- Heteroscedasticity
- Serial correlation of error terms
- Nonlinearities
- Structural changes in regression coefficients
- Omitted variables
- Functional misspecification
Diagnostic checking in stepwise regression
As stated, stepwise regression is an essential tool in data manipulation. However, there are many drawbacks that could affect the quality of results obtained from stepwise regression models, which could lead to misinterpretation of these results. Some of the drawbacks that are considered especially unfavorable include:
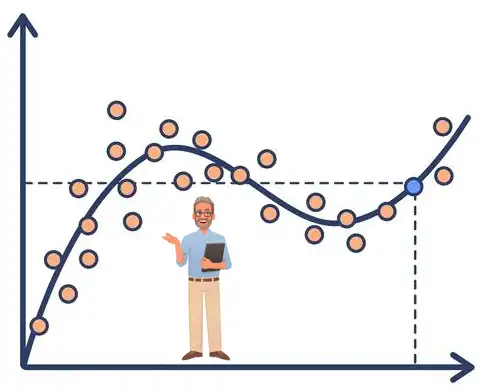
How stepwise regression works
There are two ways of performing stepwise regression:
- Starting with all the available predictor variables: In this method, you delete one variable after the other as the regression model develops or progresses. If you have a small number of variables and you wish to get rid of a few, this is the method to use. The variable with the least “F –to –remove” value is eliminated from the model at each step. Below is how you can effectively calculate the “F –to –remove” value:
- Calculate a t –statistic for the predicted coefficient of each and every variable in the regression model.
- Square the t –statistic value, and this will create the “F –to –remove” value.
- Starting the test without predictor variables: Also known as theforward method, this technique requires you to add one variable at a time as the regression model develops. It is the perfect method to use when you have a huge set of predictor variables. Here, you will create an “F –to –add” statistics using the same steps above. However, the system will compute the statistic for the variables that are not in the model. In this technique, the variable that has the highest “F –to –add” value will be added to the model.
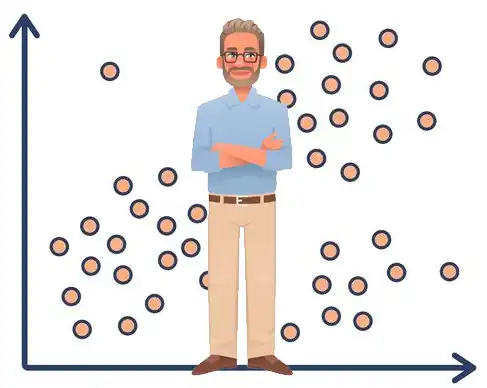
Advantages and disadvantages of stepwise regression
Stepwise regression has many advantages over other regression methods. Here are a few:
- The capability to manage a huge amount of potential predictor variables, which helps fine-tune regression models to select the most appropriate predictor variables from the given options.
- It is much faster than other automated model selection methods
- Since stepwise regression allows you to watch how variables are added or removed from the models, you can obtain valuable information about the nature and quality of the available predictor variables.
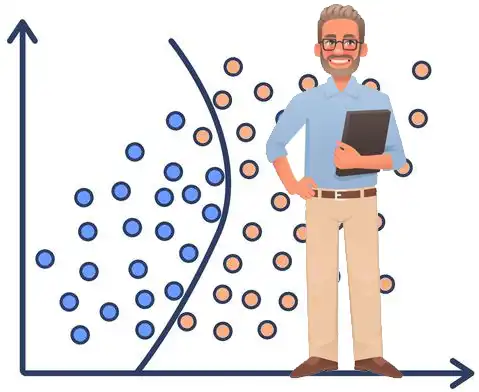