Claim Your Discount Today
Get 10% off on all Statistics homework at statisticshomeworkhelp.com! Whether it’s Probability, Regression Analysis, or Hypothesis Testing, our experts are ready to help you excel. Don’t miss out—grab this offer today! Our dedicated team ensures accurate solutions and timely delivery, boosting your grades and confidence. Hurry, this limited-time discount won’t last forever!
We Accept
- Key Concepts in Survival Analysis
- Survival Function (S (t))
- Hazard Function (λ(t))
- Censoring
- Survival Data
- Kaplan-Meier Estimator
- Hazard Functions
- Cox Proportional Hazards Model
- Handling Censored Data
- Strategies for Solving Survival Analysis Homework
- Leverage Simulation
- Sensitivity Analysis
- Real-World Case Studies
- Machine Learning Integration
- Time-Dependent Covariates
- Bayesian Survival Analysis
- Non-Proportional Hazards
- Software Proficiency
- Conclusion
Survival analysis is a powerful statistical technique used in various fields, such as medical research, finance, and engineering, to complete your survival analysis homework and analyze the time until an event of interest occurs. Whether you're a student delving into this field for the first time or an aspiring data scientist honing your skills, understanding the key topics and knowing how to approach survival analysis homework is crucial. In this blog, we'll break down the essential topics you should be familiar with before tackling survival analysis homework and provide a step-by-step guide to solving survival analysis problems effectively. Survival analysis, also known as time-to-event analysis, focuses on studying the time it takes for an event of interest to occur. This could range from the time until a patient's recovery in a medical study to the time until a component fails in an engineering context. It accounts for censored data, which occurs when the event has not occurred for certain subjects by the time of analysis
Key Concepts in Survival Analysis
Before you immerse yourself in the intricate world of solving survival analysis problems, it's crucial to build a solid foundation in the key concepts that underpin this field. These fundamental ideas are the building blocks upon which you'll construct your understanding of survival functions, hazard functions, and the nuances of censoring.
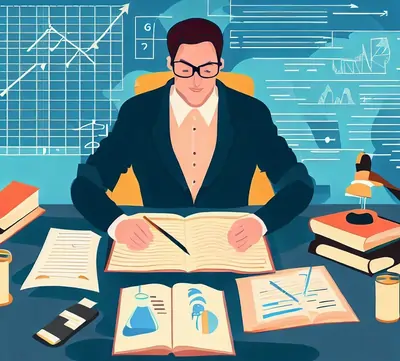
Survival Function (S (t))
At the heart of survival analysis lies the concept of the survival function, denoted as S (t). Imagine you're investigating the survival time of patients after medical treatment. The survival function provides insights into the probability that a subject will survive beyond a certain time’t’. In simpler terms, S (t) tells us the likelihood that an event (such as death, recovery, or failure) hasn't occurred by time 't'.
As an example, if S(10) = 0.8, it means that there's an 80% chance a patient will survive beyond 10 units of time (whether those units are days, months, or any other unit of measurement relevant to your analysis).
Hazard Function (λ(t))
Complementary to the survival function is the hazard function, often denoted as λ(t). While the survival function indicates the probability of an event not happening up to time 't', the hazard function provides a different perspective. It conveys the instantaneous probability that the event will happen at precisely time 't', given that it hasn't occurred until that point.
For instance, if the hazard function at t=5 is high, it suggests that the risk of the event happening at exactly time 5 is elevated. Conversely, if the hazard function is low at t=15, the event is less likely to happen around that time point.
Censoring
One of the distinctive challenges of survival analysis is dealing with incomplete data, often referred to as censoring. Censoring occurs when you don't have complete information about the event of interest for certain subjects in your study. This could be due to various reasons, such as participants dropping out of a study or the event not occurring within the study's duration.
There are two main types of censoring:
- Right-Censoring
- Left-Censoring
Right-censoring occurs when the event of interest has not happened for some subjects by the time of analysis. Imagine a study tracking the time until the failure of a machine part. If the study ends before some parts fail, you have right-censored data for those parts. This means you know they haven't failed yet, but you don't know when they will.
Conversely, left-censoring arises when the event occurred before the study started, but the exact time of the event is unknown. This could happen when you're examining the time at which patients contracted a disease, but you only began observing them after they were already diagnosed.
Understanding the nuances of censoring is vital as it impacts how you analyze and interpret your data. It's crucial to employ appropriate statistical methods to account for censoring and ensure accurate results.
Survival Data
Survival data comes in various flavours, each demanding a unique approach to analysis:
- Uncensored Data: The most straightforward type, where you have complete information on event times for all subjects.
- Right-Censored Data: This is the most common scenario. You know the event status for some subjects, but others are right-censored. The challenge is to estimate the survival function and hazard function while accounting for those censored observations.
- Left-Censored Data: While less common, left-censored data occurs when you only observe subjects after the event has already happened.
- Interval-Censored Data: Here, you know the event occurred within certain intervals, but the exact time is unknown. This arises in scenarios where events are only recorded at specific time intervals.
A firm grasp of these key concepts will pave the way for a deeper understanding of survival analysis methods and techniques. Armed with this knowledge, you're ready to embark on the journey of solving survival analysis problems with confidence and precision.
Kaplan-Meier Estimator
The Kaplan-Meier estimator is a non-parametric method to estimate the survival function. It's particularly useful for analyzing survival in the presence of censored data. Key steps include:
- Sort the data in ascending order of event times.
- Calculate the proportion of surviving subjects at each time point.
- Multiply the survival probabilities to obtain the overall survival curve.
Hazard Functions
The hazard function provides insights into the instantaneous risk of an event occurring. It's a fundamental component of survival analysis and can be estimated non-parametrically or using parametric models.
Cox Proportional Hazards Model
The Cox PH model is a widely used semi-parametric approach that examines how different variables affect the hazard function. The model assumes that the hazard ratio (relative risk) is constant over time. It's crucial to understand the basics of Cox PH and how to interpret the results.
Handling Censored Data
Censored data is common in survival analysis, and dealing with it requires attention:
- Right-Censoring: Utilize techniques like the Kaplan-Meier estimator and Cox PH model.
- Left-Censoring: Special methods are needed to handle this type of censoring.
Strategies for Solving Survival Analysis Homework
Navigating the intricacies of survival analysis homework requires a systematic and informed approach. In this section, we'll explore key strategies that will empower you to tackle survival analysis problems with confidence and precision. By following these strategies, you'll not only unravel the complexities of survival data but also develop a solid foundation for future applications of this critical statistical technique.
Leverage Simulation
Creating simulated datasets that mimic different survival scenarios can be a valuable strategy. By generating data with known properties, survival times, and censoring patterns, you can test your understanding of various techniques and models. This approach enhances your ability to grasp the impact of different factors on the analysis outcomes and provides a controlled environment for learning.
Sensitivity Analysis
Explore the sensitivity of your results to changes in assumptions or parameters. This involves analyzing variations in key variables or methods to observe how robust your conclusions are. Sensitivity analysis not only helps you understand the stability of your results but also demonstrates a higher level of analytical rigour and critical thinking.
Real-World Case Studies
Research real-world case studies where survival analysis has been applied successfully. Analyze published papers, reports, or articles that use survival analysis to answer important questions in various fields. Understanding how experts approach complex problems and interpret results can provide valuable insights into your analysis.
Machine Learning Integration
Consider incorporating machine learning techniques alongside traditional survival analysis methods. Machine learning algorithms, such as random forests or support vector machines, can complement your analysis by capturing complex interactions and patterns within the data. This hybrid approach can provide deeper insights and potentially improve prediction accuracy.
Time-Dependent Covariates
Explore the impact of time-dependent covariates in your analysis. These are variables that change over time and can influence the hazard rate. Incorporating these covariates requires advanced modelling techniques but can provide a more nuanced understanding of the underlying processes contributing to the event of interest.
Bayesian Survival Analysis
Dive into Bayesian survival analysis, which leverages Bayesian statistics to estimate survival distributions and make predictions. This approach allows you to incorporate prior knowledge and beliefs into your analysis, enabling more flexible and informative results.
Non-Proportional Hazards
Delve into scenarios where the proportional hazards assumption of the Cox PH model may not hold. Explore techniques to handle non-proportional hazards, such as time-varying effects or stratified analyses. This advanced strategy showcases your ability to tackle more intricate challenges in survival analysis.
Software Proficiency
Become proficient in using specialized software packages designed for survival analysis, such as R's survival package or Python's lifelines library. These tools offer a wide range of functions, allowing you to implement various methods efficiently and effectively.
Incorporating these unique strategies into your survival analysis toolkit can elevate your problem-solving skills and enhance your ability to tackle diverse and complex homework. Remember that mastering survival analysis is an ongoing journey, and by continuously exploring new strategies, you'll continue to refine your expertise in this intricate statistical field.
Conclusion
Survival analysis is a fascinating field with a broad range of applications. Mastering the core concepts and techniques is essential for effectively solving survival analysis homework. By comprehending the nuances of survival functions, hazard functions, and various models, and by employing systematic problem-solving strategies, you'll be well-equipped to tackle any survival analysis challenge that comes your way. Remember, consistent practice and a curious mindset will contribute to your success in this complex yet rewarding realm of statistics.