Data Presentation and Summarization
For this homework, you will be examining a selection of data from the fictitious company, Burger Kong. The data you will be using is available in the file “bksales.xlsx” posted in the Labs module on Canvas. You may use the Minitab software for data analysis, which is available for download provided by the college; see Canvas for details.
1. Download the “bksales.xlsx” file, and take a look at it on your computer. The first column of data, “Gross Sales”, describes Burger Kong’s total sales at each location for the month of May. We will be using this as our response variable; we will examine the other data to determine which, if any, may work as an explanatory variable in our model.
First, use the Gross Sales variable to calculate the mean, standard deviation, and 5-number summary. Comment on the shape of the distribution (no need to include a graph)
2. Next, examine the data in each of the columns for Population (the local population of the area), Average Income (average annual income of the area), Average Age (average age in the area), and Advertising (dollars spent on advertising in the previous month). Compute the mean and standard deviation for each variable.
3. Thinking qualitatively – is there any variable given that is likely to be a better explanatory variable for our response variable of Gross Sales than the other? Why or why not?
Regressions
4. Now, using your chosen statistical software, create a scatterplot using Population as the explanatory variable on the x-axis, and Gross Sales as the response variable on the y-axis. Comment on the shape of the scatterplot. Does the data show a strong or weak association? Is it a linear or nonlinear shape? Are there any outliers or influential points?
5. Run a Linear Regression with Gross Sales as your left-hand y-variable and Population as the x-variable on the right hand side. Give the equation, the correlation coefficient, and the R-squared value. Does this appear to be a useful regression equation?
6. Repeat steps 4 and 5, drawing a scatterplot, commenting on its appearance, running a linear regression and recording and commenting on the results, this time using Average Income as the explanatory variable, with Gross Sales as the response variable.
7. Repeat steps 4 and 5, drawing a scatterplot, commenting on its appearance, running a linear regression and recording and commenting on the results, this time using Average Age as the explanatory variable, with Gross Sales as the response variable.
8. Repeat steps 4 and 5, drawing a scatterplot, commenting on its appearance, running a linear regression and recording and commenting on the results, this time using Advertising as the explanatory variable, with Gross Sales as the response variable.
Data Analysis
9. Examine the results of your previous regressions. Which do you think is the best regression to use for predicting future sales values? Explain your choice of answer. (Note: there may be more than one right answer, depending on your explanation – be sure to justify your choice!) Your explanation should include both mathematical reasoning related to the data, and practical reasoning about the real-life situation the data describes.
10. Graph the Residuals plot for your chosen regression, and sketch the graph below or attach the image file. Can you draw any conclusions about your data from examining its residuals?
11. Suppose a new location opens in an area with a population of 144,000, an average income of $36,000, an average age of 27, and $2,000 spent on advertising in the previous month.
a. Use your chosen regression to predict Gross Sales for the month at the new location.
b. Suppose actual Gross Sales for the month were $420,624. Does this make sense, given your model and predicted value?
12. Using your chosen regression model,
a. Identify the slope and explain what it means, in the context of the model.
b. Identify the initial value or y-intercept and explain what it means, in the context of the model.
13. Take a step back, and look at the data and the different regression and correlation values you’ve found. Is there anything you can think of that may result in an improved model over the one you’ve found? Do you have any suggestions for future research that may be done, or data that may be collected, to make more accurate predictions in the future?
Solution
1.
Summary statistics for Gross sales | ||
5-number summary | Minimum | 73280 |
Q1 | 157673 | |
Median | 247360 | |
Q3 | 311212 | |
Maximum | 979810 | |
Mean and Std. Dev. | Mean | 273430.4 |
Std. Dev. | 186351.5 |
The mean, standard deviation as well as the 5-number summary of Gross sales are presented in the table above. It can be inferred from the summary statistics that the distribution is positively skewed.
2.
Variables | Mean | Std. Dev |
Population | 62452 | 95780.1839 |
Average Income | 36839.68 | 18996.9965 |
Average Age | 39.08 | 11.8810774 |
Advertising | 3232 | 6215.82121 |
3.
Population will be a better explanatory variable for the response variable Gross Sales than the remaining three variables because it is the most correlated variable with Gross Sales.
4.
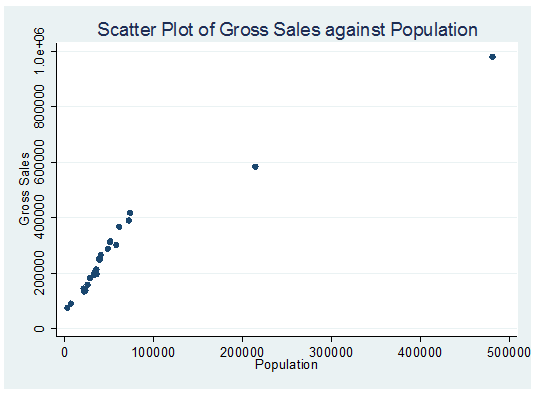
From the graph plotted, it is clear that the variables have a strong and positive association. There is also an evidence of linear relationship between the variables. However, there are two outliers in the dataset as indicated on the scatter plot.
5.
Gross Sales = 158404.5 + 1.841834*Population

The coefficient of determination is reasonable enough, hence the model is adequate and useful.
6.
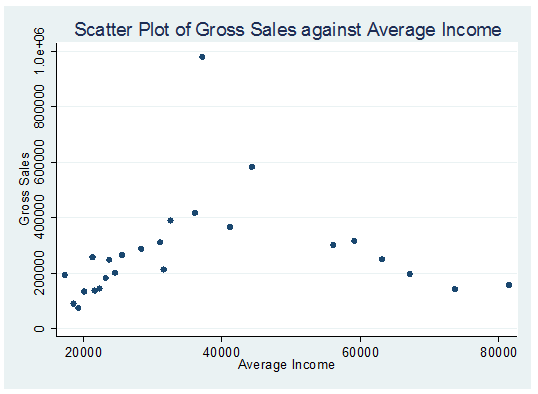
From the graph plotted, it is clear that the variables have a positive but weak association. There is no evidence of linear relationship between the variables. However, there is one outlier in the dataset as indicated on the scatter plot.
Gross Sales = 237843.7+ 0.9659872*Average_Income

The coefficient of determination is too small; hence the model is not adequate.
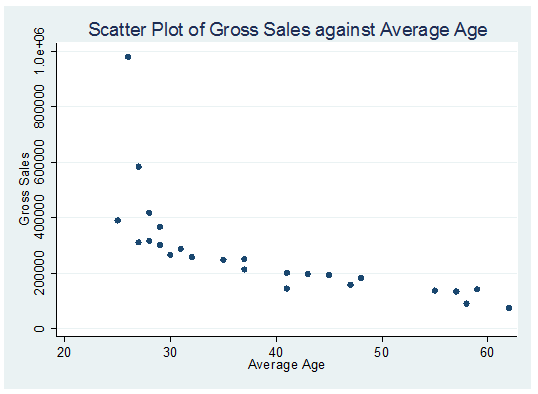
From the graph plotted, it is clear that the variables have a strong and negative association. There is also an evidence of linear relationship between the variables. However, there is only one outliers in the dataset as indicated on the scatter plot.
Gross Sales = 692430.7 -10721.6*Average_Age

The coefficient of determination is reasonable enough, hence the model is adequate and useful.
8.
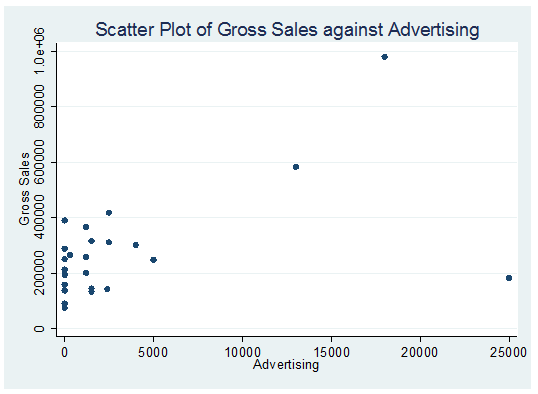
From the graph plotted, it is clear that the variables have a weak and positive association. There is no evidence of linear relationship between the variables. However, there are three outliers in the dataset as indicated on the scatter plot.
Gross Sales = 223863.5 + 15.33631*Advertising

The coefficient of determination is small, but the model is fairly adequate and useful.
Selecting Best Model(s)
From the four simple regressions conducted, three of the models are significant. population is the best predictor since it has the highest coefficient of determination. Also, Advertising plays an important role in sales. Hence, the first (Population) model and last (Advertising) model are better for predicting Gross Sales.
10.
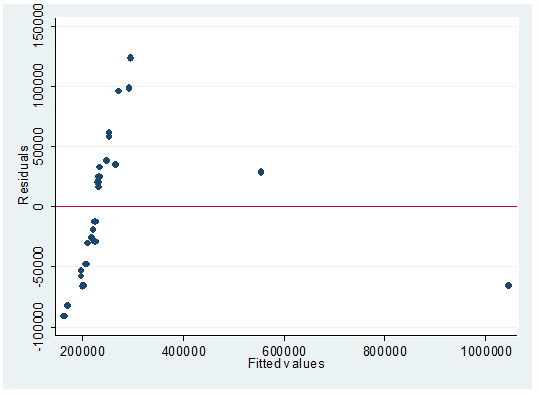
The residual is around zero and this authenticate the linearity between the variables.
11.
Gross Sales = 158404.5 + 1.841834* 144000
Gross Sales = 423628.6
If the actual Gross Sales for the month were $420,624, then the predicted value is reasonable since the deviation is not too large.
12.
a. Slope = 1.841834
The slope implies that the value of Gross Sales is expected to increase by 1.841834 as a result of a unit increase in population.
b. Intercept = 158404.5
This implies that the expected value of Gross sales is 158404.5 when the population is constant.
13. Recommendation
From the result carried out, it is recommended that a multiple regression models which incorporates all the explanatory variables into the model at once be conducted for better results and prediction.