Quantitative Data Analysis in SPSS: A Roadmap for Students
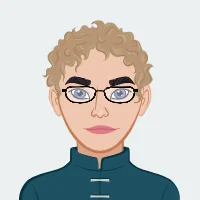
In the dynamic and constantly evolving field of academic research, the significance of quantitative data analysis is paramount. As researchers grapple with vast and complex datasets, the ability to harness the power of quantitative analysis becomes a linchpin for extracting meaningful insights. This analytical approach not only enables a deeper understanding of patterns and trends within the data but also empowers researchers to make well-informed decisions and draw accurate conclusions. Quantitative data analysis serves as a robust tool in the researcher's toolkit, offering a systematic and objective means of scrutinizing information. It goes beyond the surface-level observations, delving into the statistical intricacies that underlie patterns within datasets. Whether you need help with your SPSS homework or are simply looking to enhance your proficiency in quantitative data analysis, mastering tools like SPSS is essential for conducting rigorous and impactful research in various academic disciplines.
By employing various statistical techniques and tests, researchers can uncover relationships between variables, identify trends, and even predict future outcomes. This analytical prowess is particularly crucial in navigating the complexity of academic inquiries, where the need for precision and reliability in findings is paramount. For students venturing into the intricate realm of data analysis, the Statistical Package for the Social Sciences (SPSS) emerges as a beacon of accessibility and utility. Recognized globally as a cornerstone software for statistical analysis, SPSS caters to a wide range of users, from novices to seasoned statisticians. Its user-friendly interface, coupled with a diverse array of statistical tools, makes it an ideal choice for those seeking to delve into quantitative data analysis without being overwhelmed by complex programming languages or convoluted interfaces.
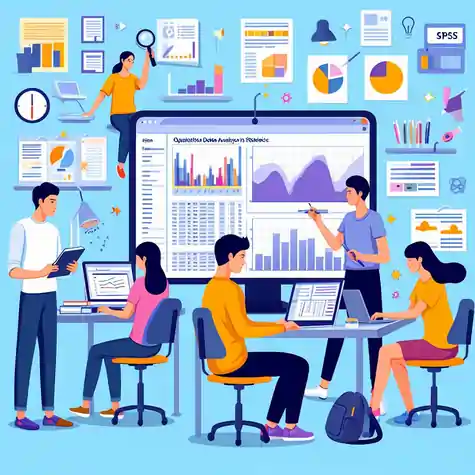
This blog endeavors to be a guiding light for students aspiring to master the art of quantitative data analysis using SPSS. By offering a comprehensive roadmap, it aims to demystify the intricacies of statistical analysis and provide a structured approach to learning and applying SPSS functionalities. The overarching goal is to empower students with the skills necessary to approach data-driven assignments with confidence and competence. The roadmap outlined in this blog spans various key facets of quantitative data analysis using SPSS. It begins by familiarizing students with the SPSS environment, ensuring they navigate the software with ease. Importantly, it emphasizes the significance of data preparation, laying the foundation for accurate and meaningful analyses. Descriptive statistics and visualization techniques are then explored, providing students with the tools to summarize and present their data effectively. Moving deeper into the world of inferential statistics, the blog introduces students to hypothesis testing and regression analysis, pivotal components of drawing valid conclusions from data. Advanced techniques like factor analysis and cluster analysis are also unveiled, opening doors to more nuanced and intricate analyses. Additionally, the blog highlights the potential for customization through SPSS syntax, allowing advanced users to streamline workflows and conduct complex analyses efficiently.
Understanding the SPSS Environment
Understanding the SPSS environment is a foundational step for any student embarking on the journey of quantitative data analysis. This section provides a comprehensive insight into two critical aspects: navigating the interface and importing/preparing data.
Navigating the Interface
Before delving into the intricacies of data analysis, it is imperative to acquaint oneself with the user-friendly SPSS interface. Designed with intuitiveness in mind, SPSS presents users with a layout comprising menus, toolbars, and a data editor. Each element serves a specific purpose, contributing to the overall ease of use for researchers and analysts.
Menus and Toolbars
SPSS boasts an array of menus and toolbars that act as gateways to its extensive functionalities. These menus, often organized categorically, offer a range of options for data manipulation, analysis, and visualization. The toolbars, strategically placed for quick access, provide shortcuts to frequently used commands. By familiarizing oneself with these menus and toolbars, users gain efficiency in navigating the software and executing tasks seamlessly.
Variable View and Data View Tabs
The 'Variable View' and 'Data View' tabs constitute the heart of the SPSS interface, providing a dynamic workspace for users. In 'Variable View,' researchers define and manage variables, specifying their types, labels, and measurement scales. This step is crucial for ensuring that the software interprets and analyzes data accurately. On the other hand, 'Data View' presents the dataset in a spreadsheet format, allowing users to input, modify, or review the actual data. Understanding the distinction and interaction between these views is fundamental for organizing and exploring datasets effectively.
Importing and Preparing Data
A robust analysis hinges on the quality of the data under scrutiny. SPSS facilitates this by offering a straightforward process for importing and preparing diverse datasets.
Importing Various File Formats
SPSS supports a multitude of file formats, including Excel, CSV, and more. Learning to import data seamlessly from these formats into SPSS is a crucial skill. This capability ensures that researchers can work with data generated from various sources, promoting versatility in analysis. As data comes in different structures, this feature enables users to adapt and integrate information seamlessly into their projects.
Preprocessing the Dataset
Preparing a dataset for analysis involves addressing several considerations. Handling missing values is a critical step in maintaining data integrity. SPSS provides tools to identify and manage missing data effectively. Checking for outliers, another essential task, involves assessing data points that deviate significantly from the norm. SPSS equips users with statistical measures and visualizations to identify and manage outliers appropriately. Additionally, transforming variables to meet specific analysis requirements is part of the preprocessing stage. This might include converting variables to different scales or creating new variables based on existing ones. A well-prepared dataset ensures that subsequent analyses are accurate and meaningful, setting the stage for informed decision-making.
Descriptive Statistics and Visualization
Descriptive statistics and data visualization are integral components of quantitative data analysis, playing a pivotal role in unraveling the intricate patterns and trends within datasets. Let's delve deeper into each aspect, exploring the significance of descriptive statistics and the art of visualization in the context of SPSS.
Descriptive Statistics
Descriptive statistics serve as the bedrock of quantitative analysis, offering a concise summary of the essential characteristics within a dataset. In the realm of SPSS, mastering these statistical measures is fundamental for any student engaging in data analysis. SPSS provides a robust set of tools designed to calculate key measures, including the mean, median, and standard deviation.
The mean, or average, is a measure of central tendency that represents the arithmetic average of all values in a dataset. It provides a quick overview of the central position of the data. The median, on the other hand, offers an alternative measure of central tendency that is less sensitive to extreme values, making it particularly useful for skewed distributions. Standard deviation, a measure of variability, indicates how spread out the values in a dataset are relative to the mean. Together, these statistics paint a comprehensive picture of the central tendency and dispersion within the data.
Data Visualization
Data Visualization emerges as a powerful companion to descriptive statistics. SPSS provides an array of graphical tools, each tailored to convey different aspects of the data. Histograms, for example, offer a visual representation of the distribution of a variable, providing insights into its shape and central tendency. This is particularly useful when dealing with continuous data, allowing researchers to discern patterns that might be less apparent in tabular form. Scatterplots, another visualization tool in SPSS, enable the exploration of relationships between two variables. By plotting points on a graph, researchers can identify patterns, trends, or potential outliers. This visual representation aids in the interpretation of correlations and associations, enhancing the depth of analysis.
Mastering the art of visualization not only facilitates a deeper understanding of the data but also serves as a powerful means of communication. Researchers often need to convey complex findings to diverse audiences, and visualizations can simplify intricate concepts. A well-crafted graph or chart can tell a compelling story, making it easier for others to grasp the essence of the data without delving into intricate statistical details.
Inferential Statistics: Unleashing the Power of Tests
Inferential statistics stands as a powerful realm within quantitative analysis, providing researchers with the tools to draw broader conclusions about populations based on sample data. This section explores two pivotal components of inferential statistics in SPSS—hypothesis testing and regression analysis.
Hypothesis Testing
At the heart of inferential statistics lies hypothesis testing, a fundamental process for researchers to make informed decisions about their data. SPSS facilitates this critical step by offering a diverse array of statistical tests. These tests, including t-tests, ANOVA (Analysis of Variance), and chi-square tests, are tailored to different research scenarios. T-tests are particularly useful when comparing the means of two groups, providing insights into whether observed differences are statistically significant. ANOVA, on the other hand, extends this comparison to multiple groups, assessing if there are significant differences among them.
Chi-square tests, often employed in categorical data analysis, help researchers understand the association between variables. Crucial to wielding these tests effectively is a deep understanding of their application. Knowing when to employ a t-test versus an ANOVA can significantly impact the accuracy and relevance of your findings. Furthermore, comprehending the nuances of interpreting p-values, confidence intervals, and effect sizes is essential for drawing meaningful conclusions from hypothesis tests.
Regression Analysis
Moving beyond hypothesis testing, regression analysis in SPSS emerges as a potent tool for researchers aiming to unravel intricate relationships between variables. Unlike descriptive statistics that merely summarize data, regression allows for predictive modeling. SPSS provides a user-friendly platform for researchers to delve into this complex analysis. Regression analysis assesses the influence of one or more independent variables on a dependent variable. This technique becomes invaluable when attempting to predict outcomes based on a set of predictors. Within SPSS, researchers navigate through regression coefficients, evaluating the strength and direction of relationships.
Assessing model fit ensures that the chosen regression model adequately represents the data, while identifying outliers becomes crucial for refining the model's accuracy. By mastering regression analysis in SPSS, researchers can unearth patterns and trends within their data, providing a deeper understanding of the factors influencing their variables of interest. Whether exploring economic trends, human behavior, or scientific phenomena, regression analysis proves indispensable for researchers seeking not only to understand but also to predict outcomes.
Advanced Techniques and Custom Analysis
In the realm of quantitative data analysis, delving into advanced techniques and custom analyses elevates researchers' capabilities to unravel intricate patterns and relationships within datasets. Two prominent tools in SPSS that facilitate this advanced exploration are Factor Analysis and Cluster Analysis.
Factor Analysis and Cluster Analysis
As researchers move beyond the basics, Factor Analysis and Cluster Analysis emerge as powerful instruments within the SPSS toolkit. Factor Analysis, a multivariate statistical method, plays a pivotal role in uncovering latent variables that may not be directly observable but influence the observed variables. This technique identifies underlying structures in the dataset, helping researchers condense complex information into a more manageable form. For instance, in social sciences, Factor Analysis might reveal latent constructs like socioeconomic status or psychological traits that contribute to observed behaviors.
Cluster Analysis, on the other hand, is instrumental in grouping similar cases based on selected variables. This method enables the identification of patterns and similarities within the data, highlighting clusters or subgroups that might share common characteristics. In marketing research, for instance, Cluster Analysis could be employed to segment customers based on their purchasing behavior, allowing businesses to tailor their strategies to specific consumer groups. These advanced techniques offer a deeper understanding of the nuances present in datasets, allowing researchers to move beyond surface-level observations.
Customizing Analysis with Syntax
For those seeking to harness the full potential of SPSS, mastering syntax becomes a game-changer. SPSS syntax refers to a series of commands written in a specialized language that allows users to automate tasks and conduct more intricate analyses than what the graphical user interface (GUI) offers. This level of customization empowers advanced users to tailor their analyses precisely to their research questions. By writing and executing syntax commands, researchers can automate repetitive tasks, ensuring consistency and reducing the likelihood of errors. For instance, if a researcher needs to perform a complex analysis on multiple datasets, using syntax allows them to create a streamlined and replicable process.
This not only saves time but also enhances the reproducibility of the analysis, a crucial aspect of robust research methodology. Moreover, delving into syntax opens the door to more sophisticated analyses that may not be readily available through the graphical interface. Users can implement complex statistical procedures, manipulate data structures, and even create customized visualizations, providing a level of flexibility that is indispensable for advanced research endeavors. While it may seem daunting initially, the efficiency gained through syntax mastery pays dividends in the form of enhanced analytical capabilities and a more nuanced understanding of the data.
Conclusion
In conclusion, mastering quantitative data analysis in SPSS is a valuable skill for students embarking on research journeys. The roadmap provided in this blog offers a structured approach to understanding the SPSS environment, conducting descriptive and inferential analyses, and exploring advanced techniques. By following this guide, students can gain confidence in tackling assignments and contribute meaningfully to the world of academic research. As technology continues to advance, proficiency in tools like SPSS becomes increasingly essential for staying ahead in the field of data analysis. Continuous practice, exploration, and a curious mindset will pave the way for students to excel in quantitative data analysis using SPSS.