables the testing of assumptions about the data, such as normality or homogeneity of variance, which is critical for accurate modeling and prediction.
- Visualization: Tools like histograms, Q-Q plots, and boxplots are employed to visually assess the distribution and identify patterns or irregularities in the data.
At StatisticsHomeworkHelper.com, we provide expert assistance with all aspects of distributional analysis, ensuring accurate results and clear interpretations to help students excel in their homework.
Can Your Experts Do My Distributional Analysis Homework on Any Topic?
At StatisticsHomeworkHelper.com, we specialize in providing top-tier distributional analysis homework help, ensuring students receive detailed and high-quality solutions for their homework. If you're wondering, “who can do my distributional analysis homework?” our team of experts is equipped to handle homework on a wide range of topics. Here are some key areas we excel in:
- Probability Distributions: Our experts craft homework covering various probability distributions, including Normal, Binomial, Poisson, and Exponential. We provide step-by-step solutions, ensuring each concept is clearly explained and applied correctly in your homework.
- Cumulative Distribution Functions (CDFs): Calculating and interpreting CDFs is a critical aspect of distributional analysis. We create homework that break down complex CDF problems, offering precise calculations and explanations that strengthen your grasp of this fundamental topic.
- Moment Generating Functions (MGFs): Our team is proficient in homework involving MGFs, showing how to derive and apply them to find moments such as mean and variance. We deliver clear, detailed solutions to ensure your homework is completed accurately and on time.
- Transformations of Random Variables: Whether it's linear transformations or more complex techniques, we excel in homework that involve transforming random variables. Our solutions simplify these challenging problems and help you understand how to handle transformations in distributional analysis.
- Joint Distributions: For homework involving the analysis of joint distributions, our experts provide comprehensive explanations and examples, covering both discrete and continuous cases. We ensure that your homework meets the highest standards of statistical accuracy.
- Central Limit Theorem (CLT): Understanding and applying the CLT is crucial in distributional analysis. We handle homework that require a deep understanding of the CLT, ensuring that your homework demonstrates the correct application of this theorem in real-world scenarios.
- Hypothesis Testing and Confidence Intervals: Our experts are skilled in handling homework related to hypothesis testing and constructing confidence intervals based on various distributions. We provide clear and concise solutions, ensuring your homework reflects a solid understanding of these statistical concepts.
- Non-parametric Distributions: We also specialize in non-parametric distributions, delivering homework that involve methods like the empirical distribution function or kernel density estimation. Our solutions cover both the theory and practical application of these techniques.
By choosing us to tackle your “do my distributional analysis homework” request, you are guaranteed expert assistance on any topic, ensuring timely completion and enhanced understanding of complex statistical concepts.
Why Should I Avail Your Online Help with Distributional Analysis Homework?
At Statistics Homework Helper, we provide expert support for students struggling with their distributional analysis homework. Our service ensures that your homework is completed accurately and delivered on time, enabling you to excel in your statistics coursework. Here are the key benefits of hiring our distributional analysis homework experts:
- Expert Assistance: Our team is made up of experienced statisticians who specialize in distributional analysis. When you use our service, you benefit from their deep knowledge and expertise, ensuring your homework are completed with precision.
- Customized Solutions: Every homework is unique, and we tailor our solutions to meet your specific needs. Our experts provide personalized assistance to ensure that your distributional analysis is perfectly aligned with your requirements.
- Timely Deliveries: We understand the importance of meeting deadlines. Our team works diligently to complete your homework on time, so you can review it and submit it before your due date.
- A+ Quality Work: We are committed to delivering top-quality homework that help you secure the best grades. Our detailed and accurate solutions will ensure you stand out in your coursework.
- 24/7 Customer Support: Our customer support team is available around the clock via live chat to assist with any questions or concerns. We are always ready to help you whenever you need it.
- Affordable Pricing: We offer competitive rates for our distributional analysis homework help, making it accessible to students without putting a strain on your budget. Our pricing ensures that you can get high-quality assistance without overspending.
By choosing our online help with distributional analysis homework service, you’ll receive expert solutions, timely deliveries, and top-notch support, all designed to help you succeed in your statistics homework.
How Our Brilliant Distributional Analysis Homework Helpers Ensure Plagiarism-Free Solutions
At StatisticsHomeworkHelper.com, our priority is delivering high-quality, original solutions for your homework. We understand the importance of academic integrity and take every step to ensure that the work we provide is 100% plagiarism-free. Our distributional analysis homework helpers are committed to crafting custom solutions that meet your specific requirements while maintaining the highest standards of originality. Here are the key measures we take to guarantee plagiarism-free solutions:
- Custom Solutions from Scratch: Our distributional analysis homework experts create each solution from scratch, tailored to the unique requirements of your homework. We never reuse or recycle old homework, ensuring that every homework submission is completely original.
- In-Depth Research: Before starting any homework, our experts conduct thorough research to ensure that they fully understand the topic and can provide accurate, relevant, and well-supported solutions. This research-driven approach ensures the work is original and insightful.
- Use of Advanced Plagiarism Detection Tools: To further guarantee originality, we run every completed homework through advanced plagiarism detection software. This ensures that any unintentional duplication is caught and corrected before delivering the final solution to you.
- Proper Citation and Referencing: When our distributional analysis homework helpers use external sources or references, they ensure proper citation and referencing in accordance with academic standards. This eliminates the risk of plagiarism and ensures that all sources are appropriately credited.
- Rigorous Quality Checks: After completing your homework, our team performs multiple quality checks to review the homework for originality, accuracy, and adherence to your requirements. This thorough process helps us deliver top-notch, plagiarism-free solutions.
By implementing these strict measures, our professional statistics homework help ensure that the work you receive is original, high-quality, and plagiarism-free, helping you achieve academic success with confidence.
Check Out the Latest from Our Blog on Distributional Analysis Techniques
Our blog is an excellent resource for students wanting to learn more about distributional analysis and statistical methods. We regularly post articles that cover a range of topics, from basic distribution types to advanced statistical applications. Our experts share insights, tips, and tutorials to help you enhance your understanding and improve your performance. Be sure to check back frequently for the latest updates in the world of statistics.
More Than 1.1K Positive Reviews with an Average Rating of 4.8/5
Our distributional analysis homework help service has consistently received high ratings and positive feedback from satisfied students. They praise us for our attention to detail, on-time delivery, and the improvements they’ve seen in their grades. We’re proud to have helped thousands of students reach their academic goals, and we continue to strive for excellence in every homework we complete. Check out some of our reviews to see how we’ve made a difference for students like you.
Meet Our 100+ Qualified Experts in Distributional Analysis
Our team is composed of highly skilled statistics professionals, specializing in various types of distributional analysis. Each expert brings years of experience and a deep understanding of statistical methods to every homework. They are dedicated to delivering top-quality solutions that help students succeed academically. With their expertise, your homework will be in excellent hands, whether it involves simple tasks like normal distribution or more complex topics like multivariate distribution analysis.
.webp)
Loretta Walk
Ph.D. in Environmental Statistics
🇬🇧 United Kingdom
Loretta Walk, from Vancouver, has a Ph.D. in Environmental Statistics from the University of British Columbia and has completed over 900 spatial statistics homework. Her expertise includes handling complex spatial data sets and providing clear, actionable insights. Dr. Martinez’s ability to simplify intricate spatial statistics concepts makes her an excellent choice for both academic and professional projects.
.webp)
Dr. Ryan Patel
Master's degree in Spatial Data Science
🇦🇺 Australia
Dr. Ryan Patel, based in Sydney, holds a Master's degree in Spatial Data Science from the University of Sydney. With over 750 completed homework, Dr. Patel has a strong background in using advanced spatial statistics tools and techniques. His practical experience and academic knowledge ensure that he provides precise and actionable insights for various spatial statistics projects.
.webp)
Dr. Julia Zhang
Master's degree in Geostatistics
🇨🇦 Canada
Dr. Julia Zhang is a spatial data analyst with a Master's degree in Geostatistics from the University of Toronto and has successfully completed over 600 homework. Her background in both academic research and practical applications equips her with a comprehensive understanding of spatial data analysis methods. Dr. Zhang is known for her meticulous approach and ability to handle a diverse range of spatial statistics tasks.
.webp)
Dr. Henry Brooks
Ph.D. in Spatial Statistics
🇦🇺 Australia
Dr. Henry Brooks holds a Ph.D. in Spatial Statistics from the University of Edinburgh and has over 10 years of experience in the field. With a remarkable track record of over 800 completed spatial statistics homework, Dr. Brooks is well-versed in both theoretical and practical aspects of spatial analysis. His expertise spans various software tools and techniques, making him a valuable asset for complex and nuanced projects.
.webp)
Zoe Martinez
Master’s degree in Data Analytics
🇦🇺 Australia
Based in Australia, Zoe Martinez holds a Master’s degree in Data Analytics and has completed more than 500 homework in fraud detection and prevention. Zoe is known for her expertise in applying data analytics to detect and prevent fraud. Her homework focus on practical solutions and effective strategies for combating fraud, providing students with a robust understanding of the field.
.webp)
Liam Patel
Masters in Statistics
🇦🇺 Australia
From the UK, Liam Patel holds a Master’s degree in Statistics and has completed over 600 homework in fraud detection and prevention. Liam is recognized for his expertise in financial fraud detection and fraud prevention technologies. His homework is characterized by their clear, actionable recommendations and adherence to current industry standards.
.webp)
Isabella Wang
Ph.D. in Information Security
🇦🇺 Australia
Isabella Wang, from Canada, has a Ph.D. in Information Security with a specialization in fraud detection and prevention. With over 650 homework completed, Isabella excels in areas such as fraud risk assessment and anti-fraud strategies. Her work integrates theoretical concepts with practical examples, ensuring a thorough understanding of fraud prevention practices.
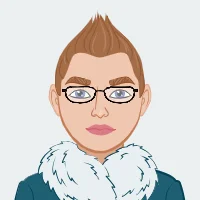
Ethan White
Master’s degree in Cybersecurity
🇦🇺 Australia
Based in the USA, Ethan White holds a Master’s degree in Cybersecurity and has completed over 750 homework in fraud detection and prevention. Ethan's expertise includes financial fraud detection techniques, anti-fraud strategies, and the implementation of fraud prevention technologies. His work is known for its depth and practical application, providing students with actionable insights into modern fraud prevention methods.
.webp)
Dr. Debra Smith
Ph.D. in Statistics
🇺🇸 United States
Dr. Debra Smith holds a PhD in Applied Statistics from the University of Toronto and has completed around 900 homework. Known for her detailed and accurate statistical analyses, Dr. Smith is proficient in tackling both standard and complex problems in categorical data analysis. Her dedication to quality and timely delivery makes her a top expert in the field.
.webp)
Dr. Richard Jones
Ph.D. in Statistics
🇺🇸 United States
Dr. Richard Jones earned his PhD in Statistical Science from the National University of Singapore and has worked on over 500 homework. His broad expertise spans various statistical methods, from basic categorical data analysis to advanced modeling techniques. His precise and detailed work makes him a great choice for high-quality solutions.
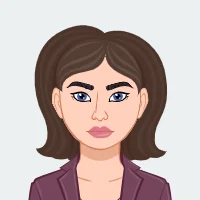
Dr. Kristen Meier
Ph.D. in Statistics
🇺🇸 United States
Dr. Kristen Meier, a PhD graduate from Emporia State University, has handled over 650 homework. With a strong background in both theoretical and applied statistics, Dr. Meier excels at providing clear, actionable insights through detailed analyses. Her expertise helps students achieve top grades with tailored, comprehensive solutions.
.webp)
Dr. Stephen Howe
Ph.D. in Statistics
🇺🇸 United States
Dr. Stephen Howe holds a PhD in Statistics from the University of Cambridge and has completed over 750 homework. His extensive experience in both academic and practical statistics ensures high-quality and insightful solutions for complex problems. Dr. Howe' deep understanding of statistical tools guarantees accurate results for every homework.
.webp)
Mia Roberts
Ph.D. in Statistics
🇺🇸 United States
Mia Roberts, a graduate of the University of Melbourne with a degree in Applied Mathematics, has worked on over 500 distributional analysis homework. Her analytical prowess and keen insight into statistical models make her a standout expert. Mia’s dedication to her work and her ability to break down complex statistical concepts into understandable terms ensure top-notch assistance for her students.
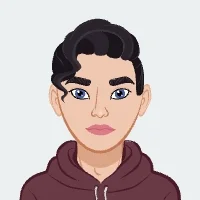
Liam Brooks
Masters in Statistics
🇺🇸 United States
Liam Brooks is a professional statistician with a Master’s degree from the University of Chicago. He has completed over 900 distributional analysis homework and is praised for his problem-solving skills and efficiency. Liam’s expertise lies in applying statistical theories to practical problems, making him adept at handling diverse homework requirements. His thorough approach ensures that each homework is handled with precision and depth.
.webp)
Jenna Collins
Ph.D. in Statistics
🇺🇸 United States
Jenna Collins is a Ph.D. candidate in Statistical Science from Harvard University with a focus on distributional analysis. With over 600 homework completed, Jenna has a robust grasp of various statistical distributions and their applications. Her analytical skills and commitment to academic excellence make her a reliable choice for students seeking expert help. Jenna's background in research and data analysis enhances her ability to provide high-quality, insightful solutions.
.webp)
Alex Turner
Masters in Statistics
🇺🇸 United States
Alex Turner holds a Master’s degree in Statistics from the University of London and has over 8 years of experience in the field. He has successfully completed more than 750 homework on distributional analysis, showcasing his deep understanding of statistical methods and analytical techniques. Alex is known for his meticulous attention to detail and his ability to simplify complex concepts for students. His extensive experience ensures that he delivers accurate and comprehensive solutions tailored to each student's needs.
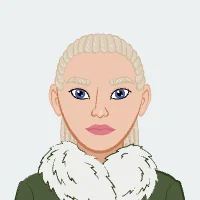
Liam Wilson
Ph.D. in Statistics
🇺🇸 United States
Liam Wilson is an expert in statistical analysis with a Ph.D. from the Australian National University and has handled over 900 exploratory data analysis homework. Liam's strong analytical skills and deep understanding of statistical theory allow him to perform detailed and high-quality analyses. His work is characterized by precision and clarity, making him a reliable choice for students seeking top-notch exploratory data analysis support.
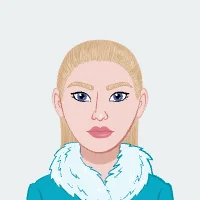
Fiona Carter
Master's degree in Statistics
🇦🇺 Australia
Fiona Carter, with a Master's degree in Statistics from the University of Melbourne, has completed over 500 exploratory data analysis homework. Her focus is on using statistical methods to uncover insights and trends in diverse datasets. Fiona’s expertise in data exploration, visualization, and interpretation ensures that students receive accurate and insightful analysis for their homework. She is known for her clear and effective communication of complex data concepts.
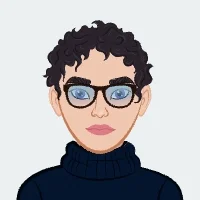
James O'Connor
Master's degree in Data Science
🇬🇧 United Kingdom
James O'Connor is a seasoned data analyst with a Master's degree in Data Science from Stanford University. With more than 600 completed homework, James excels in providing comprehensive exploratory data analysis solutions tailored to student needs. His expertise lies in analyzing large datasets and extracting meaningful patterns using modern data analytics tools and methods. James is praised for his analytical skills and ability to present complex data in an understandable format.
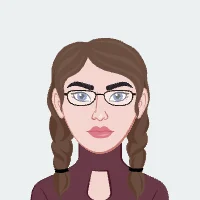
Dr. Alice Mitchell
Ph.D. in Statistics
🇦🇺 Australia
Dr. Alice Mitchell holds a Ph.D. in Statistics from the University of Cambridge and has completed over 750 exploratory data analysis homework. Her extensive academic background and practical experience make her an exceptional choice for handling complex data analysis tasks. Alice specializes in transforming raw data into actionable insights using various statistical techniques and advanced visualization tools. She is known for her meticulous attention to detail and ability to deliver high-quality work promptly.
Related Topics
Frequently Asked Questions
Got questions? Our FAQ section is here to help. We’ve answered the most common questions regarding our distributional analysis homework help, covering topics such as pricing, deadlines, and the types of homework we handle. If you don’t find the answer you're looking for, our 24/7 live chat support is always available to assist you. Explore our FAQs to learn more about how we can help you succeed in your distributional analysis course.