Principal Component Analysis: A Step-by-Step Approach for Your Stats Homework
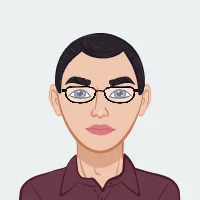
Statistics is undeniably a challenging subject for many students. The complexity of concepts, mathematical calculations, and the interpretation of data can often leave students scratching their heads. Among the myriad of topics in statistics, Principal Component Analysis (PCA) stands out as one of the more advanced and perplexing areas. But fear not! In this blog, we will guide you through a step-by-step approach to demystify PCA and help you excel in your principal component homework, providing the much-needed "Statistics Homework Help" you may be seeking.
Principal Component Analysis, commonly known as PCA, is a statistical technique with a reputation for complexity. It's used for reducing the dimensionality of data while preserving its essential information. In simpler terms, PCA enables you to simplify a dataset, making it more manageable, without losing the critical insights within the data. This technique is often used in various fields, such as data science, machine learning, and biology, to name just a few.

Understanding Principal Component Analysis (PCA)
Principal Component Analysis (PCA) is a fundamental technique in the realm of statistics and data analysis, wielding substantial significance in simplifying complex datasets. At its core, PCA functions as a powerful method for reducing the dimensionality of data, facilitating the extraction of essential information while mitigating the burden of dealing with numerous variables.
Why is PCA important in the realm of statistics and data analysis? One of its pivotal roles is in the reduction of dimensionality within a dataset. This means diminishing the number of variables, which consequently enhances the ease of data handling and computation. By doing so, it eliminates noise and redundancies that might exist in the original, multi-dimensional dataset, ensuring that the most critical elements remain in focus. Moreover, PCA plays a significant role in data visualization. It can take high-dimensional data and project it onto lower dimensions, such as two or three dimensions, allowing for more accessible visualization and interpretation. This aspect is particularly valuable in scenarios where the human mind finds it challenging to comprehend or analyze complex data in its original form.
The process of data reduction through PCA leads to noise reduction, which is crucial for data analysis. By eliminating less relevant information, PCA effectively increases the signal-to-noise ratio in your dataset. This means that the essential information in the data becomes clearer and more distinguishable, allowing for more accurate analysis and interpretation. When it comes to statistics homework, comprehending and applying PCA can be a game-changer. Tackling assignments involving PCA might initially seem daunting, but with a methodical approach and understanding of its fundamental principles, it can become a valuable tool in your statistical toolkit. "Statistics Homework Help" can encompass various topics, and PCA is undoubtedly one of those areas where students might seek guidance and clarity. Understanding PCA thoroughly not only assists in completing assignments but also enriches your knowledge and skills in the broader field of statistics.
Step-by-Step Approach for Principal Component Analysis
Certainly, let's delve deeper into the step-by-step approach for Principal Component Analysis (PCA) to help you gain a thorough understanding of each step and its importance in the data analysis process.
Step 1: Data Preprocessing
Data preprocessing is the initial and critical step in any data analysis, and PCA is no exception. This step comprises two essential components:
Data Cleaning
Before you can embark on PCA, your dataset needs to be cleaned thoroughly. This involves ensuring that the data is free from missing values, outliers, or any inconsistencies that could negatively impact the results of PCA. Data cleaning is essential because it lays the foundation for meaningful and accurate analysis. Here's what you can do in this phase:
- Handling Missing Values: If your dataset contains missing values, you need to decide whether to impute them or exclude the corresponding data points. Imputation methods can include mean imputation, median imputation, or advanced techniques like regression imputation, depending on your data's nature.
- Outlier Detection and Treatment: Outliers are data points that significantly deviate from the rest of the data. They can distort PCA results. Therefore, identifying and handling outliers is essential. Techniques such as the Z-score method or the Interquartile Range (IQR) method can help you detect and handle outliers effectively.
- Data Consistency Check: Ensure that your data is consistent and coherent. It's crucial to verify that the data you're working with makes sense in the context of your analysis.
Standardization
Once your data is cleaned and ready, you must standardize it. Standardization transforms your data so that it has a mean of 0 and a standard deviation of 1. This step is crucial because PCA is sensitive to the scale of your variables. Here's why standardization is essential:
- Equal Scaling: Standardizing your data ensures that all variables are on the same scale. Since PCA is based on the calculation of covariance, the scale of your variables can greatly affect the results. Standardization eliminates this issue.
- Preventing Dominance: In cases where some variables have much larger scales than others, PCA might predominantly focus on those variables, neglecting the importance of others. Standardization ensures that each variable has an equal influence on the principal components.
Step 2: Compute the Covariance Matrix
Once your data is standardized, the next step is to calculate the covariance matrix of your dataset. The covariance matrix provides insight into how different variables are related to each other. This is a pivotal step in PCA, as it forms the basis for identifying the principal components. The covariance matrix is symmetric, with variances on the diagonal and covariances off the diagonal.
By computing the covariance matrix, you're essentially quantifying the relationships between variables. Strong positive covariances indicate that variables increase together, while strong negative covariances suggest that one variable increases as the other decreases.
Step 3: Eigendecomposition
Eigendecomposition is the mathematical procedure used to find the eigenvalues and eigenvectors of the covariance matrix. These are crucial components in PCA, as they define the principal components of your data.
Calculate Eigenvalues
Eigenvalues are a central concept in PCA. They represent the variance explained by each principal component. Larger eigenvalues correspond to principal components that capture more of the total variance in the data. To calculate eigenvalues, you can use various software tools like Python with NumPy, R, or even spreadsheet software like Excel. Once you have these eigenvalues, you'll be able to assess their magnitude to determine the most important principal components.
Calculate Eigenvectors
Eigenvectors accompany eigenvalues. They represent the direction in which the data varies the most, or in simpler terms, they define the new coordinate system in which your data will be transformed. Eigenvectors point out the directions in which your data spreads out or clusters together. These directions are the principal components of your dataset, and they're critical for dimensionality reduction.
Step 4: Select Principal Components
Having obtained the eigenvalues and eigenvectors, your next task is to select the principal components that capture the most variance in your data. This is typically done by ordering the eigenvalues from largest to smallest. By selecting the top 'k' eigenvectors that explain a significant portion of the variance, you can simplify your data.
The idea is to retain as much variance as possible while reducing the dimensionality of the dataset. Selecting a subset of principal components, often significantly fewer than the original variables, allows for a simplified representation of your data, making it more manageable and interpretable.
Step 5: Transform the Data
With the selected principal components in hand, you're ready to transform your original data into a lower-dimensional space. This step simplifies your dataset, making it easier to work with while retaining the most important information. The transformed data preserves the relationships between variables, allowing you to perform analyses and visualizations more effectively.
By following this comprehensive step-by-step approach, you can confidently apply Principal Component Analysis to your data and gain valuable insights. PCA not only simplifies complex data but also helps you extract the most meaningful information from your dataset, which is invaluable in various fields, from finance to biology, and beyond.
Seeking Help When Needed
Statistics can be a daunting subject, and it's entirely normal to encounter difficulties along the way. When you find yourself struggling with a specific topic, such as Principal Component Analysis (PCA) or any other statistics concept, it's essential not to let your uncertainties fester. Seeking help when needed is a crucial step towards mastering the subject.
- Consult Your Professor: Your professors are valuable resources when it comes to understanding challenging statistical concepts. Don't hesitate to reach out to them during office hours or schedule a meeting to clarify your doubts. They can provide explanations, guidance, and additional resources tailored to your specific needs.
- Use Online Resources: The internet is teeming with resources to aid your understanding of statistics. Websites, forums, and online communities dedicated to statistics and data analysis provide a wealth of information, tutorials, and discussions. Platforms like Khan Academy, Coursera, and edX offer online courses that can supplement your learning.
- Consider a Tutor: Sometimes, personalized assistance can make a significant difference in your understanding of statistics. If you find yourself consistently struggling or need intensive help with your assignments, consider hiring a tutor. A tutor can provide one-on-one guidance, addressing your specific questions and concerns, and offering expert insights to overcome difficulties effectively.
Practicing Regularly
Statistics is not a subject that you can master overnight. It requires consistent practice and hands-on experience to build a strong foundation and excel in it. Here's why regular practice is crucial:
- Reinforce Your Knowledge: Regular practice helps you reinforce the concepts you've learned. It ensures that you don't forget what you've studied and helps you retain important information for the long term.
- Build Problem-Solving Skills: Statistics is all about solving problems. Regular practice hones your problem-solving skills, enabling you to approach complex assignments with confidence. As you tackle more problems, you'll become adept at identifying patterns, choosing the right techniques, and drawing meaningful conclusions.
- Stay Current: Statistics is a dynamic field with evolving methodologies and tools. Regular practice keeps you up to date with the latest trends and developments. It ensures that you are well-prepared to handle the challenges posed by modern statistical analyses.
Utilizing Software Tools
The days of laboriously calculating statistics by hand are long gone, thanks to the availability of powerful software tools. Leveraging these tools can streamline your work and enhance your productivity. Here's how you can make the most of software tools in your statistics homework:
- Python and R: These programming languages are widely used in statistics and data analysis. Python libraries like NumPy, pandas, and scikit-learn, as well as R packages, offer a vast array of functions for data manipulation, visualization, and statistical analysis. Learning to use these tools can save you time and help you perform complex calculations with ease.
- Statistical Software Packages: Software like SPSS, SAS, and STATA are specifically designed for statistical analysis. These tools offer user-friendly interfaces and a broad range of statistical tests and data modeling capabilities. While they may require licenses, many educational institutions provide access to these software packages for students.
- Spreadsheet Software: Don't underestimate the power of spreadsheet software like Microsoft Excel or Google Sheets. These tools are excellent for basic data analysis, visualization, and simple statistical calculations. They can be particularly useful for introductory statistics assignments and coursework.
Collaborating with Peers
Working with your peers can be a valuable strategy for excelling in your statistics assignments. Collaboration can take different forms, including study groups, team projects, and peer review. Here are some compelling reasons to collaborate with your classmates:
- Diverse Perspectives: Your peers may have different insights, approaches, and perspectives on statistical problems. Engaging in discussions with them can broaden your understanding and expose you to alternative methods of solving assignments.
- Shared Resources: Collaboration allows you to pool resources and share study materials. Your peers might have notes, textbooks, or online resources that you haven't come across. Leveraging these shared resources can enhance your learning experience.
- Mutual Support: Studying and tackling assignments together creates a supportive environment. When you encounter challenges, your peers can provide encouragement, help clarify doubts, and offer constructive feedback. You'll find that learning becomes a more enjoyable and less daunting experience when you're not tackling it alone.
Conclusion
Principal Component Analysis is a powerful statistical technique that can help you simplify complex data and improve your understanding of high-dimensional datasets. By following the step-by-step approach outlined in this blog, you'll be better equipped to tackle PCA in your statistics homework. Remember to practice, seek help when needed, and use available resources to excel in this challenging yet rewarding subject.
With the right approach and a little determination, you can master statistics and ace your assignments, ensuring that "Statistics Homework Help" is a phrase that you no longer fear.