Claim Your Discount Today
Get 10% off on all Statistics homework at statisticshomeworkhelp.com! Whether it’s Probability, Regression Analysis, or Hypothesis Testing, our experts are ready to help you excel. Don’t miss out—grab this offer today! Our dedicated team ensures accurate solutions and timely delivery, boosting your grades and confidence. Hurry, this limited-time discount won’t last forever!
We Accept
Explore Our Quality-Guaranteed Homework Samples
To give you confidence in our services, we provide sample homework solutions showcasing our work’s quality and precision. These samples offer a clear view of the level of detail and accuracy you can expect from us, helping you make an informed decision about using our services.
Data Analysis
Data Analysis
Data Analysis
How We Make Categorical Data Analysis Homework Help Affordable
We recognize that students often face financial constraints, so we are committed to offering affordable categorical data analysis homework help. Our pricing is tailored to the specifics of your homework, including its complexity, deadline, and level of expertise needed. By customizing our rates, we ensure that you receive the support you need without exceeding your budget. Here’s a snapshot of our pricing structure:
Service | Price Range |
---|---|
Basic Categorical Data Analysis | $30 - $50 |
Intermediate-Level Analysis | $50 - $80 |
Complex Categorical Analysis | $80 - $120 |
Last-Minute Assistance | $60 - $100 |
Advanced Data Analysis Projects | $100 - $150 |
- Categorical Data Analysis Homework Help
- What Sets Our Categorical Data Analysis Homework Helpers Apart?
- Do My Categorical Data Analysis Homework: Precise Solutions on Diverse Topics
- Benefits of Choosing Our Premier Help with Categorical Data Analysis Homework
- Why Students Online Categorical Data Analysis Homework Help
- How Our Categorical Data Analysis Homework Help Service Works
Categorical Data Analysis Homework Help
Balancing a busy college schedule with demanding coursework can be overwhelming, especially when tackling complex categorical data analysis homework. That’s where our categorical data analysis homework help comes in. We specialize in providing tailored solutions to ensure your homework is completed accurately and on time. Our dedicated team of experts is here to deliver top-notch solutions that meet your academic needs and help you achieve the best possible grades.
Whether you’re working with chi-square tests, logistic regression, contingency tables, or other categorical data techniques, our specialists are well-versed in the latest statistical methods and tools. We cover a broad spectrum of statistical software, including SPSS, SAS, R Programming, Python, and more. With our help, you can navigate your categorical data homework with confidence, ensuring you receive precise, high-quality solutions promptly.
What Sets Our Categorical Data Analysis Homework Helpers Apart?
At StatisticsHomeworkHelper.com, we understand that categorical data analysis requires both skill and expertise. Our team of categorical data analysis homework helpers is selected for their exceptional qualifications and experience, ensuring that you receive the best support for your homework needs. Here’s what makes our team stand out:
- Advanced Degree Holders: Our categorical data analysis homework helpers include experts with advanced degrees, such as PhDs, in statistics or related fields. Their deep understanding of statistical principles and categorical data analysis methods ensures that your homework are handled with the utmost precision and expertise.
- Specialized Categorical Data Analysts: We pride ourselves on having specialists who focus on categorical data analysis. These experts are adept at handling various types of categorical data problems, from chi-square tests to logistic regression, ensuring that your homework solutions are both accurate and insightful.
- Experienced Academics: Our team includes former professors and lecturers who have taught advanced statistics courses. Their extensive academic background equips them with the knowledge needed to produce high-quality work that meets the rigorous standards of academic coursework.
- Industry Professionals: In addition to academics, our team features industry professionals who apply categorical data analysis techniques in real-world scenarios. Their practical experience enhances the relevance and applicability of your homework solutions, bridging the gap between theory and practice.
With these qualities, our categorical data analysis homework helpers are dedicated to providing exceptional support, ensuring that you receive comprehensive, accurate, and timely solutions for your homework.
Do My Categorical Data Analysis Homework: Precise Solutions on Diverse Topics
When you find yourself asking, “Can I pay someone to handle my categorical data analysis homework on time?” our team is here to assist you. We specialize in delivering high-quality solutions for categorical data analysis homework, ensuring you get the precise help you need, exactly when you need it.
- Chi-Square Tests: Chi-square tests are essential for examining the relationship between categorical variables. Our experts excel in solving homework involving chi-square tests for independence and goodness-of-fit, providing accurate and well-explained solutions to meet your deadlines.
- Contingency Tables: Creating and interpreting contingency tables can be complex. If you need help with your homework involving cross-tabulations and marginal distributions, our team is skilled at providing clear, detailed answers that make sense of your categorical data.
- Logistic Regression: Logistic regression is used for modeling binary outcomes. Our professionals can handle your homework on logistic regression, ensuring that your analysis is thorough and that you receive high-quality work, even on short notice.
- Multinomial Logistic Regression: For homework involving outcomes with more than two categories, multinomial logistic regression is crucial. We provide expert assistance with this advanced topic, delivering comprehensive and accurate solutions for your categorical data analysis needs.
- Factor Analysis: Factor analysis is used to identify underlying relationships between variables. If you need help with homework involving factor extraction and rotation methods, our team can provide precise and insightful solutions to enhance your understanding.
- Correspondence Analysis: Correspondence analysis is a technique used to visualize the relationships between categorical variables. Our experts can deliver well-organized homework on this topic, offering clear visual representations and interpretations of your data.
- Cluster Analysis: Cluster analysis groups similar observations based on categorical data. Our professionals are adept at performing and interpreting cluster analysis, providing detailed homework that help you grasp complex concepts efficiently.
- Principal Component Analysis (PCA): While PCA is often used for continuous data, it can also apply to categorical variables through specific adaptations. Our team is ready to assist with your PCA homework, ensuring accurate application and interpretation of this technique.
For reliable and timely help with categorical data analysis homework, reach out to our experts. We’re here to provide you with the support you need to excel in your coursework.
Benefits of Choosing Our Premier Help with Categorical Data Analysis Homework
When dealing with categorical data analysis, it's essential to get precise and reliable help from experts who understand the intricacies of the subject. Our help with categorical data analysis homework at Statistics Homework Helper is designed to provide you with efficient and effective solutions tailored to your academic needs. Here's why our service stands out:
- Expert Solutions from Qualified Professionals: Our team of experienced professionals specializes in categorical data analysis. With extensive knowledge and practical experience in the field, they provide accurate and insightful solutions to even the most complex problems, ensuring your homework is handled with the highest level of expertise.
- 24/7 Support for Your Convenience: We understand that academic challenges can occur at any hour, which is why our support team is available 24/7. Whether you need urgent assistance or have specific questions about your homework, we're here to provide prompt and helpful responses at any time.
- Timely Delivery of Your Homework: Deadlines are crucial in academic settings, and our service is committed to delivering your categorical data analysis homework on time. Even with tight schedules, you can trust us to provide timely and well-crafted solutions that meet your deadlines.
- High-Quality, A+ Grade Solutions: We aim for excellence in every homework we handle. Our categorical data analysis homework help is designed to achieve the best possible grades, meeting and often exceeding your academic expectations with high-quality, detailed solutions.
- Unlimited Revisions for Your Satisfaction: Your satisfaction is our priority. We offer unlimited revisions to ensure that every aspect of your homework meets your needs. If any part of your homework requires adjustments, our experts will make the necessary changes until you're completely satisfied.
- No-Cost Rework Policy: In the rare instance that significant revisions are needed, our free rework policy ensures that you receive the required modifications at no additional cost. We're dedicated to delivering solutions that align perfectly with your homework requirements.
- Money-Back Guarantee for Peace of Mind: We are confident in the quality of our work and back it up with a money-back guarantee. If our categorical data analysis homework help does not meet your expectations, you can request a refund, knowing that your investment is secure.
Choose StatisticsHomeworkHelper.com for reliable and expert help with your categorical data analysis homework, and experience the benefits of our dedicated service firsthand.
Why Students Online Categorical Data Analysis Homework Help
At StatisticsHomeworkHelper.com, we understand that categorical data analysis homework can be particularly challenging, especially when time is running out. Students often face numerous hurdles that make it difficult to complete their homework on their own. Recognizing these challenges can help you see why turning to professional help for your categorical data analysis homework is a practical and effective solution.
- Time Constraints: With demanding schedules that include lectures, part-time jobs, and extracurricular activities, university students often find themselves overwhelmed. Balancing multiple responsibilities can leave little time for thorough completion of categorical data analysis homework, making professional help essential.
- Unexpected Events: Life can be unpredictable, with unexpected issues such as illness or personal emergencies disrupting students' ability to meet deadlines. In such cases, professional help ensures that your categorical data analysis homework is completed on time, despite any unforeseen setbacks.
- Aiming for Excellence: Despite the pressure of approaching deadlines, students strive for high academic performance. Professional assistance can turn a rushed homework into a well-crafted piece of work, meeting academic standards and helping you achieve the best possible grades.
- Managing Stress: The stress of looming deadlines can impact a student's focus and performance. Seeking expert help for categorical data analysis homework can reduce this stress, allowing students to manage their workload more effectively and maintain their overall well-being.
How Our Categorical Data Analysis Homework Help Service Works
When you need reliable and timely assistance with your categorical data analysis homework, our service is designed to provide you with the support you need. Here’s a step-by-step guide on how our categorical data analysis homework help works, ensuring you receive expert assistance precisely when you need it.
- Submit Your Homework Details: Begin by providing the specifics of your categorical data analysis homework through our straightforward online form. Include all pertinent information, such as the topic, homework requirements, deadline, and any data or materials that should be incorporated.
- Make Payment: After receiving your quote, proceed to make a secure payment through our website. We offer various payment options to facilitate a smooth and convenient transaction.
- Get Your Homework Completed by an Expert: Once your payment is confirmed, your homework is assigned to one of our qualified experts in categorical data analysis.
- Receive and Review Your Completed Homework: After your homework is completed, it will be delivered to you before the deadline. Review the work to ensure it meets your requirements.
Our categorical data analysis homework help service is designed to provide you with accurate and timely solutions, helping you achieve the best possible results.
Discover Valuable Tips & Insights on Categorical Data Analysis
Stay informed with our latest blog posts, where we share expert tips and insights on categorical data analysis. Our blog covers various topics, from basic concepts to advanced techniques, offering practical advice to help you excel in your homework. Written by our experienced statisticians, these posts are designed to enhance your understanding and provide useful strategies. Visit our blog regularly for the latest updates and valuable learning resources.
Over 1.1K Verified Reviews & Testimonials from Satisfied Students
Our commitment to excellence is reflected in the positive feedback from our clients. The numerous reviews emphasize the high quality and reliability of our categorical data analysis homework help. Students appreciate our prompt and professional service, which consistently meets their academic needs. Browse our reviews to see why we’re a trusted choice for tackling complex homework challenges.
Choose from Our Experienced Categorical Data Analysis Experts
Our team comprises highly skilled statisticians with extensive experience in categorical data analysis. Each expert brings specialized knowledge to ensure you receive exceptional assistance with your homework. From fundamental concepts to advanced analysis, our experts are equipped to handle homework of any complexity. We match each project with the most suitable expert to guarantee precise and high-quality results.
.webp)
Loretta Walk
Ph.D. in Environmental Statistics
🇬🇧 United Kingdom
Loretta Walk, from Vancouver, has a Ph.D. in Environmental Statistics from the University of British Columbia and has completed over 900 spatial statistics homework. Her expertise includes handling complex spatial data sets and providing clear, actionable insights. Dr. Martinez’s ability to simplify intricate spatial statistics concepts makes her an excellent choice for both academic and professional projects.
.webp)
Dr. Ryan Patel
Master's degree in Spatial Data Science
🇦🇺 Australia
Dr. Ryan Patel, based in Sydney, holds a Master's degree in Spatial Data Science from the University of Sydney. With over 750 completed homework, Dr. Patel has a strong background in using advanced spatial statistics tools and techniques. His practical experience and academic knowledge ensure that he provides precise and actionable insights for various spatial statistics projects.
.webp)
Dr. Julia Zhang
Master's degree in Geostatistics
🇨🇦 Canada
Dr. Julia Zhang is a spatial data analyst with a Master's degree in Geostatistics from the University of Toronto and has successfully completed over 600 homework. Her background in both academic research and practical applications equips her with a comprehensive understanding of spatial data analysis methods. Dr. Zhang is known for her meticulous approach and ability to handle a diverse range of spatial statistics tasks.
.webp)
Dr. Henry Brooks
Ph.D. in Spatial Statistics
🇦🇺 Australia
Dr. Henry Brooks holds a Ph.D. in Spatial Statistics from the University of Edinburgh and has over 10 years of experience in the field. With a remarkable track record of over 800 completed spatial statistics homework, Dr. Brooks is well-versed in both theoretical and practical aspects of spatial analysis. His expertise spans various software tools and techniques, making him a valuable asset for complex and nuanced projects.
.webp)
Zoe Martinez
Master’s degree in Data Analytics
🇦🇺 Australia
Based in Australia, Zoe Martinez holds a Master’s degree in Data Analytics and has completed more than 500 homework in fraud detection and prevention. Zoe is known for her expertise in applying data analytics to detect and prevent fraud. Her homework focus on practical solutions and effective strategies for combating fraud, providing students with a robust understanding of the field.
.webp)
Liam Patel
Masters in Statistics
🇦🇺 Australia
From the UK, Liam Patel holds a Master’s degree in Statistics and has completed over 600 homework in fraud detection and prevention. Liam is recognized for his expertise in financial fraud detection and fraud prevention technologies. His homework is characterized by their clear, actionable recommendations and adherence to current industry standards.
.webp)
Isabella Wang
Ph.D. in Information Security
🇦🇺 Australia
Isabella Wang, from Canada, has a Ph.D. in Information Security with a specialization in fraud detection and prevention. With over 650 homework completed, Isabella excels in areas such as fraud risk assessment and anti-fraud strategies. Her work integrates theoretical concepts with practical examples, ensuring a thorough understanding of fraud prevention practices.
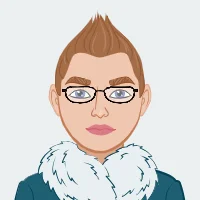
Ethan White
Master’s degree in Cybersecurity
🇦🇺 Australia
Based in the USA, Ethan White holds a Master’s degree in Cybersecurity and has completed over 750 homework in fraud detection and prevention. Ethan's expertise includes financial fraud detection techniques, anti-fraud strategies, and the implementation of fraud prevention technologies. His work is known for its depth and practical application, providing students with actionable insights into modern fraud prevention methods.
.webp)
Dr. Debra Smith
Ph.D. in Statistics
🇺🇸 United States
Dr. Debra Smith holds a PhD in Applied Statistics from the University of Toronto and has completed around 900 homework. Known for her detailed and accurate statistical analyses, Dr. Smith is proficient in tackling both standard and complex problems in categorical data analysis. Her dedication to quality and timely delivery makes her a top expert in the field.
.webp)
Dr. Richard Jones
Ph.D. in Statistics
🇺🇸 United States
Dr. Richard Jones earned his PhD in Statistical Science from the National University of Singapore and has worked on over 500 homework. His broad expertise spans various statistical methods, from basic categorical data analysis to advanced modeling techniques. His precise and detailed work makes him a great choice for high-quality solutions.
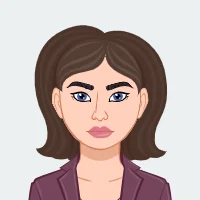
Dr. Kristen Meier
Ph.D. in Statistics
🇺🇸 United States
Dr. Kristen Meier, a PhD graduate from Emporia State University, has handled over 650 homework. With a strong background in both theoretical and applied statistics, Dr. Meier excels at providing clear, actionable insights through detailed analyses. Her expertise helps students achieve top grades with tailored, comprehensive solutions.
.webp)
Dr. Stephen Howe
Ph.D. in Statistics
🇺🇸 United States
Dr. Stephen Howe holds a PhD in Statistics from the University of Cambridge and has completed over 750 homework. His extensive experience in both academic and practical statistics ensures high-quality and insightful solutions for complex problems. Dr. Howe' deep understanding of statistical tools guarantees accurate results for every homework.
.webp)
Mia Roberts
Ph.D. in Statistics
🇺🇸 United States
Mia Roberts, a graduate of the University of Melbourne with a degree in Applied Mathematics, has worked on over 500 distributional analysis homework. Her analytical prowess and keen insight into statistical models make her a standout expert. Mia’s dedication to her work and her ability to break down complex statistical concepts into understandable terms ensure top-notch assistance for her students.
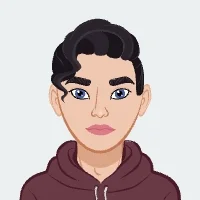
Liam Brooks
Masters in Statistics
🇺🇸 United States
Liam Brooks is a professional statistician with a Master’s degree from the University of Chicago. He has completed over 900 distributional analysis homework and is praised for his problem-solving skills and efficiency. Liam’s expertise lies in applying statistical theories to practical problems, making him adept at handling diverse homework requirements. His thorough approach ensures that each homework is handled with precision and depth.
.webp)
Jenna Collins
Ph.D. in Statistics
🇺🇸 United States
Jenna Collins is a Ph.D. candidate in Statistical Science from Harvard University with a focus on distributional analysis. With over 600 homework completed, Jenna has a robust grasp of various statistical distributions and their applications. Her analytical skills and commitment to academic excellence make her a reliable choice for students seeking expert help. Jenna's background in research and data analysis enhances her ability to provide high-quality, insightful solutions.
.webp)
Alex Turner
Masters in Statistics
🇺🇸 United States
Alex Turner holds a Master’s degree in Statistics from the University of London and has over 8 years of experience in the field. He has successfully completed more than 750 homework on distributional analysis, showcasing his deep understanding of statistical methods and analytical techniques. Alex is known for his meticulous attention to detail and his ability to simplify complex concepts for students. His extensive experience ensures that he delivers accurate and comprehensive solutions tailored to each student's needs.
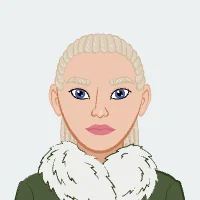
Liam Wilson
Ph.D. in Statistics
🇺🇸 United States
Liam Wilson is an expert in statistical analysis with a Ph.D. from the Australian National University and has handled over 900 exploratory data analysis homework. Liam's strong analytical skills and deep understanding of statistical theory allow him to perform detailed and high-quality analyses. His work is characterized by precision and clarity, making him a reliable choice for students seeking top-notch exploratory data analysis support.
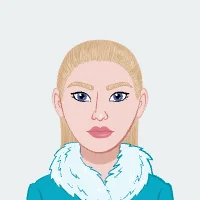
Fiona Carter
Master's degree in Statistics
🇦🇺 Australia
Fiona Carter, with a Master's degree in Statistics from the University of Melbourne, has completed over 500 exploratory data analysis homework. Her focus is on using statistical methods to uncover insights and trends in diverse datasets. Fiona’s expertise in data exploration, visualization, and interpretation ensures that students receive accurate and insightful analysis for their homework. She is known for her clear and effective communication of complex data concepts.
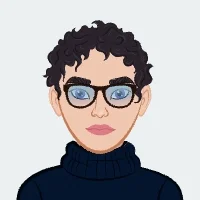
James O'Connor
Master's degree in Data Science
🇬🇧 United Kingdom
James O'Connor is a seasoned data analyst with a Master's degree in Data Science from Stanford University. With more than 600 completed homework, James excels in providing comprehensive exploratory data analysis solutions tailored to student needs. His expertise lies in analyzing large datasets and extracting meaningful patterns using modern data analytics tools and methods. James is praised for his analytical skills and ability to present complex data in an understandable format.
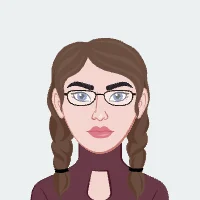
Dr. Alice Mitchell
Ph.D. in Statistics
🇦🇺 Australia
Dr. Alice Mitchell holds a Ph.D. in Statistics from the University of Cambridge and has completed over 750 exploratory data analysis homework. Her extensive academic background and practical experience make her an exceptional choice for handling complex data analysis tasks. Alice specializes in transforming raw data into actionable insights using various statistical techniques and advanced visualization tools. She is known for her meticulous attention to detail and ability to deliver high-quality work promptly.
Related Topics
Frequently Asked Questions
Have questions about our categorical data analysis homework help? Our FAQs section addresses common queries about our process, pricing, and policies. If you need more information or have specific concerns, our 24/7 live chat support is always available to assist you. Whether you have questions about our turnaround times, revision policies, or expert qualifications, our FAQs provide comprehensive answers to guide you.