Claim Your Discount Today
Get 10% off on all Statistics homework at statisticshomeworkhelp.com! Whether it’s Probability, Regression Analysis, or Hypothesis Testing, our experts are ready to help you excel. Don’t miss out—grab this offer today! Our dedicated team ensures accurate solutions and timely delivery, boosting your grades and confidence. Hurry, this limited-time discount won’t last forever!
We Accept
- Understanding the Basics of LISREL
- Lack of Familiarity with SEM Concepts
- Mastering LISREL Syntax and Commands
- Data Preparation and Model Specification
- Overcoming Challenges in LISREL Assignments
- Seek Guidance and Support
- Break Down Complex Problems
- Utilize Resources Effectively
- Collaborate and Engage in Peer Learning
- Conclusion
Students tackling LISREL homework often encounter a myriad of challenges that can impede their progress and understanding. One common hurdle is the intricate nature of Structural Equation Modeling (SEM) itself, which forms the backbone of LISREL. Many students find it challenging to grasp the foundational concepts of SEM, including latent variables, observed variables, and the interpretation of path diagrams. Without a solid understanding of these concepts, navigating LISREL's interface and syntax becomes significantly more daunting. Additionally, students may struggle with data preparation and model specification, unsure of which variables to include, how to handle missing data, and whether their models align with theoretical frameworks. Overcoming these challenges requires a combination of theoretical knowledge, technical skills, and practical experience.
To mitigate the obstacles posed by LISREL assignments, students can adopt several strategies to enhance their learning experience and proficiency. Seeking guidance and support from professors, instructors, or peers can provide invaluable insights and clarification on complex concepts. Breaking down assignments into smaller, manageable tasks can make them more approachable, allowing students to focus on understanding each component thoroughly. Moreover, utilizing resources such as textbooks, online tutorials, and software documentation can supplement classroom learning and facilitate self-paced exploration. Collaboration and peer learning also play a pivotal role, as engaging with peers in study groups or online forums fosters a sense of community and provides opportunities for knowledge exchange and peer teaching. By employing these strategies and embracing the learning process, students can navigate the challenges of LISREL assignments with confidence and proficiency.
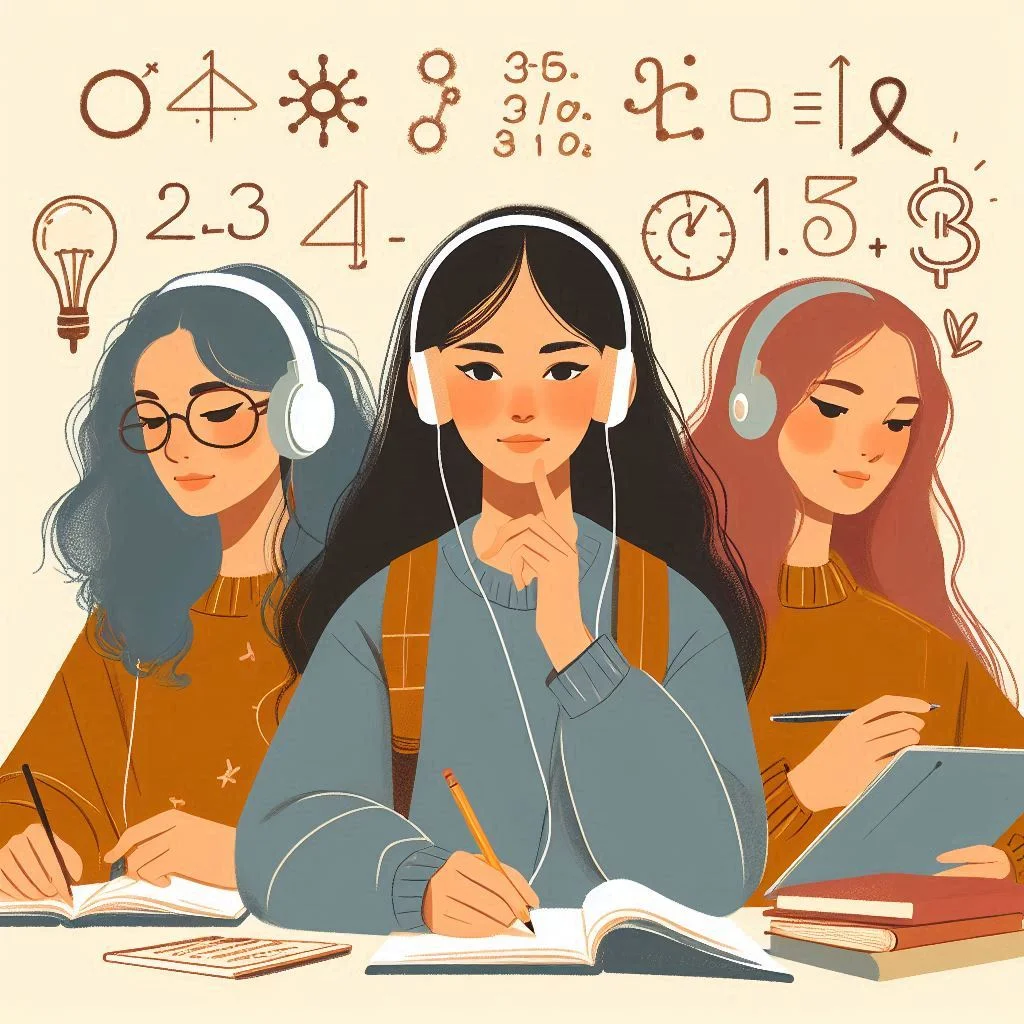
Understanding the Basics of LISREL
Mastering the basics of LISREL begins with a solid understanding of structural equation modeling (SEM) principles. For many students, SEM represents a paradigm shift in statistical analysis, as it allows for the examination of complex relationships among latent and observed variables. Central to SEM is the concept of latent variables, which are unobservable constructs that underlie observed variables. Students must grasp the distinction between latent and observed variables and understand how they relate to one another within the context of a structural model. Moreover, comprehending path diagrams, factor loadings, and model fit indices is essential for interpreting SEM results accurately. By delving into SEM literature and engaging in hands-on practice with LISREL, students can gradually build their proficiency and confidence in navigating the intricacies of SEM analysis.
Furthermore, familiarity with LISREL's interface and syntax is paramount for executing SEM models effectively. LISREL's syntax-driven approach requires students to learn specific commands for model specification, estimation, and modification. This often entails writing scripts to define the relationships between variables, specify measurement models, and assess model fit. Students must invest time in studying LISREL documentation, tutorials, and examples to become proficient in writing syntax and interpreting output. Additionally, exploring alternative SEM software packages can provide students with a broader perspective on SEM analysis and facilitate the transfer of skills across platforms. Through dedicated practice and a commitment to understanding SEM principles, students can overcome the initial challenges associated with mastering LISREL and emerge as adept users of this powerful statistical tool.
Lack of Familiarity with SEM Concepts
Structural Equation Modeling (SEM) encompasses a diverse array of complex statistical concepts that can overwhelm students new to the field. The lack of familiarity with SEM concepts poses a significant challenge, hindering students' ability to effectively utilize LISREL for data analysis. SEM introduces abstract constructs like latent variables, which may not have direct observable measures but are inferred through observed variables. Understanding the relationships between latent and observed variables, as well as comprehending the nuances of path diagrams and factor loadings, requires a solid foundation in statistical theory and methodology. Moreover, grasping the significance of model fit indices and interpreting SEM results in the context of theoretical frameworks further compounds the challenge for students venturing into SEM territory. Overcoming the lack of familiarity with SEM concepts demands dedication to studying foundational literature, engaging in hands-on practice with SEM software, and seeking guidance from experienced mentors or instructors to demystify complex theoretical constructs.
In essence, conquering the barrier of SEM concept familiarity is pivotal for students embarking on LISREL assignments. Embracing a proactive approach to learning, including interactive discussions, practical exercises, and exposure to real-world applications of SEM, can help bridge the gap between theoretical knowledge and practical implementation. By nurturing a deep understanding of SEM principles and techniques, students can not only surmount the initial hurdles of unfamiliarity but also cultivate the analytical skills necessary to tackle sophisticated research questions and contribute meaningfully to the ever-evolving landscape of structural equation modeling and statistical analysis.
Mastering LISREL Syntax and Commands
Mastering LISREL syntax and commands is a pivotal aspect of becoming proficient in structural equation modeling (SEM). Many students encounter initial hurdles in understanding the intricate syntax required to specify, estimate, and modify models in LISREL. The syntax-driven nature of LISREL demands a meticulous approach to coding, where even a small error can significantly impact the analysis results. Thus, students must invest time and effort into familiarizing themselves with LISREL's command structure, understanding the purpose of each command, and learning how to troubleshoot common syntax errors. Furthermore, exploring the documentation provided by LISREL, along with seeking supplementary resources like tutorials and online forums, can aid students in deciphering the complexities of LISREL syntax.
Beyond understanding the syntax itself, mastering LISREL commands involves a deeper comprehension of the theoretical foundations of SEM. Each command in LISREL serves a specific function within the SEM framework, such as specifying latent variables, defining relationships between variables, and evaluating model fit indices. Students must not only memorize the syntax but also grasp the underlying statistical concepts and assumptions behind each command. Engaging in hands-on practice with sample datasets and conducting exploratory analyses can reinforce understanding and proficiency in using LISREL commands effectively. Moreover, seeking guidance from instructors or experienced practitioners can provide invaluable insights into best practices and strategies for optimizing LISREL syntax for various SEM models and analyses.
Data Preparation and Model Specification
Data preparation is a crucial step in SEM analysis, as the quality of input data directly influences the validity and reliability of model estimates. Students often face challenges in preparing data for LISREL assignments, including handling missing data, assessing data distribution, and ensuring the compatibility of variables with the proposed theoretical model. Prior to model specification, it is essential to conduct exploratory data analysis (EDA) to gain insights into the distribution, variability, and relationships among variables. Techniques such as histograms, scatterplots, and correlation matrices can aid in identifying outliers, detecting patterns, and assessing multicollinearity among variables. Additionally, students should employ strategies for handling missing data, such as deletion techniques, imputation methods, or maximum likelihood estimation, based on the nature and extent of missingness in the dataset.
Model specification involves translating theoretical concepts and hypotheses into a formal structural equation model that can be analyzed using LISREL. This process requires careful consideration of the theoretical framework, identification of latent and observed variables, specification of directional relationships among variables, and inclusion of measurement error terms where applicable. Students must articulate hypotheses, justify model assumptions, and validate the model against empirical data. Model specification often involves iterative refinement, where students modify the initial model based on fit indices, modification indices, and theoretical insights. By paying attention to data quality, theoretical coherence, and model specification techniques, students can enhance the robustness and validity of their SEM models in LISREL assignments.
Overcoming Challenges in LISREL Assignments
Overcoming challenges in LISREL assignments requires a multifaceted approach that combines theoretical understanding, technical proficiency, and effective problem-solving strategies. Firstly, students should prioritize a deep comprehension of the underlying principles of Structural Equation Modeling (SEM) and the specific methodologies employed within LISREL. This entails investing time in studying SEM theory, understanding latent and observed variables, comprehending path diagrams, and interpreting model fit indices. With a solid theoretical foundation, students can effectively navigate the complexities of LISREL assignments and make informed decisions at each stage of the analysis process, from data preparation to model specification and interpretation of results.
Secondly, mastering the technical aspects of LISREL involves practical experience and hands-on engagement with the software. Students should dedicate time to familiarizing themselves with LISREL syntax, commands, and procedures for model estimation and modification. Breaking down complex problems into manageable tasks, students can incrementally build their proficiency in LISREL through practice exercises, tutorials, and real-world applications. Moreover, seeking guidance from instructors, collaborating with peers, and leveraging online resources can provide valuable support and facilitate learning. By embracing a proactive and systematic approach, students can overcome challenges in LISREL assignments and develop the skills necessary for advanced statistical analysis and research in the field of social sciences and beyond.
Seek Guidance and Support
Seeking guidance and support is paramount for students facing challenges in LISREL assignments. Professors and instructors are valuable resources who can provide clarity on complex concepts, offer personalized assistance, and address specific questions related to SEM and LISREL. Office hours and one-on-one consultations create opportunities for in-depth discussions and tailored guidance, enabling students to overcome obstacles effectively. Furthermore, reaching out to peers who have experience with LISREL can foster a sense of camaraderie and mutual support. Online forums, discussion groups, and study sessions facilitate collaboration, allowing students to share insights, troubleshoot problems together, and benefit from diverse perspectives. Engaging with a supportive community not only enhances learning but also instills confidence and resilience in tackling LISREL assignments.
Moreover, seeking guidance extends beyond academic settings to encompass online resources and software documentation. Tutorials, video lectures, and written guides offered by educational websites and software providers offer step-by-step instructions and practical tips for using LISREL effectively. Online forums and Q&A platforms provide platforms for students to seek clarification on specific issues, learn from others' experiences, and stay updated on best practices in SEM analysis. By leveraging a combination of academic support, peer collaboration, and online resources, students can navigate the intricacies of LISREL assignments with greater confidence and competence.
Break Down Complex Problems
Breaking down complex problems is a fundamental strategy for tackling LISREL assignments effectively. When faced with intricate modeling tasks or data analyses, students can benefit immensely from breaking the problem into smaller, more manageable components. This approach allows for a systematic exploration of each aspect of the assignment, from data preparation to model specification and interpretation of results. By breaking down the problem, students can focus their attention on understanding the underlying concepts and relationships within the model, ensuring clarity and coherence in their analysis.
Furthermore, breaking down complex problems fosters a sense of progress and accomplishment as students make incremental advancements towards solving the assignment. Each component tackled provides an opportunity for deeper learning and mastery of specific techniques and methodologies. By dividing the problem into smaller tasks, students can identify areas of difficulty more readily and seek targeted assistance or resources to overcome challenges. Ultimately, breaking down complex problems empowers students to approach LISREL assignments with confidence and precision, leading to more robust analyses and insights in structural equation modeling.
Utilize Resources Effectively
Utilizing resources effectively is paramount for students striving to excel in LISREL assignments. Textbooks, online tutorials, and software documentation serve as foundational pillars in understanding the intricacies of LISREL and structural equation modeling (SEM). By delving into comprehensive textbooks authored by experts in the field, students gain insights into theoretical frameworks, model specification techniques, and interpretation of results. Additionally, online tutorials and video lectures offer dynamic learning experiences, providing step-by-step guidance on syntax writing, data preparation, and model estimation within the LISREL environment. Moreover, software documentation acts as a valuable reference guide, offering detailed explanations of LISREL commands, functions, and procedures, thereby empowering students to navigate the software with confidence and precision.
Furthermore, actively engaging with sample datasets and conducting exploratory analyses reinforces theoretical concepts and enhances practical skills. Students can leverage sample datasets provided by textbooks, academic journals, and online repositories to practice model specification, parameter estimation, and model evaluation. Through hands-on experimentation, students develop proficiency in data manipulation techniques, error detection, and troubleshooting, thereby bolstering their problem-solving abilities. Additionally, exploring alternative software packages and tools that complement LISREL expands students' analytical toolkit and fosters adaptability in addressing diverse research questions and analytical challenges. By embracing a multifaceted approach to resource utilization, students cultivate a robust foundation in LISREL and SEM, equipping themselves for success in academic endeavors and beyond.
Collaborate and Engage in Peer Learning
Collaborating and engaging in peer learning can significantly enhance students' understanding of LISREL and SEM concepts. By forming study groups or joining online communities, students create environments conducive to knowledge exchange and collective problem-solving. Within these groups, individuals with varying levels of expertise can offer unique perspectives, fostering a rich learning experience. Through active participation in discussions, students can clarify doubts, share insights, and brainstorm solutions to challenging problems. Moreover, explaining complex concepts to peers not only reinforces understanding but also cultivates communication and teaching skills, essential for academic and professional growth in the field of statistics and structural equation modeling.
Peer learning also promotes a sense of accountability and motivation among students. Knowing that they are part of a collaborative effort encourages individuals to stay committed to their learning goals and contribute actively to group activities. Additionally, peer feedback provides valuable insights into one's strengths and areas for improvement, facilitating continuous learning and self-reflection. By harnessing the collective knowledge and support of peers, students can overcome obstacles more effectively and build a strong foundation for mastering LISREL and excelling in SEM analyses.
Conclusion
In conclusion, students venturing into LISREL assignments should approach the challenges with a proactive mindset, recognizing that the learning curve is an integral part of mastering this sophisticated statistical tool. The intricacies of SEM and the specificities of LISREL syntax demand a strategic blend of theoretical understanding and hands-on practice. By acknowledging the challenges associated with SEM concepts, syntax, and data preparation, students can adopt a structured learning approach, breaking down complex tasks into manageable steps. Seeking guidance, collaborating with peers, and utilizing available resources are indispensable strategies that empower students to overcome obstacles and emerge as confident users of LISREL.
As the field of structural equation modeling continues to evolve, the ability to navigate LISREL proficiently opens doors to a deeper understanding of complex relationships within data. Embracing the challenges of LISREL assignments not only contributes to academic success but also equips students with valuable skills applicable in diverse research and analytical endeavors. Ultimately, the journey of conquering LISREL challenges becomes a transformative experience, shaping students into adept statisticians and researchers prepared to unravel the complexities of real-world data with precision and confidence.
In summary, the journey to proficiency in LISREL may be challenging, but with perseverance and the right strategies, students can navigate through complexities and emerge as proficient users of this powerful statistical tool.