Mixed Models in SPSS: Understanding Advanced Statistical Techniques
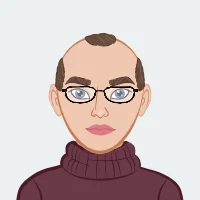
Statistics, a formidable tool in the landscape of research, serves as the compass that guides us through the vast sea of data, enabling us to extract meaningful insights and draw informed conclusions. Amidst the diverse array of statistical techniques, one that has gained prominence in recent years is the application of mixed models. Also recognized as hierarchical linear models or multilevel models, mixed models offer a versatile framework for dissecting data characterized by intricate structures. As we embark on this exploration of mixed models within the context of statistical analysis, the focus turns to SPSS, a widely embraced statistical software package. With its user-friendly interface and robust functionalities, SPSS emerges as a beacon for researchers and students alike, providing a powerful platform to unravel the complexities associated with mixed models. If you need help with your SPSS homework, don't hesitate to reach out for expert support.
These models are well-suited for scenarios where standard linear models fall short, capturing the nuanced relationships within the data. SPSS, as the chosen vehicle for our exploration, facilitates the implementation of mixed models with remarkable ease. Its intuitive interface guides users through the process of specifying both random and fixed effects, essential components of mixed models. Random effects capture the variability at different levels, acknowledging and incorporating the hierarchical nature of the data. On the other hand, fixed effects represent systematic influences on the dependent variable, providing a means to explore the impact of specific conditions or factors of interest. As we navigate the SPSS interface, the step-by-step process of setting up a mixed model unfolds. Dialog boxes and menus become our tools of choice, allowing us to define the covariance structure, specify fixed and random effects, and tailor the model to the intricacies of the dataset at hand. The user-friendly nature of SPSS demystifies the complexities of mixed models, making them accessible to both novice and experienced researchers.
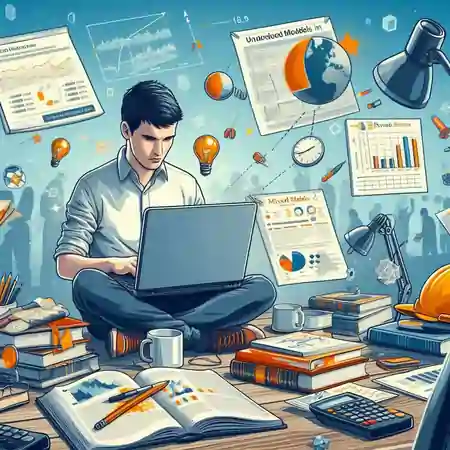
The Basics of Mixed Models
Understanding the fundamentals of mixed models is crucial for researchers and students aiming to harness the power of this versatile statistical technique. At the heart of mixed models lies the recognition that many datasets exhibit a hierarchical structure. Whether we're examining students nested within schools or repeated measurements within individuals, acknowledging and accounting for this hierarchy is essential for accurate statistical modeling. In this section, we will explore the basics of mixed models, with a focus on hierarchical structure, random effects, fixed effects, and the seamless implementation of these concepts using SPSS.
Hierarchical Structure and Random Effects
One of the distinguishing features of mixed models is their ability to handle data with a hierarchical structure effectively. In many real-world scenarios, observations are not independent; instead, they are nested within higher-level units. For example, students are nested within schools, and measurements are nested within individuals. Recognizing this hierarchical arrangement is crucial for capturing the inherent complexity of such data. Random effects play a pivotal role in mixed models by allowing researchers to model and account for variability at different levels of the hierarchy. Unlike fixed effects, which represent systematic influences on the dependent variable, random effects account for unobserved or latent sources of variability.
In our school and student example, random effects would capture the variability between schools, acknowledging that students within the same school may be more similar to each other than to students in other schools. SPSS, a user-friendly statistical software package, simplifies the incorporation of hierarchical structure and random effects into the modeling process. The software's intuitive interface guides users through the specification of random effects, making it accessible even to those new to the complexities of hierarchical modeling. This ensures that researchers and students can easily implement mixed models without getting bogged down by the intricacies of statistical modeling.
Fixed Effects and Covariates
Complementing the role of random effects are fixed effects, which capture systematic influences on the dependent variable. These effects represent conditions that are deliberately manipulated or selected in a study, such as treatment conditions, time points, or any other variable of interest. In our school and student example, fixed effects might represent the impact of a specific teaching method applied uniformly across all schools. SPSS facilitates the seamless inclusion of fixed effects, allowing users to specify and estimate the impact of different conditions on the dependent variable. Moreover, the software provides a user-friendly platform for incorporating covariates into the model.
Covariates are additional variables that may influence the relationship between the independent and dependent variables, providing a more nuanced understanding of the relationships within the data. By incorporating fixed effects and covariates, researchers can account for systematic influences beyond the hierarchical structure, enriching the model's explanatory power. SPSS's user-friendly interface ensures that users can easily include these components in their analyses, empowering them to conduct more sophisticated and comprehensive statistical modeling.
Implementing Mixed Models in SPSS
Mixed models, also known as hierarchical linear models, have become increasingly popular in the field of statistics for their ability to handle complex data structures. Implementing these models in SPSS, a user-friendly statistical software, is an accessible yet powerful process. In this section, we will explore the basics of implementing mixed models in SPSS, providing a step-by-step guide to help users make the most of this versatile tool.
Navigating the SPSS Interface for Mixed Models
One of the key advantages of using SPSS for mixed models is its intuitive interface. Navigating through the software, users can seamlessly specify both random and fixed effects, as well as define the covariance structure. The user-friendly menus and dialog boxes simplify what might otherwise be a daunting process, particularly for those new to advanced statistical techniques. To get started, users can access the mixed models feature within the "Analyse" menu. From there, they can select the "Mixed" option, initiating the setup process. Within the dialog box, researchers can define the dependent variable, fixed factors, and random factors.
SPSS guides users through each step, ensuring that the model is appropriately configured to address the hierarchical nature of the data. This section acts as a virtual tour guide, walking users through the various steps of setting up a mixed model. Whether you are dealing with nested data, repeated measures, or both, SPSS's interface allows for the seamless incorporation of these complexities. By the end of this step-by-step process, users will have a well-specified mixed model ready for analysis.
Interpreting Output and Diagnostics
Once the model is set up and executed, the next critical step is interpreting the output. SPSS provides a comprehensive set of results, including parameter estimates, standard errors, and significance tests. Understanding how to extract meaningful insights from this wealth of information is crucial for researchers and students alike. This section of the guide focuses on developing the skills needed to interpret output and conduct diagnostics. Users will learn to decipher parameter estimates, discern the significance of fixed and random effects, and identify potential issues such as multicollinearity or outliers.
SPSS offers various diagnostic tools, and knowing how to utilize them ensures the validity of the mixed model. By guiding users through the intricacies of output interpretation, this section enhances the user's ability to draw meaningful conclusions from their analyses. It emphasizes the importance of not only running the model but also scrutinizing the results to ensure that the model accurately represents the underlying structure of the data.
Advanced Topics in Mixed Models
As researchers progress in their statistical journey, they often find themselves faced with more intricate data structures and relationships. This is where advanced topics in mixed models become invaluable. These topics, including cross-level interactions and nonlinear effects, elevate statistical analyses to a higher level of sophistication. In this section, we will explore the nuances of these advanced concepts within the realm of mixed models and how SPSS facilitates their implementation.
Cross-Level Interactions and Nonlinear Effects
As you delve deeper into the realm of mixed models, you'll encounter scenarios where the relationships between variables are not constant across all levels of your data hierarchy. This phenomenon, known as cross-level interaction, highlights the dynamic nature of relationships that may change depending on the level of analysis. SPSS, with its robust capabilities, enables researchers to explore and incorporate cross-level interactions seamlessly. Cross-level interactions allow for a more nuanced understanding of how the effect of one variable on the outcome may vary across different levels. For instance, in educational research, the impact of a teaching intervention may differ among various schools.
SPSS empowers researchers to model and interpret these variations, shedding light on the context-specific nature of relationships. In addition to cross-level interactions, SPSS also accommodates the inclusion of nonlinear effects within mixed models. Nonlinear effects acknowledge that the relationship between variables may not follow a straight line. This is particularly relevant when dealing with complex, real-world data where linear relationships might oversimplify the true nature of the associations.
Dealing with Missing Data and Model Comparison
In the pursuit of robust statistical analyses, researchers often grapple with missing data. Mixed models in SPSS offer sophisticated strategies to address this common challenge effectively. Whether data are missing completely at random, missing at random, or missing not at random, SPSS provides options to handle missing data appropriately, preventing biased or misleading results.
Moreover, researchers frequently encounter the need to compare different model specifications to determine the most appropriate and parsimonious representation of their data. This process, known as model comparison, is crucial for ensuring that the chosen model accurately captures the underlying patterns in the data. SPSS facilitates model comparison by offering various statistical criteria, such as the Akaike Information Criterion (AIC) or the Bayesian Information Criterion (BIC), aiding researchers in selecting the model that strikes the optimal balance between goodness of fit and simplicity.
Conclusion:
Mastering mixed models in SPSS is not just a skill; it's a gateway to a realm of possibilities for both seasoned researchers and students venturing into the world of advanced statistical techniques. The journey towards proficiency involves a multi-faceted approach that encompasses understanding the basics, implementing models in SPSS, and delving into advanced topics. This comprehensive skill set not only elevates your statistical analyses but also equips you to navigate the complexities of real-world data.
At its core, understanding the basics of mixed models is pivotal. Recognizing and appreciating the hierarchical structures within your data is the first step. Whether your data involves repeated measurements within individuals, students nested within schools, or any other nested structure, acknowledging and accounting for this hierarchy is crucial. The integration of random effects in mixed models allows for the modeling of variability at different levels, providing a more accurate representation of the intricacies present in many datasets.