Claim Your Discount Today
Get 10% off on all Statistics homework at statisticshomeworkhelp.com! Whether it’s Probability, Regression Analysis, or Hypothesis Testing, our experts are ready to help you excel. Don’t miss out—grab this offer today! Our dedicated team ensures accurate solutions and timely delivery, boosting your grades and confidence. Hurry, this limited-time discount won’t last forever!
We Accept
- Understanding the Basics
- The Origin of Monte Carlo Simulations
- Fundamental Principles of Monte Carlo Simulations
- Navigating the Monte Carlo Maze
- Step 1: Define the Problem
- Step 2: Choose the Simulation Method
- Step 3: Generate Random Numbers
- Step 4: Run the Simulation
- Common Challenges and Solutions
- Handling Complexity
- Divide and Conquer
- Sensitivity Analysis
- Dealing with Uncertainty
- Confidence Intervals
- Monte Carlo Error Analysis
- Conclusion
As students delve into the intricate world of statistics and probability, one concept that often emerges as a challenging maze is the Monte Carlo simulation. This powerful technique, named after the famous Monaco city known for its casinos, is widely used in various fields, including finance, engineering, and science. However, for students facing assignments involving Monte Carlo simulations and needing to complete their Statistics Homework, navigating this maze can be a daunting task. In this blog, we will break down the complexities and provide a comprehensive guide to help students conquer Monte Carlo simulations in their homework.
Understanding the Basics
Monte Carlo simulations are particularly effective in situations where deterministic approaches fall short, such as in the presence of complex interactions or uncertain parameters. By harnessing the power of randomness, these simulations provide a probabilistic lens through which to view and understand intricate systems. In essence, the fundamental principles of Monte Carlo simulations empower scientists, engineers, and analysts to navigate the uncertainties of the real world with a robust and adaptable tool.
The Origin of Monte Carlo Simulations
Monte Carlo simulations, as we know them today, trace their roots back to the Manhattan Project in the 1940s. The project, which aimed to develop the atomic bomb during World War II, required a method to model the behavior of neutrons in complex systems. Scientists, led by Stanislaw Ulam and John von Neumann, faced the challenge of predicting neutron movement within fissile materials accurately. Traditional analytical methods proved insufficient due to the complexity of the systems under study. In addressing this challenge, Ulam and von Neumann conceived the idea of employing random sampling to approximate the behavior of neutrons. This marked the inception of what we now recognize as Monte Carlo simulations. The name itself is a nod to the Monte Carlo Casino in Monaco, known for chance and randomness, reflecting the stochastic nature of the method.
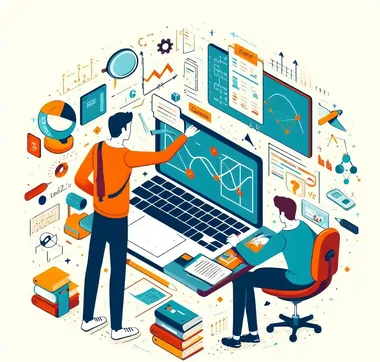
Fundamental Principles of Monte Carlo Simulations
At its core, Monte Carlo simulation is grounded in the principles of probability and statistical inference. The method revolves around the idea of using random sampling to obtain numerical results for a wide range of problems. Whether it's estimating the value of a mathematical integral or simulating the behavior of a dynamic system over time, Monte Carlo methods provide a powerful framework for tackling complex, real-world scenarios. In practical terms, the process begins by defining the problem at hand. This involves identifying the variables and parameters relevant to the system under study. Once the problem is well-defined, Monte Carlo simulations proceed by generating random inputs to the model. These inputs, often guided by probability distributions, represent the inherent uncertainty and variability in the system.
Navigating the Monte Carlo Maze
In the context of the Monte Carlo maze, success is not just about reaching the endpoint; it's about understanding the nuances of the journey. By clearly defining variables and objectives, students illuminate the path ahead, making subsequent steps more deliberate and informed. The ability to navigate this initial phase with precision lays the foundation for a successful and insightful Monte Carlo simulation.
Step 1: Define the Problem
Embarking on the journey of a Monte Carlo simulation is akin to navigating a maze, where each turn represents a crucial decision that shapes the outcome. The first and perhaps the most pivotal turn in this maze is to precisely define the problem at hand. Imagine standing at the entrance, equipped with the tools of probability and statistical analysis, ready to delve into the complexities of predicting stock prices or assessing the reliability of a system. In this labyrinth of uncertainties, a well-defined problem acts as the compass, guiding students through the twists and turns of Monte Carlo simulations.
Clearly Define Variables and Parameters
The path within the Monte Carlo maze starts with identifying the variables and parameters crucial to the simulation. These can range from financial variables, such as stock prices and interest rates, to physical constants or any other factors exerting influence on the system under scrutiny. It's akin to equipping oneself with a map detailing the terrain; without a clear understanding of the variables at play, students risk getting lost in the intricacies of the simulation.
Establish the Objective of the Simulation
As students progress deeper into the maze, they encounter crossroads that demand a decision on the overarching goals of the simulation. Are they navigating this labyrinth to predict future trends, assess the risk inherent in a financial model, or optimize a complex process? Each objective shapes the trajectory of the simulation, determining the methodologies to be employed and the parameters to be prioritized. This step is akin to setting a destination within the maze, guiding the simulation towards a meaningful and purposeful outcome.
Step 2: Choose the Simulation Method
In the intricate world of Monte Carlo simulations, the second step of choosing the right simulation method is a critical juncture that can significantly impact the accuracy and efficiency of results. With an array of methods available, understanding the nuances of sampling techniques and simulation algorithms becomes paramount for success.
Sampling Techniques
Sampling is the cornerstone of Monte Carlo simulations, representing the process of selecting a subset of data points to make inferences about the entire population. Two fundamental sampling techniques, random sampling and stratified sampling, play pivotal roles in shaping the reliability of simulation outcomes.
Simulation Algorithms
Once the sampling technique is chosen, the next crucial aspect is selecting an appropriate simulation algorithm. Various algorithms, each with its unique strengths, are available to cater to different types of problems. Two prominent examples are the Metropolis algorithm and the Markov Chain Monte Carlo (MCMC) method.
Step 3: Generate Random Numbers
In the intricate dance of Monte Carlo simulations, Step 3 takes center stage: generating random numbers. The heart of this simulation method lies in the ability to mimic true randomness through pseudorandom number generators. The quality of these numbers directly influences the accuracy and reliability of the results obtained, making it a pivotal aspect of the entire process.
Pseudorandom Number Generators
Understanding the intricacies of pseudorandom number generators (PRNGs) is essential for any student venturing into the world of Monte Carlo simulations. Unlike true randomness, which is challenging to achieve in a computational setting, PRNGs produce sequences of numbers that closely resemble randomness. These generators rely on mathematical algorithms, often deterministic in nature, to create seemingly unpredictable sequences.
Choosing the right PRNG is akin to selecting the brushstrokes for a masterpiece. The reliability of your simulation outcomes hinges on this choice. Some well-known PRNGs include the Mersenne Twister and the Linear Congruential Generator. Each comes with its own set of strengths and weaknesses, and the suitability of a particular generator depends on the specific requirements of your simulation.
Random Number Seeds
Comprehending the concept of random number seeds adds another layer of sophistication to the process. A seed is the initial input given to a PRNG, acting as a starting point for the generation of the entire sequence. Seeds play a pivotal role in ensuring the reproducibility of simulation results — a critical aspect when validating and refining your models.
Think of the seed as the unique DNA of your simulation. Using the same seed will produce the same sequence of random numbers, allowing you to replicate your results precisely. This reproducibility is invaluable when sharing your work, collaborating with peers, or refining your simulation based on feedback.
Step 4: Run the Simulation
With the groundwork laid, students enter the pivotal phase of their Monte Carlo journey - running the simulation. This step involves translating theoretical concepts into actionable insights by implementing the chosen method, generating random numbers, and observing the system's behavior over multiple iterations. In this dynamic process, two critical aspects demand focused attention: Iteration and Convergence, and Managing Computational Resources.
Iteration and Convergence
Iteration is the heartbeat of Monte Carlo simulations. It refers to the repetition of the simulation process to obtain a set of outcomes. The significance of iteration lies in its ability to capture the randomness inherent in the system, providing a comprehensive view of possible scenarios. Each iteration involves generating a new set of random numbers, simulating the system, and recording the results.
However, the true measure of success in Monte Carlo simulations lies in convergence. Convergence is the point at which the simulation results stabilize, indicating that further iterations are unlikely to significantly alter the outcomes. Monitoring convergence is essential for determining the reliability and accuracy of the simulation results. Achieving convergence ensures that the simulation has adequately explored the parameter space, providing a robust foundation for drawing conclusions and making predictions.
Managing Computational Resources
In the realm of Monte Carlo simulations, efficiency is paramount. Managing computational resources becomes crucial, especially when dealing with complex problems that demand substantial computing power. Two strategies come to the forefront: parallel computing and cloud-based solutions.
Parallel computing involves breaking down the simulation into smaller, independent tasks that can be executed simultaneously. This significantly reduces the time required to complete the simulation, making it feasible to tackle more intricate problems. Leveraging the power of multiple processors or computing nodes, parallel computing optimizes resource utilization and accelerates the simulation process.
Common Challenges and Solutions
Monte Carlo simulations, while powerful, come with their set of challenges. Common issues include handling uncertainty, managing computational resources, and ensuring the reliability of results. Solutions involve techniques like confidence intervals, Monte Carlo error analysis, and optimization strategies. By understanding and applying these solutions, students can navigate the maze of Monte Carlo simulations with confidence and precision.
Handling Complexity
Handling complexity in Monte Carlo simulations is akin to navigating a labyrinth of interconnected variables and intricate systems. As students grapple with assignments involving high-dimensional problems, unraveling the intricacies becomes a paramount challenge. The key to overcoming this challenge lies in the strategic implementation of the "Divide and Conquer" approach.
Divide and Conquer
Dividing the problem into smaller, more manageable parts is a foundational strategy in simplifying Monte Carlo simulations. When faced with a complex system, students can break it down into discrete components, each of which is more comprehensible and analyzable. This not only streamlines the simulation process but also fosters a clearer understanding of the interactions between different components. For instance, in a financial modeling scenario, instead of simulating the entire market, students might break it down into sectors, analyzing each sector's behavior individually. This approach transforms an overwhelming problem into a series of manageable tasks, making the overall simulation more approachable and comprehensible.
Sensitivity Analysis
In addition to dividing and conquering, sensitivity analysis stands as another potent weapon against complexity in Monte Carlo simulations. Given the intricate web of variables, understanding which ones wield the most significant influence on simulation outcomes is crucial. Sensitivity analysis allows students to discern these key variables and prioritize their attention accordingly.
Dealing with Uncertainty
In the realm of Monte Carlo simulations, grappling with uncertainty is an inevitable challenge. Uncertainty arises from the inherent randomness in the simulation process, and mastering how to quantify and manage it is essential for extracting meaningful insights. Two crucial techniques for dealing with uncertainty are Confidence Intervals and Monte Carlo Error Analysis.
Confidence Intervals
In the face of uncertainty, Confidence Intervals (CIs) stand as a powerful tool to provide a clearer picture of the range within which simulation results are likely to fall. Calculating confidence intervals involves statistical methods that take into account the variability in the data. A confidence interval is essentially a range around a point estimate, such as a mean or a proportion, within which we can be reasonably confident the true value lies.
Monte Carlo Error Analysis
Complementing confidence intervals, Monte Carlo Error Analysis provides a deeper understanding of the precision of simulation results. This analysis involves assessing the sources of error within the Monte Carlo simulation, identifying areas where the model may deviate from reality. By scrutinizing the error components, students can refine their models, enhancing accuracy in subsequent simulations.
Conclusion
In conclusion, navigating the maze of Monte Carlo simulations in student homework requires a systematic approach. By understanding the basics, following a structured methodology, and addressing common challenges, students can not only conquer their assignments but also gain valuable insights into the fascinating world of probabilistic modeling. Remember, Monte Carlo simulations are not just a tool; they are a gateway to unraveling the complexities of real-world scenarios, making the journey through the maze both challenging and rewarding.