Claim Your Discount Today
Get 10% off on all Statistics homework at statisticshomeworkhelp.com! Whether it’s Probability, Regression Analysis, or Hypothesis Testing, our experts are ready to help you excel. Don’t miss out—grab this offer today! Our dedicated team ensures accurate solutions and timely delivery, boosting your grades and confidence. Hurry, this limited-time discount won’t last forever!
We Accept
- Understanding the Research Question
- Identifying the Objective
- Defining the Scope
- Formulating Hypotheses
- Designing the Experiment
- Control and Treatment Groups
- Replication and Randomization
- Variables and Measurements
- Data Collection and Management
- Accurate Data Collection
- Systematic Data Management
- Preliminary Data Analysis
- Statistical Analysis
- Descriptive Statistics
- Comparative Analysis
- Interaction Effects
- Regression Analysis
- Interpretation of Results
- Summarizing Key Findings
- Writing the Report
- Quality Assurance
- Conclusion
Phytoremediation, the process of using plants to clean up contaminated environments, is a fascinating and complex area of study. Whether you're working on a homework like the one described or tackling a different but related project, understanding the experimental design and data analysis processes is crucial. This guide provides detailed steps and strategies to help you approach such homework with confidence and expertise. It covers key aspects such as identifying research objectives clearly, designing experiments with control and treatment groups, ensuring replication and randomization for reliable results, and meticulously collecting and managing data. Additionally, it delves into the nuances of statistical analysis, from descriptive statistics and comparative tests to interpreting results effectively. By following these comprehensive guidelines, you'll be well-equipped to navigate the challenges of phytoremediation research, draw meaningful conclusions, and communicate findings effectively in your reports or academic endeavors. Whether you're a student, researcher, or professional in the field, mastering these techniques will not only enhance your understanding of phytoremediation but also contribute to impactful environmental science solutions. This guide will provide the insights and methods necessary to excel in your data analysis homework and achieve reliable results.
Understanding the Research Question
Before delving into experimental design and data analysis, it's crucial to grasp the research question fully. This involves breaking down its key components and considerations. Understanding the research question sets the foundation for the entire research process, guiding the selection of variables, experimental design, and statistical analyses. It ensures that the study remains focused and addresses the core objectives effectively. By clarifying the research question upfront, researchers can streamline their efforts, minimize potential biases, and produce findings that are relevant and insightful. This initial step is fundamental in shaping the direction and outcomes of scientific investigations.
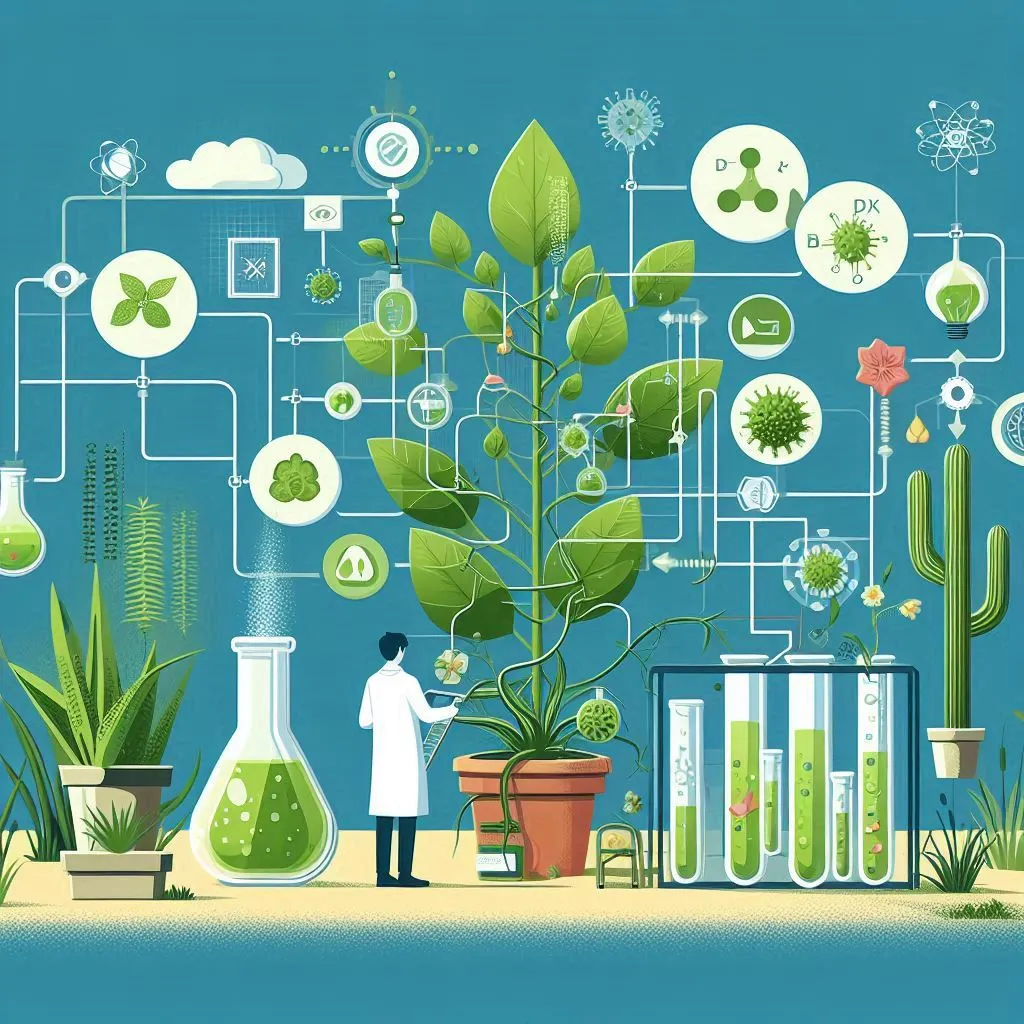
Identifying the Objective
The first step in any experimental design is to identify the primary objective of the research. In the provided homework, the goal is to determine whether Plant2 is more effective than Plant1 at sequestering heavy metals from the soil. Clarifying this objective is crucial as it sets the direction for the entire experimental process.
Defining the Scope
Understanding the scope of the research involves identifying the specific parameters and limitations of the study. For example, in the given homework, the study is conducted at 12 contaminated sites with specific treatment groups and variables. Defining the scope helps to establish clear boundaries and focus for the research.
Formulating Hypotheses
Based on the research question, formulate clear and testable hypotheses. For instance, one hypothesis might be that Plant2 is more effective than Plant1 at sequestering heavy metals from the soil. Another hypothesis could involve the impact of nitrogen fertilizer on the effectiveness of phytoremediation. These hypotheses will guide the experimental design and analysis.
Designing the Experiment
A well-designed experiment is the foundation of reliable and valid research. Here, we will explore key aspects of experimental design, including control and treatment groups, replication and randomization, and variables and measurements.
Control and Treatment Groups
Importance of Control Groups
Control groups are essential in experimental design as they provide a baseline for comparison. In the provided example, Plant1 serves as the control group because it is known to be an effective phytoremediator. This allows for a direct comparison of the effectiveness of Plant2.
Designing Treatment Groups
Treatment groups involve the variables being tested. In this case, Plant2 is the treatment group whose effectiveness is unknown. Additionally, the presence or absence of nitrogen fertilizer constitutes another treatment variable. Properly defining these groups is crucial for obtaining meaningful results.
Ensuring Comparability
To ensure comparability, it is important to standardize conditions across control and treatment groups. This includes maintaining consistent environmental conditions, such as soil type, watering, and sunlight exposure. Standardization helps to isolate the effects of the variables being tested.
Replication and Randomization
Importance of Replication
Replication involves repeating the experiment multiple times to obtain reliable data. In the provided homework, each site has four plots (two for each plant), with and without nitrogen fertilizer. Replication increases the robustness and reliability of the results.
Randomization Techniques
Randomization helps to eliminate bias and ensures that the results are generalizable. Techniques such as random homework of treatments to plots or random selection of sites help to reduce systematic errors and increase the validity of the findings.
Addressing Potential Confounding Factors
Confounding factors are variables that may influence the outcome of the experiment but are not the focus of the study. Identifying and controlling for these factors is important to ensure that the results are attributable to the variables being tested. In the provided example, soil pH is a potential confounding factor that needs to be measured and accounted for.
Variables and Measurements
Identifying Independent and Dependent Variables
Clearly defining the independent and dependent variables is crucial for experimental design. In the provided homework, the independent variables are the plant species (Plant1 and Plant2) and the presence of nitrogen fertilizer. The dependent variable is the concentration of heavy metals in the plants.
Standardizing Measurement Procedures
Consistent and accurate measurement procedures are essential for obtaining reliable data. This includes using standardized instruments and protocols for measuring heavy metal concentrations, soil pH, and other relevant variables. Proper calibration and maintenance of instruments are also important.
Collecting and Recording Data
Systematic data collection and recording are vital for the integrity of the research. This involves documenting all measurements, observations, and experimental conditions in a detailed and organized manner. Proper data management practices help to ensure that the data is complete, accurate, and easily accessible for analysis.
Data Collection and Management
Accurate and systematic data collection is essential for drawing valid conclusions from your experiment. This section explores best practices for data collection, management, and preliminary analysis.
Accurate Data Collection
Importance of Consistency
Consistency in data collection ensures that measurements are comparable across different samples and conditions. In the provided homework, this means consistently measuring heavy metal concentrations in plants at the end of the growing season and ensuring that all other variables, such as soil pH, are measured uniformly.
Avoiding Measurement Errors
Minimizing measurement errors involves using precise instruments and standardized procedures. Regular calibration and maintenance of instruments, as well as training personnel in proper measurement techniques, can help reduce errors and improve data quality.
Documenting Experimental Conditions
Documenting all relevant experimental conditions, such as weather conditions, soil characteristics, and any deviations from the planned procedures, is important for interpreting the results. This information provides context and helps to identify potential sources of variability in the data.
Systematic Data Management
Organizing Data
Organizing data in a structured and systematic manner is essential for effective analysis. This involves creating a clear and logical file structure, labeling data files appropriately, and maintaining a consistent format for data entry.
Data Cleaning
Data cleaning involves identifying and correcting errors, inconsistencies, and missing values in the dataset. This step is crucial for ensuring that the data is accurate and reliable for analysis. Techniques such as outlier detection, duplicate removal, and imputation of missing values can be used to clean the data.
Ensuring Data Security
Data security involves protecting the data from unauthorized access, loss, or corruption. This includes implementing secure storage solutions, regularly backing up the data, and restricting access to authorized personnel only. Ensuring data security is important for maintaining the integrity and confidentiality of the research.
Preliminary Data Analysis
Descriptive Statistics
Descriptive statistics provide a summary of the dataset, including measures such as mean, median, standard deviation, and range. These statistics help to understand the overall distribution and variability of the data and provide a basis for further analysis.
Data Visualization
Data visualization techniques, such as histograms, box plots, and scatter plots, can help to identify patterns, trends, and outliers in the data. Visualizing the data provides insights into the underlying relationships between variables and can guide the selection of appropriate statistical tests.
Exploratory Data Analysis
Exploratory data analysis (EDA) involves using various statistical and graphical techniques to explore the data and generate hypotheses. EDA helps to identify potential relationships and patterns that may not be immediately apparent and provides a foundation for more formal hypothesis testing.
Statistical Analysis
Statistical analysis is the core of experimental research, allowing researchers to draw meaningful conclusions from their data. This section outlines the key steps and considerations for conducting statistical analysis in experimental design and data analysis homework.
Descriptive Statistics
Summarizing Data
Begin with descriptive statistics to summarize your data. Calculate means, standard deviations, ranges, and other relevant measures for heavy metal concentrations in the plants. These statistics provide an overview of the data and help to identify any initial patterns or anomalies.
Visualizing Data Distributions
Use graphical techniques, such as histograms, box plots, and scatter plots, to visualize the distribution of your data. These visualizations help to identify the central tendency, variability, and any potential outliers in the data.
Comparing Groups
Comparing groups involves using descriptive statistics to summarize the data for different treatment groups. For example, compare the mean heavy metal concentrations between Plant1 and Plant2, as well as between plants with and without nitrogen fertilizer. These comparisons provide initial insights into the differences between groups.
Comparative Analysis
Choosing Appropriate Statistical Tests
Choosing the appropriate statistical tests depends on the research question, the type of data, and the experimental design. Common tests for comparing groups include t-tests, ANOVA, and non-parametric tests such as the Mann-Whitney U test. Ensure that the chosen tests are suitable for the data and the research question.
Conducting Hypothesis Tests
Hypothesis testing involves using statistical tests to determine whether there are significant differences between groups. For example, conduct a t-test to compare the mean heavy metal concentrations between Plant1 and Plant2. Ensure that the assumptions of the tests are met and interpret the results in the context of the research question.
Interpreting P-Values and Confidence Intervals
P-values and confidence intervals are key components of hypothesis testing. A low p-value (typically less than 0.05) indicates that there is a significant difference between groups. Confidence intervals provide a range of values within which the true population parameter is likely to fall. Interpret these results in the context of the research question and the experimental design.
Interaction Effects
Understanding Interaction Effects
Interaction effects occur when the effect of one variable depends on the level of another variable. In the provided homework, there may be an interaction between plant species and nitrogen fertilizer. Understanding these interactions is important for interpreting the results and making informed conclusions.
Using Factorial ANOVA
Factorial ANOVA is a statistical technique used to assess interaction effects between multiple independent variables. Use factorial ANOVA to analyze the interaction between plant species and nitrogen fertilizer on heavy metal concentrations. Ensure that the assumptions of the test are met and interpret the results accordingly.
Interpreting Interaction Plots
Interaction plots visually represent the interaction effects between variables. These plots help to identify and interpret the nature of the interactions, such as whether the effect of nitrogen fertilizer on heavy metal concentrations differs between Plant1 and Plant2. Use interaction plots to
complement the results of the factorial ANOVA.
Regression Analysis
Applying Regression Techniques
Regression analysis is used to model the relationship between a dependent variable and one or more independent variables. In the provided homework, use regression analysis to assess the impact of soil pH on heavy metal concentrations. Choose the appropriate regression model based on the type of data and the research question.
Interpreting Regression Coefficients
Regression coefficients represent the strength and direction of the relationship between the independent and dependent variables. Interpret these coefficients to understand the impact of soil pH on heavy metal concentrations. Consider the practical significance of the results in addition to the statistical significance.
Assessing Model Fit
Assessing the fit of the regression model involves evaluating how well the model explains the variability in the data. Use measures such as R-squared, adjusted R-squared, and residual plots to assess the model fit. Ensure that the model assumptions are met and consider alternative models if necessary.
Interpretation of Results
Interpreting the results involves synthesizing the findings and drawing meaningful conclusions. This section explores key considerations for interpreting the results and communicating them effectively.
Summarizing Key Findings
Highlighting Significant Results
Begin by summarizing the key findings of the study, focusing on the significant results. For example, if Plant2 shows significantly higher heavy metal concentrations than Plant1, highlight this finding as it directly addresses the research question.
Considering Practical Implications
Consider the practical implications of the findings, such as the cost-effectiveness and feasibility of using Plant2 for phytoremediation. Discuss the potential benefits and challenges of implementing the findings in real-world scenarios.
Addressing Limitations
Acknowledge any limitations of the study, such as potential confounding factors, measurement errors, or limitations in the experimental design. Discuss how these limitations may impact the interpretation of the results and suggest ways to address them in future research.
Writing the Report
Structuring the Report
Structure the report in a clear and logical manner, following standard scientific report formats. The report should include the following sections:
- Introduction: Provide background information and state the research question and hypotheses.
- Methods: Detail the experimental design, data collection methods, and statistical analyses.
- Results: Present the findings with appropriate tables and figures.
- Discussion: Interpret the results and discuss their implications.
- Conclusion: Summarize the key findings and suggest directions for future research.
Methods Section
The Methods section should clearly communicate all the important statistical procedures carried out in the analysis of the data. Provide sufficient detail to allow others to replicate the study, including justifications for any decisions made in the analysis. Ensure that the language used is precise and appropriate for a professional audience.
Results Section
The Results section should clearly and concisely explain the findings of the study. Use tables and figures to present the data in a clear and organized manner. Ensure that the figures and tables are properly labeled and formatted according to standard conventions.
Discussion Summary
The Discussion section should synthesize the results and state their implications. Model this section after the Discussion section of a published paper, focusing on the key findings and their relevance to the research question. Provide a concise summary that highlights the main conclusions and suggests potential applications or further research.
Quality Assurance
Proofreading and Editing
Proofread the report to correct any typos, grammatical errors, or structural issues. Clear and professional writing enhances the credibility of the report and ensures that the findings are communicated effectively.
Seeking Feedback
Seek feedback from peers, mentors, or other experts to ensure that the report is clear, accurate, and well-structured. Incorporate any constructive feedback to improve the quality of the report.
Ensuring Compliance with Guidelines
Ensure that the report complies with any guidelines or requirements specified in the homework or by the stakeholders. This includes formatting, citation style, and file naming conventions. Adhering to these guidelines is important for maintaining professionalism and meeting the expectations of the audience.
Conclusion
By following these steps and applying critical thinking throughout the process, you can effectively tackle homework in experimental design and data analysis within the field of phytoremediation or any other scientific study. This guide provides a comprehensive framework to help you navigate the complexities of such homework and achieve success.