Two-way scatter Plot
Trump_clinton_2pvdiff r_d_2pvdiff_2012
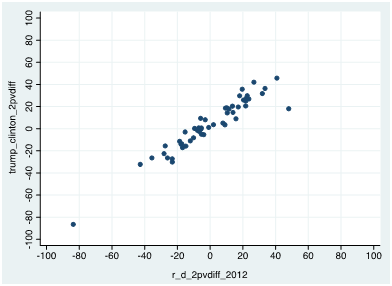
- Things to look for in a scatterplot:
i. Units of measurement and range of variation of the variables.
- Percentage points. The distance between the Republican candidate and the Democratic one.
- Similar between 2012 and 2016 (the data are closely organized). Look:
gen diff1216=trump_clinton_2pvdiff - r_d_2pvdiff_2012
sum diff1216
Variable | Obs Mean Std. Dev. Min Max
-------------+---------------------------------------------------------
diff1216 | 51 3.687255 7.318076 -29.96 16.1
graph box r_d_2pvdiff_2012 trump_clinton_2pvdiff diff1216
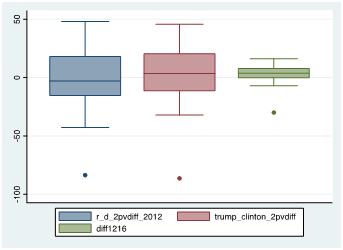
What do you observe?
kdensity r_d_2pvdiff_2012, addplot(kdensity trump_clinton_2pvdiff)

What do you observe?
tabstat r_d_2pvdiff_2012 trump_clinton_2pvdiff diff1216, stat(mean median sd min max range)
stats | r_d~2012 trump_~f diff1216
---------+------------------------------
mean | -0.03 3.66 3.69
p50 | -2.98 3.54 3.80
sd | 23.54 23.77 7.32
min | -83.63 -86.41 -29.96
max | 48.04 45.77 16.10
range | 131.67 132.18 46.06
----------------------------------------
ii. Outliers (extreme values in the main pattern of the data) and high-leverage values (values that deviate from the main pattern)
Yes, one outlier and one observation with high leverage.
Interestingly, the high-leverage observation cannot be visualized in box plots, density plots, or histograms.

iii. Tendency/direction of the data (positive or negative association).
Positive association: Values in the Y-axis increase as we move from left to right in the X-axis.
iv. Type of association (e.g., linear, nonlinear)
Linear. All the data points are organized in a linear pattern. The only data point that deviates from the linear pattern is the state with high leverage (Utah).
Utah deviates from the linear pattern because, in 2016, the two-party vote distance was much smaller than expected from 2012 (in 2012 it was almost 49 percentage points; in 2016 about 19 pp — i.e., a 30 pp difference: a reduction of about 60%).
v. Dispersion of the data: Strength of the association (strong, moderate, weak)
The data are tightly organized in a linear pattern, meaning that the relationship is strong.
We can actually measure how strong is an association using the Pearson’s Correlation Coefficient:
It varies from -1 (strongest possible [linear] negative association) to 1 (strongest possible [linear] positive association).
A value of 0 indicates there is no association between the variables.
Here are a couple of visualizations:
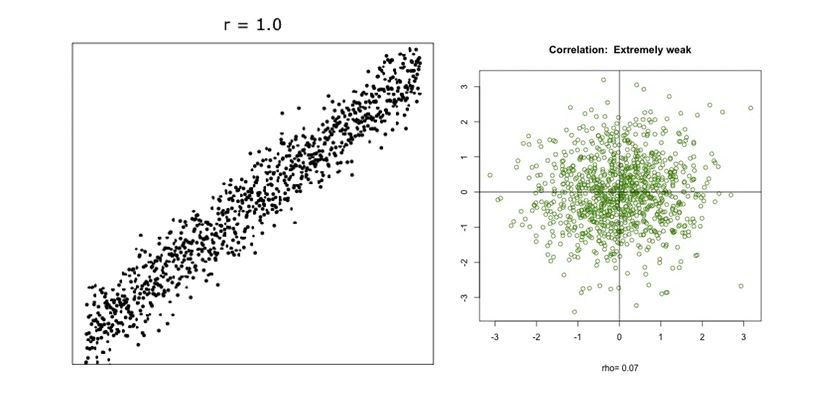
In Stata, we can calculate the correlation coefficient by typing the command corr.
For example, let’s see how correlated are the two-party vote difference in 2012, 2016, and the percentage of the state’s population that lives in urban areas:
corr trump_clinton_2pvdiff r_d_2pvdiff_2012 perct_urban
| trump_~f r_d~2012 perct_~n
-------------+---------------------------
trump_clin~f | 1.0000
r_d_2pv~2012 | 0.9522 1.0000
perct_urban | -0.5633 -0.4259 1.0000
Correlation Between Two Variables
As expected, the correlation between the two variables measuring the two-party vote share differences is positive and very high (=0.95). Differently, the correlation between these two variables and a states’ urban populationis negative and moderately-highly correlated (= -0.56 and -0.43).
In social science data, approximately:
- 0-10: Very low correlation
- 0-20: Low
- 20-40: moderate
- 40-60: moderate-high
- 60+: high
Here are the three scatterplots:

Please interpret the negative association between urban population and two-party vote share differnces.
vi. Clustering/grouping/locations of the data (sources of diversity and inequality)
Many times, it is not only about observing clusters in the data, but also identifying key locations in the scatterplot that bring meaning to the analysis. For homework 5, please briefly:
a. Interpret data values above and under “0” in the Y-axis.
b. Interpret data values to the left and right of “0” in the X-axis.
c. Interpret data values located in the four quadrants of the scatterplot.
d. Interpret data values close to the “0” intersection.
e. What would be the meaning of a 45-degree line?
Interpret data values above and under the 45-degree line.
f. Using only the interpretations of the data (“a” through “e” above), offer a brief description of the factors that, relative to the partisan political climate in 2012, led Donald Trump to victory in 2016. What is the basic story the data are telling? If possible, use quantifications to back up the story.
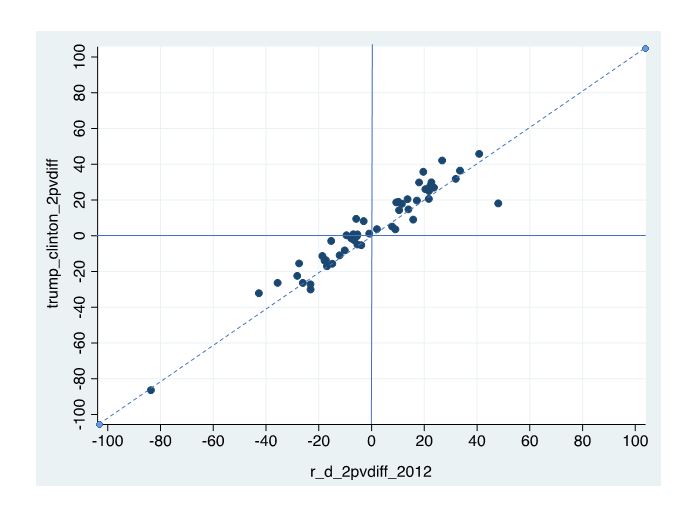
The 2016 election and healthy life expectancy
The healthy life expectancy at birth is the average number of years a newborn can expect to live in good health. It is, therefore, a variable that captures much of the factors that go in enjoyingthe good things in life, accessing the benefits of civilization, and the wealth and development of our time.A healthy life expectancy starts in our homes, schools, workplaces, neighborhoods, and communities. It is determined by access to social and economic opportunities; the healthcare resources and supports available where we are born, live, and age; the quality of our schooling; the safety and freedom of our workplaces; the cleanliness of our water, food, and air; and the nature of our social interactions and relationships. Is the healthy life expectancy related to voting behavior? Are people supporting or punishing politicians on the basis of receiving or not what gives them a good and healthy life?
Here are some useful correlations:
corr trump_clinton_2pvdiff hle90 hle16 diffhle
| trump_~f hle90 hle16 diffhle
-------------+------------------------------------
trump_clin~f | 1.0000
hle90 | 0.1902 1.0000
hle16 | -0.5078 0.6656 1.0000
diffhle | -0.8580 -0.3841 0.4334 1.0000
What do you observe?
Here are some useful scatterplots:
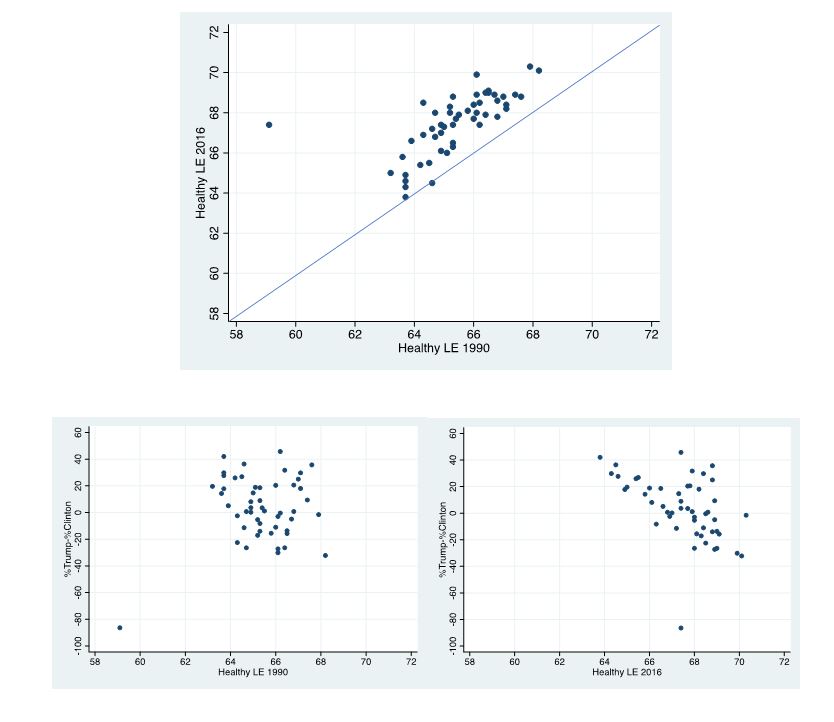
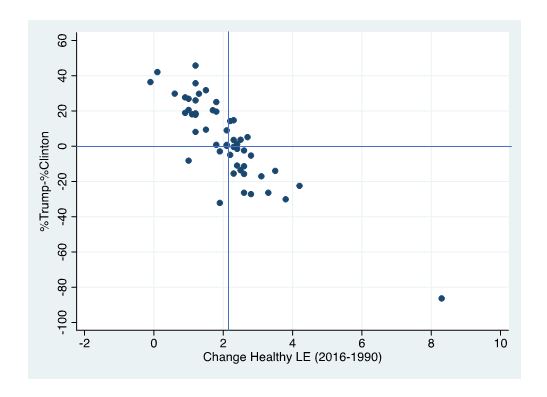
Important groups of states? How about battleground states? How many of them, say within a range of 6 pp (against and in favor of Trump in 2016)?
list state trump_clinton_2pvdiff if trump_clinton_2pvdiff<6 & trump_clinton_2pvdiff>-6
+---------------------------+
| state trump_~f |
|---------------------------|
3. | Arizona 3.54 |
6. | Colorado -4.91|
10. | Florida 1.2 |
11. | Georgia 5.13 |
20. | Maine -2.96 |
|---------------------------|
23. | Michigan .23 |
24. | Minnesota -1.52 |
29. | Nevada -2.42 |
30. | New Hampshire -.37 |
34. | North Carolina 3.66 |
|---------------------------|
39. | Pennsylvania .72 |
47. | Virginia -5.32 |
50. | Wisconsin .77 |
+---------------------------+
That’s 13 states; 7 favored Trump in 2016. Given that a pandemic hit in 2020, and pandemics hit healthy life expectancy, what do you think happened to these 13 states?
+---------------------------+
| statetrump-Biden
|---------------------------|
3. | Arizona -0.3|
6. | Colorado -13.5|
10. | Florida 3.4 |
11. | Georgia -0.2|
20. | Maine -9.0|
|---------------------------|
23. | Michigan -2.8|
24. | Minnesota -7.1|
29. | Nevada -2.4 |
30. | New Hampshire -7.4|
34. | North Carolina 0.1 |
|---------------------------|
39. | Pennsylvania -0.1|
47. | Virginia -10.1|
50. | Wisconsin -0.6|
+---------------------------+
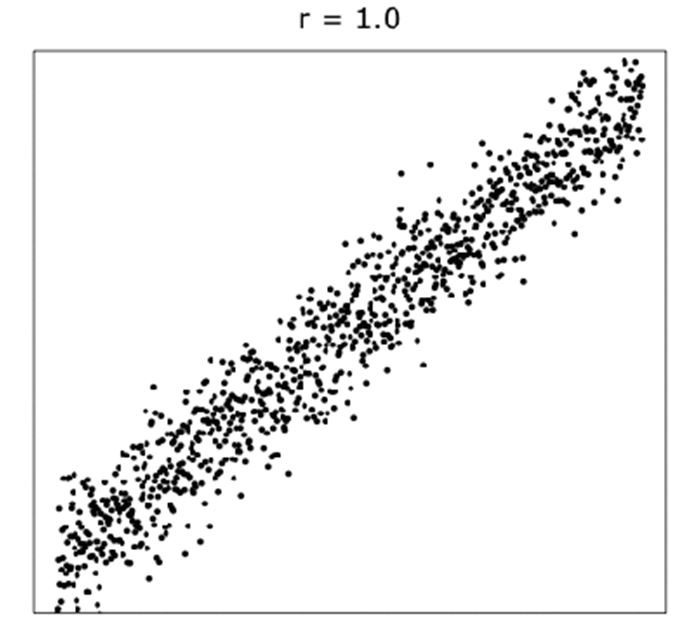
Scatter Plots Interpretation Solutions
• The first chart above has a negative correlation while the second and third chart has a positive correlation.
• With y=0 on the y axis it means that there is an intercept of zero i.e. no correlation
• The left and right of 0 shows that there is a negative correlation between the Republican and Democratic candidate in the USA election.
• The four quadrant describes the data sets used for the USA election between the Republican and the Democratic candidate. The first quadrant shows weak positive correlation, the second quadrant shows a strong positive correlation, the third quadrant shows a strong negative correlation while the fourth quadrant show no correlation between the Republican and Democratic candidate in the USA election.
• The values to y=0 shows a strong correlation between the datasets.
• The values close to the zero intersection shows how close the two candidates her to one another in the USA election.
• The values under the 45 degree line shows where the aggregate between the Republican and Democratic candidates are equal.
• The factors that led to Trump winning in 2016 based on the 2012 election is stated below;
The number of people electing not to vote for the Republican or Democratic nominee went up by 4.5 million votes, nearly tripling from 2012. Young voters were the same share of the electorate (19 percent), but went in smaller margins for Clinton than Obama and they jumped in third-party support from 3 percent to 8 percent. They were a group Democrats used to compete with. In 1992 and 1996, Bill Clinton won them by a point. But they have fled to the GOP in the years since and now the gap between whites with college degrees and without appears to be the widest ever. A whopping 35 points. Overall, the reason Trump won was because he flipped big margins with white, working class voters in the Midwest and Pennsylvania something that was always a possibility. Black voters were down as a share of the electorate slightly and went for Clinton in a smaller margin more like 2004 numbers for John Kerry. 2012: 13% of electorate, 93-6 Obama, 2016: 12% of electorate, 88-8 Clinton. Latinos went up as a share of the electorate from 10 percent to 11 percent, but the idea that they would turn out for Clinton in bigger numbers than for Obama because of Trump turned out just not be true overall and a significant share, especially among Latinos.