Claim Your Discount Today
Get 10% off on all Statistics homework at statisticshomeworkhelp.com! Whether it’s Probability, Regression Analysis, or Hypothesis Testing, our experts are ready to help you excel. Don’t miss out—grab this offer today! Our dedicated team ensures accurate solutions and timely delivery, boosting your grades and confidence. Hurry, this limited-time discount won’t last forever!
We Accept
Review Our Past Time Series Analysis Homework for Inspiration
Peek at our completed Time Series Analysis homework to see the quality and precision we deliver. Each sample reflects our commitment to excellence and can inspire your own projects. Explore these examples to understand how we can assist with your homework and elevate your academic performance.
Time Series Analysis
Time Series Analysis
No Hidden Fees – See Our Upfront Pricing for Time Series Analysis Homework Help
We believe in transparent pricing, so you’ll always know what you’re paying for when you request our Time Series Analysis homework help. Our rates are fair and based on the complexity of each homework. To get an accurate estimate, we offer a free quote tailored to your specific homework needs.
Homework Type | Basic | Intermediate | Advanced |
---|---|---|---|
Simple Forecasting | $50 | $75 | $100 |
ARIMA Modeling | $60 | $85 | $110 |
Seasonal Decomposition Analysis | $65 | $90 | $120 |
Autocorrelation Analysis | $55 | $80 | $105 |
Advanced Time Series Regression | $70 | $100 | $130 |
- Save Time and Boost Grades with Foolproof Time Series Analysis Homework Help Online
- Expert Help with Time Series Analysis Homework for Every Topic You Face
- We Boast Verified Time Series Analysis Homework Doers Who Overcome All Your Challenges
- We Are Available to Do Your Time Series Analysis Homework 24/7
- Acquiring Our Time Series Analysis Homework Help Is Easier Than You Think
Save Time and Boost Grades with Foolproof Time Series Analysis Homework Help Online
Maximize your efficiency and elevate your grades with our expert Time Series Analysis homework help. Our tailored assistance ensures that complex tasks are handled swiftly, giving you more time to focus on other priorities. With our support, you can confidently tackle your homework and see improvements in your academic performance.
Our Time Series Analysis homework assistance provides clear, accurate solutions that align with your course requirements. We simplify intricate concepts and deliver well-structured homework, helping you grasp key principles and excel in your studies. Trust our team to offer practical guidance and boost your understanding of time series analysis.
Don’t let deadlines stress you out. Our efficient approach to handling time series analysis homework allows you to save valuable time while achieving top grades. By choosing our professional statistics homework help services, you get dependable and expert support designed to enhance your academic success and ease your workload.
Expert Help with Time Series Analysis Homework for Every Topic You Face
When you encounter challenges with your time series analysis homework, our service offers comprehensive help with Time Series Analysis homework. From fundamental concepts to advanced techniques, we provide solutions that cover a wide range of topics. Our expertise ensures that no matter what you’re working on, you’ll receive accurate and detailed assistance.
For students seeking professional assistance with Time Series Analysis homework, our team is equipped to handle every topic you face. We break down complex problems and deliver well-crafted solutions tailored to your needs. With our support, you’ll gain a better grasp of time series methods and improve your overall performance in homework.
Here are some of the topics we’ve tackled before:
- Seasonal Decomposition of Time Series
- ARIMA and SARIMA Models
- Forecasting with Exponential Smoothing
- Stationarity and Differencing Techniques
- Autocorrelation and Partial Autocorrelation Functions
- Time Series Regression Analysis
- Long-Term Trends and Cyclic Patterns
Our expertise spans various aspects of time series analysis, ensuring you receive the precise support needed to excel.
We Boast Verified Time Series Analysis Homework Doers Who Overcome All Your Challenges
Our team consists of verified Time Series Analysis homework helpers who are skilled at tackling every challenge students face. With their expertise, they ensure that your homework are completed efficiently and with precision. Whether it’s handling complicated datasets or addressing intricate models, our professionals are well-prepared to provide thorough solutions to your homework problems.
Our experienced Time Series Analysis homework writers have been carefully vetted to guarantee top-notch assistance for any task. They understand the specific challenges students face and work diligently to overcome them. With their knowledge, you can rest assured that even the most complex aspects of time series analysis will be handled with care.
Here are six common challenges our experts help students overcome:
- Misinterpreting autocorrelation and partial autocorrelation functions
- Struggling with ARIMA model identification
- Understanding stationarity and transformation techniques
- Dealing with seasonal adjustment issues
- Implementing accurate forecasting models
- Handling large datasets with missing values
By relying on our verified homework doers, you can confidently navigate these obstacles and achieve the best results.
We Are Available to Do Your Time Series Analysis Homework 24/7
If you need someone to do my Time Series Analysis homework, our team is here around the clock to assist. We understand that tight deadlines and sudden homework can arise at any moment, which is why we offer 24/7 availability. Our team works tirelessly to ensure you get the help you need, whenever you need it.
Whether you’re looking for someone to write your Time Series Analysis homework or simply provide expert assistance, we’ve got you covered. Our round-the-clock service ensures that no matter when a problem arises, we can help you get it solved promptly. We're always here to lighten your load.
Here’s how we make sure our service is always running:
- Experts available across different time zones
- Dedicated support team for real-time responses
- Fast homework allocation system
- Instant updates on homework progress
- 24-hour monitoring to handle urgent requests
- Reliable infrastructure to prevent service downtime
With us, you can confidently rely on timely and consistent support, no matter the time of day.
Acquiring Our Time Series Analysis Homework Help Is Easier Than You Think
Getting professional help with your time series analysis homework has never been simpler. At Statistics Homework Helper, we have streamlined the process to make it quick and hassle-free. Whether you need support with complex models or basic analysis, our service ensures you receive accurate, timely solutions every time.
Here’s how you can get started with our Time Series Analysis homework help:
- Send us the details of your homework through our order form or contact our customer support team.
- Receive a cost-effective price quote based on the complexity and scope of your homework.
- Make the initial payment, and our experts will begin working on your homework right away.
- Get notified when your homework is completed and ready for review.
- Pay the remaining balance and download your completed solutions.
- Share your feedback and review our service to help us improve.
By following this simple process, you can easily access the expert help you need from Statistics Homework Helper. We ensure a smooth experience from start to finish, so you can focus on achieving better grades without stress.
Your Guide to Time Series Analysis Success – Blog Articles and More
Stay ahead in your studies with our insightful Time Series Analysis blogs. Our articles cover everything from key techniques to advanced strategies, designed to help students excel in their homework. Whether you need updates on new methods or guidance on tricky homework, our blogs offer practical advice and expert tips for success in time series analysis.
Our Time Series Analysis Homework Assistance: Reviewed by Real Students
Want to know what students think of our service? Check out these genuine reviews from students who’ve used our Time Series Analysis homework assistance. Their feedback highlights the quality, accuracy, and timely support they received, offering an honest look into how we’ve helped them succeed with their homework.
Meet The Minds Behind Time Series Analysis Homework Help
Our team consists of highly qualified and experienced professionals from around the globe, each bringing unique expertise to Time Series Analysis homework. These experts are committed to delivering precise and reliable solutions to students, ensuring academic success. Here are a few of the brilliant minds who help make it all possible:
.webp)
Mr. Oliver Yan
Master’s in Data Science
🇭🇰 Hong Kong
Mr. Oliver Yan completed his Master’s in Data Science from Hong Kong Baptist University. With over 320 homework and 6 years of professional experience, Mr. Yan excels in implementing sophisticated time series models and forecasting techniques. He is particularly known for his ability to simplify complex concepts, making his solutions both accurate and easy to understand for students.
.webp)
Dr. Gwenyth Evans
Ph.D. in Statistics
🇬🇧 United Kingdom
Dr. Gwenyth Evans holds a Ph.D. in Statistics from Aberystwyth University. She has completed over 370 homework and has 8 years of research and teaching experience. Dr. Evans is a seasoned expert in handling multivariate and nonlinear time series, ensuring that students receive comprehensive and in-depth solutions to their homework challenges. Her work is highly praised for its accuracy and clarity.
.webp)
Professor Henry Pembroke
degree in Applied Statistics
🇬🇧 United Kingdom
Professor Henry Pembroke holds a degree in Applied Statistics from the University of Bath. With more than 400 homework completed and 10 years of teaching and research experience, Professor Pembroke is adept at tackling a wide range of time series problems. His thorough understanding of time-series econometrics and model development makes him a reliable expert for even the most challenging homework tasks.
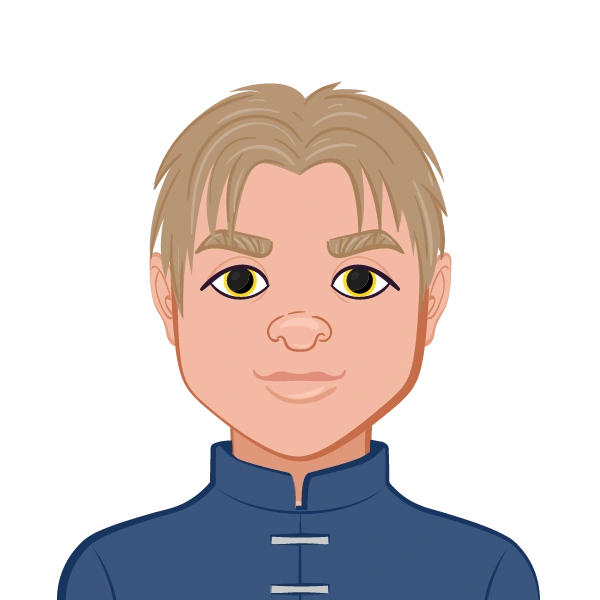
Dr. Vivian Caldwell
Ph.D. in Statistics
🇺🇸 United States
Dr. Vivian Caldwell earned her Ph.D. in Statistics from Claremont Graduate University. With over 380 completed homework and 7 years of experience, she has developed a deep understanding of statistical modeling and time series analysis. Dr. Caldwell is known for her attention to detail and ability to break down complex data into clear, actionable insights. She is highly regarded for delivering accurate and well-structured solutions.
.webp)
Dr. Fiona Edwards
PhD in Applied Mathematics
🇦🇺 Australia
Dr. Fiona Edwards received her PhD in Applied Mathematics from Simon Fraser University. She has completed 620+ homework and boasts 9 years of experience. Her analytical skills and thorough understanding of forecasting methods provide top-notch support for students' academic needs.
.webp)
Cordell Mattie
Masters in Statistics
🇨🇦 Canada
Cordell Mattie holds a master’s degree in applied Statistics from Emporia State University. He has completed over 600 homework, with 8 years of practical experience in forecasting techniques. His expertise helps students tackle various complex problems with confidence and accuracy.
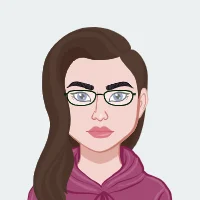
Dr. Emily Harper
Ph.D. in Statistics
🇬🇧 United Kingdom
Dr. Emily Harper earned her PhD in Statistical Science from the University of Exeter and has successfully completed over 900 homework. With 10 years of experience in providing detailed forecasting techniques assistance, she brings a wealth of knowledge and precision to her work, ensuring students receive expert support.
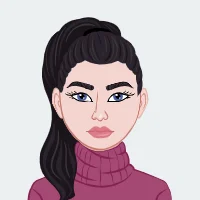
Professor Nigel Blackwood
Ph.D. in Statistics
🇨🇦 Canada
Holding a PhD in Statistics from the University of Illinois at Urbana-Champaign, Professor Nigel Blackwood has completed 750+ homework. With over 12 years of experience in academic support, he excels in delivering high-quality forecasting solutions. His extensive background and commitment to education make him a valued asset to students needing expert help.
.webp)
Mr. Dylan Edwards
Master’s in Financial Mathematics
🇬🇧 United Kingdom
Mr. Dylan Edwards holds a Master’s in Financial Mathematics from Cardiff University and has completed over 300 homework. His practical approach to financial time series analysis and extensive experience with various statistical methods ensure reliable, high-quality results. Mr. Edwards’s expertise is ideal for students needing detailed and accurate analysis of financial data.
.webp)
Dr. Mei Chen
Ph.D. in Applied Mathematics
🇨🇳 China
Dr. Mei Chen received her Ph.D. in Applied Mathematics from Fudan University and has successfully completed more than 340 homework. Her expertise in financial time series analysis is underscored by her ability to apply advanced statistical techniques to real-world data. Dr. Zhang’s methodological rigor and practical insights make her an invaluable resource for complex homework.
.webp)
Professor Aisling Collins
Master’s in Statistics
🇬🇧 United Kingdom
Professor Aisling O’Connor earned her Master’s in Statistics from the University of Leicester and has completed over 320 homework. She combines rigorous academic knowledge with practical experience, making her an expert in analyzing financial time series data. Her approach emphasizes clarity and precision, ensuring comprehensive support for students tackling challenging financial analysis projects.
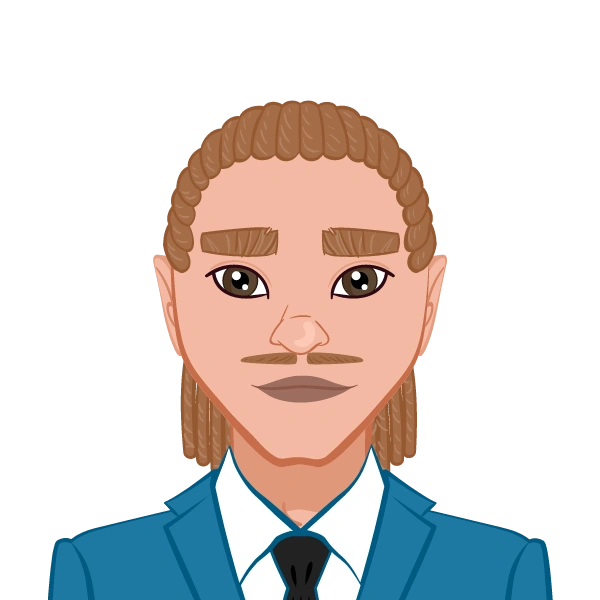
Dr. Jasper Collins
Ph.D. in Financial Economics
🇺🇸 United States
Dr. Jasper Collins holds a Ph.D. in Financial Economics from the University of California, Riverside. With over 350 homework completed, he brings a wealth of experience to financial time series analysis. Dr. Collins has a strong track record of delivering insightful, data-driven solutions tailored to complex financial models. His extensive expertise ensures high-quality assistance for intricate homework.
Frequently Asked Questions
Got questions about our Time series analysis homework help? Our FAQ section covers everything you need to know, from who can use our services to how we handle urgent requests. Discover the details about our affordable pricing, qualified writers, and 24/7 availability. Get all the answers you need to make informed decisions.