Dealing with Outliers: How to Clean Your Data Set for a Statistics Homework
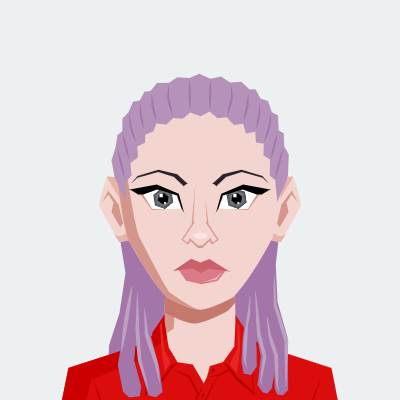
Data is the cornerstone of statistical analysis, forming the basis for drawing meaningful conclusions and making informed decisions. However, not all data is created equal. If you need help with your data analysis homework, outliers, those pesky data points that deviate significantly from the rest of the data, can throw a wrench into your analysis if not properly handled. In this blog, we'll dive into the world of outliers, understanding what they are, why they matter, and most importantly, how to effectively clean your data set for your statistics homework.
Understanding Outliers
Outliers are data points that lie far away from the central tendency of a data set, often significantly higher or lower than the rest of the data. These data points can arise due to various reasons such as measurement errors, experimental anomalies, or even genuine extreme observations. Outliers have the potential to distort statistical analyses, leading to inaccurate interpretations and misleading conclusions. Therefore, it's crucial to identify and deal with outliers appropriately.
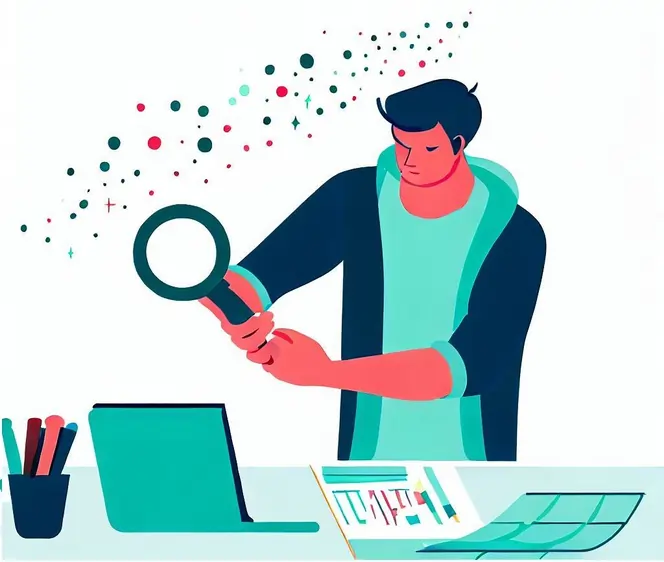
Why Outliers Matter
Outliers can exert a disproportionate influence on summary statistics like the mean and standard deviation. For instance, if you're calculating the average income in a neighborhood and a billionaire happens to live there, the mean income will be much higher than what's representative of the majority. Outliers can also impact the distribution's shape, leading to mischaracterizations of the data's underlying patterns.
Identifying Outliers
Identifying outliers is a crucial preliminary step in data analysis, as outliers can significantly impact the validity and reliability of your statistical conclusions. Outliers can distort statistical summaries and influence the interpretation of underlying data patterns. Let's delve deeper into the common methods for identifying outliers and explore their nuances.
- Visual Inspection:
- Scatter Plots: Scatter plots are particularly useful for identifying outliers in two-variable data sets. Outliers appear as data points that are distant from the bulk of the data points. If you notice isolated points far from the general trend, those might be outliers.
- Histograms: Histograms display the frequency distribution of a continuous variable. Outliers can be identified as bars that are significantly higher or lower than the majority of bars. They might create tails or spikes at the extreme ends of the histogram.
- Box Plots (Box-and-Whisker Plots): Box plots provide a graphical summary of the distribution of the data. Outliers are often displayed as individual points outside the "whiskers" of the box plot. Box plots also help in identifying skewness in the data.
- Z-Score:
- Interquartile Range (IQR):
- Domain Knowledge:
Visual inspection involves creating graphical representations of your data, such as scatter plots, histograms, and box plots. These visualizations provide an intuitive sense of the distribution of your data and help in spotting potential outliers.
Visual inspection is a quick and intuitive way to identify potential outliers, but it might not always provide precise measurements of their impact.
The Z-score is a standardized measure that quantifies how far a data point is from the mean of the dataset, in terms of standard deviations. A Z-score greater than 3 or less than -3 is often used as a threshold for identifying outliers. This method is scale-independent and works for datasets of various distributions.
The Interquartile Range (IQR) method focuses on the range between the first quartile (25th percentile) and the third quartile (75th percentile) of the data. Data points outside a defined range, typically 1.5 times the IQR, are flagged as potential outliers. This approach is particularly effective for skewed distributions.
Statistical methods are essential, but so is context. Sometimes, what might seem like an outlier could actually be a valid observation with meaningful significance. This is where domain knowledge comes into play. If you're working with data from a specific field, consulting experts who understand the domain can help you determine whether an observation is a legitimate outlier or not.
For example, in medical research, an unusually high blood pressure reading might be an outlier for the general population, but it could be normal for a certain medical condition.
Identifying outliers is an art as much as it is a science. While methods like visual inspection, z-scores, and the IQR offer systematic ways to flag potential outliers, they should be used in conjunction with domain knowledge and a deep understanding of the data you're working with. Outliers can hold valuable insights or introduce noise, and the goal is to strike a balance between identifying and acting on outliers while preserving the integrity of your analysis. Remember, accurate outlier identification is the foundation on which you'll build your data cleaning strategies for your statistics homework, ultimately leading to more accurate and meaningful results.
Dealing with Outliers
Identifying outliers in your dataset is just the first step. Once you've pinpointed these unusual data points, you'll need to decide how to handle them in a way that preserves the integrity of your analysis. Here are several strategies you can use to deal with outliers:
1. Remove Them:
One of the most straightforward approaches to dealing with outliers is to remove them from your dataset. If outliers are indeed the result of errors or anomalies, eliminating them can improve the accuracy of your analysis. However, you need to be cautious when taking this approach. Removing too many outliers can lead to a biased and skewed view of your data. Before removing any data points, consider the impact on your analysis and whether the removal aligns with the goals of your research.
2. Transform Data:
Another way to mitigate the influence of outliers is by applying mathematical transformations to your data. This approach is particularly useful when the distribution of your data is heavily skewed due to the presence of outliers. Common transformations include taking the logarithm, square root, or reciprocal of the data. These transformations can compress the range of extreme values and make the distribution more symmetric, thus reducing the impact of outliers.
3. Winsorization:
Winsorization is a technique that involves modifying extreme values to make them less extreme. Instead of removing outliers, you adjust their values to be closer to the rest of the data. For example, you might replace values above the 95th percentile with the value at the 95th percentile itself. This method ensures that extreme values still have an impact on your analysis but don't overly skew the results.
4. Use Robust Statistics:
If your data contains outliers that you believe are valid and meaningful, but you're concerned about their influence on traditional statistics, consider using robust statistical measures. These measures are less sensitive to outliers and provide a more accurate representation of central tendency and dispersion. For example, instead of using the mean, you can use the median as a measure of central tendency. Similarly, the interquartile range (IQR) can be used as a robust alternative to the standard deviation.
5. Create Categorical Variables:
In some cases, outliers may carry significant information that you don't want to lose. If you suspect that the presence of outliers is meaningful, you can create a categorical variable to indicate whether a data point is an outlier or not. This approach allows you to retain the original data while also accounting for the potential impact of outliers in your analysis. Categorizing outliers can provide a nuanced perspective on how these extreme observations affect different aspects of your study.
Dealing with outliers is a critical aspect of data analysis that requires careful consideration. The approach you choose should align with the goals of your research and the nature of your data. Removing outliers can enhance the accuracy of your analysis but should be done cautiously to avoid bias. Transforming data, winsorization, and using robust statistics provide alternatives to outright removal, allowing you to maintain the integrity of your data while managing outlier influence. Ultimately, the decision on how to handle outliers depends on your understanding of the data and the insights you aim to extract from your analysis.
Maintaining Transparency
As you engage in the process of cleaning your data for a statistics homework assignment, ensuring transparency is paramount. Documenting the steps you've taken to deal with outliers not only demonstrates your analytical rigor but also provides clarity for both yourself and others who might review your work. Here's why maintaining transparency is essential and how you can achieve it:
The Importance of Transparency
Transparency is the cornerstone of robust data analysis. It allows others to replicate and validate your findings, enhancing the credibility of your work. When it comes to outlier handling, transparency is particularly critical because outlier decisions can significantly impact the results and interpretations of your analysis. By documenting your approach to handling outliers, you provide a comprehensive view of your analytical process, making it easier for others to comprehend and critique your work.
Documenting Outlier Handling
To maintain transparency in outlier handling, consider the following steps:
- Explain Detection Methods: Start by detailing the methods you used to identify outliers. Describe whether you relied on visual inspection, Z-scores, IQR, domain knowledge, or a combination of these methods. This explanation sets the foundation for understanding the subsequent steps.
- Describe Outlier Impact: Discuss the potential impact of outliers on your data analysis. Explain how outliers could skew summary statistics, distort distributions, or influence patterns you're investigating. This shows your awareness of the significance of outliers in your analysis.
- Detail Treatment Methods: Clearly outline the approaches you employed to deal with outliers. If you chose to remove outliers, explain your rationale and provide insights into how many data points were affected. If you opted for transformations, winsorization, or robust statistics, elaborate on the reasoning behind your choices.
- Include Visuals: Visual aids, such as annotated plots or histograms, can help convey your outlier handling process more effectively. Visuals provide a clear representation of the changes you made and the resulting impact on the data.
- Quantify Impact: Whenever possible, quantify the impact of outlier handling on your analysis. For instance, if removing outliers led to a significant change in the mean or standard deviation, document these changes. This provides a concrete measure of how your decisions influenced the results.
- Discuss Sensitivity Analysis: Consider discussing how sensitive your analysis is to outlier handling decisions. Explore what happens to your results when you apply different outlier treatment strategies. This demonstrates that you've considered the robustness of your conclusions.
- Address Limitations: Acknowledge any limitations or uncertainties in your outlier handling methods. Highlight areas where your choices might be subjective or where assumptions were necessary.
Benefits of Transparent Documentation
Maintaining transparency in your outlier handling documentation offers several benefits:
- Reproducibility: Others can replicate your analysis with confidence, knowing the steps you took to address outliers.
- Peer Review: Transparent documentation invites constructive feedback from peers, enhancing the quality of your analysis.
- Credibility: Transparent handling of outliers establishes your credibility as a diligent and conscientious data analyst.
- Learning Opportunity: Documenting your outlier handling process is an opportunity for you to reflect on your choices and learn from the experience.
Conclusion
Dealing with outliers is a critical step in the data analysis process. Ignoring outliers or mishandling them can lead to skewed results and misguided conclusions. By understanding what outliers are, why they matter, and how to effectively identify and deal with them, you can ensure that your statistics homework is based on a clean and reliable data set, leading to more accurate and insightful analyses.