Claim Your Discount Today
Get 10% off on all Statistics homework at statisticshomeworkhelp.com! Whether it’s Probability, Regression Analysis, or Hypothesis Testing, our experts are ready to help you excel. Don’t miss out—grab this offer today! Our dedicated team ensures accurate solutions and timely delivery, boosting your grades and confidence. Hurry, this limited-time discount won’t last forever!
We Accept
Review Our Past Panel Data Analysis Homework for Inspiration
Step into a world of exemplary work with our collection of completed Panel Data Analysis homework. These samples showcase the high standards and meticulous attention to detail our experts bring to every project. Browse through our work to see how we tackle complex problems and gain inspiration for your own homework.
Econometrics
Econometrics
Our Flexible Pricing for All Panel Data Analysis Homework Solutions
We offer flexible pricing tailored to fit various needs. Our rates are designed to accommodate different types of Panel Data Analysis homework. We provide a free quote based on the complexity and requirements of your homework. Below is a guiding price table to give you an idea of our rates.
Homework Type | Basic | Intermediate | Advanced |
---|---|---|---|
Simple Data Analysis | $50 | $75 | $100 |
Regression Models | $60 | $85 | $120 |
Fixed/Random Effects Models | $70 | $95 | $130 |
Time-Series Analysis | $80 | $105 | $140 |
Dynamic Panel Data | $90 | $115 | $150 |
- Stressed Over Statistics? Our Panel Data Analysis Homework Help Can Save You
- We Offer Professional Help with Panel Data Analysis Homework That Covers Every Topic
- Maximize Your Grades by Hiring Our Seasoned Panel Data Analysis Homework Solvers
- Here Is How to Get Us to Do Your Panel Data Analysis Homework
- Our Panel Data Analysis Homework Help Saves You Time
Stressed Over Statistics? Our Panel Data Analysis Homework Help Can Save You
Feeling overwhelmed by your statistics homework? You're not alone. When dealing with Panel Data Analysis, the complexity of managing time-series and cross-sectional data can be challenging. Our Panel Data Analysis homework help provides the support you need, making sure your homework are completed accurately and without unnecessary stress.
We save students by offering reliable solutions, ensuring on-time delivery, and providing clarity on even the most complex problems. Our panel data analysis homework assistance is designed to take the pressure off, allowing you to focus on other important tasks while we handle your homework.
Don’t let statistics cause you unnecessary stress. Whether it’s managing multiple variables or interpreting difficult datasets, our homework help can save you from sleepless nights and missed deadlines. Let us simplify the process and get your homework completed efficiently.
We Offer Professional Help with Panel Data Analysis Homework That Covers Every Topic
Our statistics homework helper service provides thorough help with Panel Data Analysis homework, ensuring that every topic is handled with precision. Whether you're dealing with fixed or random effects models or trying to interpret complex regression results, we have the expertise to guide you through your homework and deliver accurate solutions.
We pride ourselves on offering professional assistance with Panel Data Analysis homework that covers a wide range of topics. No matter the level of complexity, our experts are equipped to handle various aspects of your homework, ensuring that all concepts are clearly explained and correctly applied.
Here are some sub-topics we have helped students with before:
- Fixed and random effects models
- Dynamic panel data models
- Time-series and cross-sectional data interpretation
- Instrumental variable estimation
- Hausman test for model selection
- GMM estimation techniques
- Variance-covariance structure analysis
Maximize Your Grades by Hiring Our Seasoned Panel Data Analysis Homework Solvers
Achieving top grades in statistics doesn’t have to be stressful. Our seasoned Panel Data Analysis homework solvers are here to help you tackle even the toughest homework with ease. By hiring our experts, you’ll benefit from clear, concise solutions that will not only complete your homework but also help you understand key concepts.
Our Panel Data Analysis homework helpers bring years of experience and knowledge to every homework. With their expertise, you can be confident that your homework will be handled efficiently and accurately, leading to higher grades and a better grasp of the subject. Our writers are skilled in a wide range of statistical methods and data models, making sure every detail is addressed.
Here are 5 qualifications that set our homework writers apart:
- Extensive experience in data analysis and statistics
- Advanced degrees in econometrics and related fields
- Proven track record of completing complex homework
- Familiarity with the latest statistical software and tools
- Ability to explain difficult concepts in simple terms
By working with our experts, you’ll be giving yourself the best chance to maximize your grades and succeed in your academic journey.
Here Is How to Get Us to Do Your Panel Data Analysis Homework
If you’re wondering how to get us to do your Panel Data Analysis homework, the process is straightforward and efficient. Start by sending us the details of your homework through our order form or by contacting our customer support team. This ensures we have all the necessary information to handle your homework effectively.
Once we receive your homework details, we’ll provide a clear and cost-effective quote based on the specifics of your work. After making the recommended initial payment, we’ll start working on your homework right away, ensuring timely completion. You will be notified once your homework is finished and ready for review.
Here’s how you can proceed:
- Submit homework details via our order form or customer support
- Receive a price quote tailored to your needs
- Make the initial payment to commence work
- Get notified when your homework is completed
- Pay the remaining balance and download your completed work
- Provide feedback and review our service
This streamlined process ensures your Panel Data Analysis homework is completed professionally and on time.
Our Panel Data Analysis Homework Help Saves You Time
Managing multiple homework and tight deadlines can be overwhelming. Our Panel Data Analysis homework help is designed to save you valuable time and reduce stress. By relying on our services, you can focus on other important tasks while we handle your complex homework.
Here’s how our help with Panel Data Analysis homework efficiently saves you time:
- We help you meet tight deadlines: Our team ensures your homework is completed on time, even when you have a looming deadline.
- Quick turnaround on complex homework: We work efficiently to tackle even the most challenging problems, so you don’t have to spend hours figuring them out.
- Reduced research time: Our experts are skilled at quickly finding and applying the right methods, cutting down the time you would spend on research.
- Efficient data analysis: We handle all aspects of your data analysis promptly, allowing you to use your time more effectively elsewhere.
- Immediate access to completed work: Once your homework is done, you receive it quickly, giving you ample time to review and submit it.
By choosing our professional statistics homework help, you streamline your workload and make better use of your time.
Explore Expert Advice and Tips in Our Panel Data Analysis Blogs
Unlock valuable insights with our Panel Data Analysis blogs. Each post is crafted to provide students with practical advice, the latest updates, and expert tips to excel in their homework. Whether you're seeking to deepen your understanding or stay current with new techniques, our blogs offer essential guidance for mastering panel data analysis.
Real Reviews from Students Who Excelled with Our Assistance
Curious about the impact of our services? Discover firsthand accounts from students who have transformed their academic performance with our help. These real reviews offer a glimpse into how our expertise in panel data analysis has made a difference, showcasing genuine experiences and success stories from those who sought our assistance.
Introducing Our Top Panel Data Analysis Homework Solvers
Meet our expert team dedicated to providing top-notch help with your Panel Data Analysis homework. Each of our professionals brings a wealth of knowledge and experience to ensure you receive accurate and timely solutions. Learn more about our accomplished experts below.
.webp)
Charlotte Warren
Ph.D. in Statistics
🇬🇧 United Kingdom
Charlotte Warren earned her Ph.D. from Dartmouth College and offers 9 years of experience in hypothesis testing. Her GRETL expertise is invaluable for students requiring thorough and accurate testing of statistical hypotheses and validating research results.
.webp)
Thomas Baldwin
Ph.D. in Statistics
🇬🇧 United Kingdom
Thomas Baldwin graduated with a Ph.D. from Virginia Polytechnic Institute and State University, with 11 years of experience in panel data analysis. His proficiency in GRETL helps students excel in handling longitudinal data and performing sophisticated statistical evaluations.
.webp)
Declan Brady
Ph.D. in Statistics
🇨🇦 Canada
Declan Brady earned his Ph.D. at Copenhagen Business School and has 15 years of experience in GRETL. His expertise lies in econometric modeling and time series analysis, providing comprehensive assistance with data forecasting and analytical techniques.
.webp)
Katie Bryant
Ph.D. in Statistics
🇬🇧 United Kingdom
Katie Bryant, a Ph.D. graduate from RWTH Aachen University, brings 18 years of experience to her role as a GRETL expert. Specializing in advanced statistical modeling and complex data analysis, Katie offers exceptional support for intricate GRETL assignments.
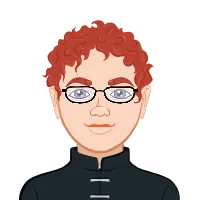
Jayden Gallagher
Ph.D.
🇩🇪 Germany
Jayden Gallagher received his Ph.D. from TU Dortmund University and has 9 years of experience in Econometrics Homework. Specializing in GARCH Models, Jayden provides expert analysis and solutions for time series data, leveraging his strong academic background and hands-on experience in econometrics.
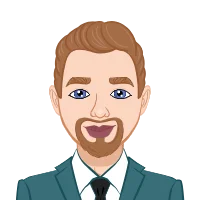
Harley Bradshaw
Ph.D.
🇺🇸 United States
Harley Bradshaw completed his Ph.D. at Virginia Polytechnic Institute and State University and boasts 11 years of experience in Econometrics Homework. His expertise in Cointegration Analysis allows him to tackle challenging econometric assignments with precision, offering expert solutions to complex data issues.
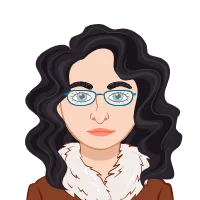
Kayleigh North
Ph.D.
🇺🇸 United States
Kayleigh North, a Ph.D. graduate from the University of Maryland, College Park, has 15 years of experience in Econometrics Homework. With a focus on Structural Equations Modeling, Kayleigh applies her deep knowledge to solve intricate econometric problems, ensuring accurate and reliable outcomes for students.

Caitlin Jackson
Ph.D.
🇩🇪 Germany
Caitlin Jackson earned her Ph.D. from RWTH Aachen University and has 18 years of experience in Econometrics Homework. Specializing in Panel Data Analysis, Caitlin brings extensive expertise to complex econometric models, offering insightful solutions and guidance based on her comprehensive academic and professional background.
.webp)
Dr. Aisha Sharma
Ph.D. in Applied Statistics
🇬🇧 United Kingdom
Dr. Sharma received her Ph.D. from the Aberystwyth University and has worked on over 320 homework. With 9 years of experience, she specializes in developing and implementing detailed volatility models for various financial applications. Her expertise in statistical and econometric analysis allows her to provide comprehensive and effective solutions. Dr. Sharma is praised for her detailed-oriented approach and ability to deliver high-quality results on time.
.webp)
Mr. Gareth Reedy
Master’s degree in Applied Mathematics
🇬🇧 United Kingdom
Mr. Reedy has a Master’s degree in Applied Mathematics from Aberystwyth University and has completed more than 300 homework. With 8 years of experience, he brings expertise in advanced volatility modeling techniques and computational finance. Mr. Reedy is adept at applying sophisticated methods to practical problems, ensuring that homework are completed with both accuracy and clarity. His analytical skills are a great asset in tackling complex financial models.
.webp)
Professor Rajesh
M.Sc. in Statistics
🇨🇦 Canada
Professor Patel earned his M.Sc. in Statistics from the University of Toronto and has completed over 400 homework. With a decade of experience, he offers exceptional support in statistical modeling and volatility analysis. His academic background combined with practical experience allows him to deliver accurate and insightful solutions. Professor Patel is known for his thorough approach to understanding and solving complex volatility problems.
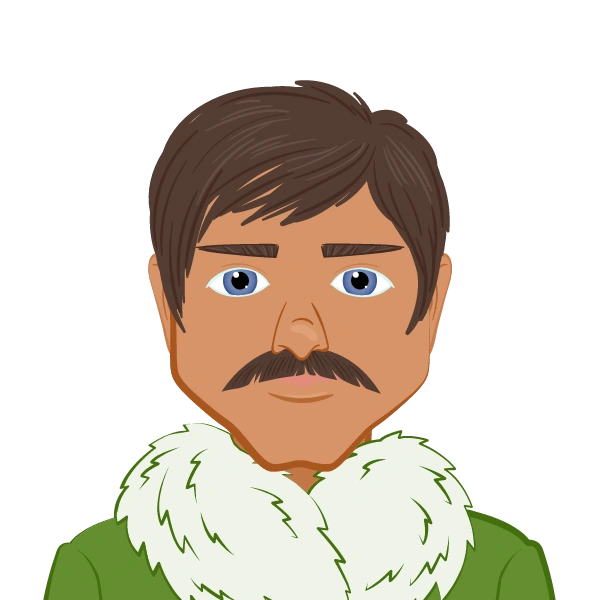
Dr. Alistair Ravenscroft
Ph.D. in Finance
🇬🇧 United Kingdom
Dr. Ravenscroft holds a Ph.D. in Finance from the University of Reading. With over 350 homework completed and 12 years of industry experience, he excels in creating and analyzing complex volatility models. His expertise ensures that each homework is handled with precision and in-depth knowledge. Dr. Ravenscroft is well-regarded for his ability to translate complex financial theories into actionable insights.
.webp)
Dr. Fiona Rhys
Ph.D. in Quantitative Analysis
🇺🇸 United States
Dr. Fiona Rhys earned her Ph.D. in Quantitative Analysis from Cardiff University. With over 970 homework completed and 7 years of experience in both academic and practical data analysis, Dr. Rhys is adept at handling a variety of statistical challenges. Her expertise ensures thorough analysis and high-quality results for all types of panel data homework.
.webp)
Mr. Liam Gallagher
Master’s in Applied Statistics
🇨🇦 Canada
Mr. Liam Gallagher holds a Master’s in Applied Statistics from McMaster University. He has completed over 720 homework and has 6 years of experience specializing in data analysis. Mr. Gallagher's hands-on approach and deep understanding of statistical methods ensure that students receive well-researched and precise solutions tailored to their specific homework needs.
.webp)
Professor Rupert Davies
degree in Statistics
🇬🇧 United Kingdom
Professor Rupert Davies earned his degree in Statistics from the University of Bristol. With more than 850 homework completed and 10 years of experience in both teaching and research, Professor Davies is well-versed in complex statistical models and data analysis. His robust knowledge and practical skills make him an invaluable resource for tackling diverse and challenging statistical problems.
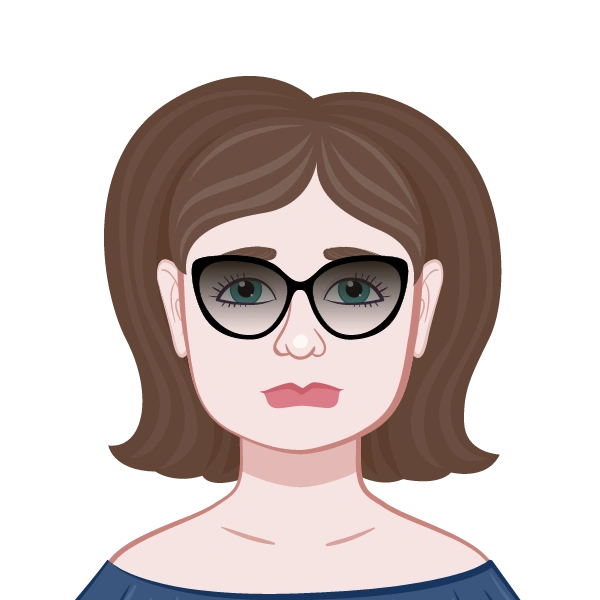
Dr. Eleanor Wexley
Ph.D. in Statistics
🇬🇧 United Kingdom
Dr. Eleanor Wexley holds a Ph.D. in Statistics from the University of Chicago and has completed over 600 homework. With 8 years of experience in both academic and applied statistics, Dr. Wexley excels in handling intricate data analysis tasks and offers in-depth understanding of panel data techniques. Her comprehensive expertise ensures that your homework is tackled with accuracy and insight.
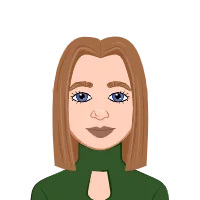
Dr. Georgina Nelson
Ph.D.
🇩🇪 Germany
Dr. Georgina Nelson earned her Ph.D. in Statistics from the University of Liverpool and has over 13 years of experience in Financial Econometrics. With an impressive record of completing over 700 Financial Econometrics homework assignments, Dr. Nelson offers unparalleled expertise and insight. Her deep knowledge and extensive experience make her a top choice for students seeking thorough and accurate econometric solutions.
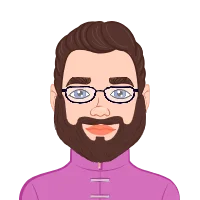
Dr. Kyle Ward
Ph.D.
🇺🇸 United States
Dr. Kyle Ward, a distinguished expert with a Ph.D. in Statistics from the University of Leeds, has accumulated over 12 years of experience in Financial Econometrics. With more than 600 completed Financial Econometrics homework assignments, Dr. Ward is renowned for his comprehensive understanding and practical application of econometric principles. His expertise provides students with reliable and effective solutions to their most complex problems.

Dr. Isobel Knowles
Ph.D.
🇺🇸 United States
Dr. Isobel Knowles holds a Ph.D. in Statistics from the University of Florida and boasts over 11 years of experience in Financial Econometrics. Having completed over 515 Financial Econometrics homework assignments, Dr. Knowles combines extensive knowledge with practical expertise. Her commitment to delivering high-quality, accurate solutions makes her an exceptional resource for students aiming to excel in their econometrics studies.
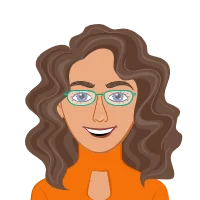
Dr. Mollie Conway
Ph.D.
🇦🇱 Albania
Dr. Mollie Conway, with a Ph.D. in Statistics from the University of Bath, brings over 10 years of experience in Financial Econometrics. Her extensive expertise is highlighted by successfully completing over 400 Financial Econometrics homework assignments. Dr. Conway's deep understanding of complex econometric models ensures precise and insightful solutions, making her a valuable asset to students seeking expert help in this challenging field.
Frequently Asked Questions
Our FAQ section provides clear answers to common questions, offering insights into what we guarantee, how we work, and the expertise of our writers. Discover how we can support your academic journey and address your concerns effectively and efficiently.