Claim Your Discount Today
Get 10% off on all Statistics homework at statisticshomeworkhelp.com! Whether it’s Probability, Regression Analysis, or Hypothesis Testing, our experts are ready to help you excel. Don’t miss out—grab this offer today! Our dedicated team ensures accurate solutions and timely delivery, boosting your grades and confidence. Hurry, this limited-time discount won’t last forever!
We Accept
View Our Collection of Free Multivariate Analysis Homework Samples
Explore our collection of free multivariate analysis homework samples at statisticshomeworkhelper.com. These samples showcase the high-quality multivariate analysis homework help we offer. If you’re looking for help with multivariate analysis homework, our samples reflect the expertise of our multivariate analysis homework experts. For comprehensive statistics homework help, review our free examples and see how our services can assist you in mastering your assignments.
Statistics
Statistics
Statistics
Statistics
Statistics
Statistics
Statistics
Statistics
Statistics
Statistics
Statistics
Statistics
Statistics
Statistics
Statistics
Statistics
Statistics
Statistics
Time Series Analysis
Statistical Models
Get Affordable Multivariate Analysis Homework Help from Us
Get affordable multivariate analysis homework help from statisticshomeworkhelper.com. Our multivariate analysis homework experts provide top-notch help with multivariate analysis homework at student-friendly prices. We offer reliable statistics homework help to ensure you excel in your assignments without breaking the bank. Trust us for cost-effective, professional assistance tailored to your needs.
Description | PriceRange |
---|---|
Basic Multivariate Analysis Concepts | $20 - $40 |
Intermediate Multivariate Techniques (PCA, FactorAnalysis) | $45 - $70 |
Advanced Multivariate Analysis Skills (ClusterAnalysis, MANOVA) | $75 - $110 |
Complex Multivariate Analysis Solutions (DetailedData Interpretation) | $130 - $220+ |
Rapid Service for Urgent Multivariate Analysis Homeowrk | Additional 50% |
Personalized Consultation and Assistance | $25 - $60 per hour |
- Multivariate Analysis Homework Help
- Why Online Multivariate Analysis Homework Help is Valuable for Students?
- Exclusive Advantages of Choosing Our Specialized Multivariate Analysis Homework Help Service
- Different Topics Handled by Our Expert Multivariate Analysis Homework Help Service
- Step-by-Step Process for Using Our Multivariate Analysis Homework Help Service
- We Provide Unlimited Free Revisions to Ensure Your Homework Meets Expectations
Multivariate Analysis Homework Help
At statisticshomeworkhelper.com, we offer comprehensive multivariate analysis homework help tailored to meet your academic needs. Our team of experienced multivariate analysis homework experts provides in-depth assistance with a wide range of topics, from dimensionality reduction to cluster analysis. Whether you're struggling with complex multivariate techniques or need clear explanations, our experts are here to provide the support you need.
We understand that each student’s requirements are unique, which is why we offer personalized help with multivariate analysis homework. Our dedicated professionals ensure that you receive high-quality solutions that adhere to your academic guidelines. Additionally, we offer extensive statistics homework help, covering various statistical methods and analyses to help you excel in your coursework.
With statisticshomeworkhelper.com, you can trust that you are receiving expert guidance and detailed assistance for all your multivariate analysis homework needs. Our goal is to help you achieve academic success by providing reliable, timely, and effective support. Don't hesitate to reach out and experience the difference our expert help can make in your studies.
Why Online Multivariate Analysis Homework Help is Valuable for Students?
Online multivariate analysis homework help is invaluable for students due to the complexity and challenges of the subject. Multivariate analysis involves intricate techniques that require a high level of precision, which can be daunting under tight deadlines and high academic expectations. By seeking help with multivariate analysis homework from a subject matter expert, students can ensure that their work meets accuracy requirements and adheres to academic standards.
Statistics homework help from experts at statisticshomeworkhelper.com provides tailored support to address these challenges, alleviating the pressure and helping students achieve better grades while managing their workload effectively. If you are concerned about who will do my multivariate analysis homework? Rest assured, statisticshomeworkhelper.com is specially designed to provide expert assistance with your multivariate analysis homework!
- Fear of Failure: Students often experience fear of failure with multivariate analysis due to its complexity, leading to anxiety and stress over potential poor grades.
- Desire for Higher Grades: The desire for higher grades drives students to seek help, as they need accurate and expert assistance to excel in challenging multivariate analysis assignments.
- Complex Techniques: Multivariate analysis involves complex techniques that can overwhelm students, making it difficult to grasp and apply advanced statistical methods correctly.
- Tight Deadlines: Tight deadlines add pressure, forcing students to manage extensive multivariate analysis tasks quickly, often resulting in incomplete or inaccurate work without proper support.
- High Academic Expectations: High academic expectations require precise and in-depth analysis, causing stress as students strive to meet these standards and produce flawless multivariate analysis solutions.
- Accuracy Requirement: The accuracy requirement in multivariate analysis is crucial, and the fear of making errors can be paralyzing, prompting students to seek expert assistance for reliable results.
Exclusive Advantages of Choosing Our Specialized Multivariate Analysis Homework Help Service
Choosing our specialized multivariate analysis homework help service at statisticshomeworkhelper.com offers exclusive advantages tailored to meet your academic needs. Our multivariate analysis homework experts, with years of experience in the field, provide in-depth assistance and ensure high-quality results. With our help with multivariate analysis homework, you benefit from personalized support that addresses the complexities of multivariate techniques. Additionally, we offer comprehensive statistics homework help, covering various statistical methods to enhance your performance. Our statistics assignment help service is designed to give you effective solutions for your coursework.
Our service is designed to provide accurate, timely solutions, helping you achieve better grades and manage your coursework effectively. Experience the unique benefits of expert guidance and tailored support with our specialized service. If you are concerned about who will do my multivariate analysis homework? Don’t worry, our dedicated team is ready to provide expert multivariate analysis homework assistance tailored to your academic needs!
- Expert Guidance: Receive assistance from multivariate analysis homework experts who offer detailed explanations and solutions tailored to your specific academic needs and challenges.
- Timely Delivery: Enjoy prompt service with on-time delivery for your multivariate analysis homework, ensuring you meet deadlines and manage your coursework efficiently.
- Personalized Support: Get customized help with multivariate analysis homework designed to address your unique questions and difficulties, providing a tailored approach to your assignments.
- Comprehensive Solutions: Benefit from thorough and accurate statistics homework help, covering various techniques and methods to ensure your assignments are well-researched and precise.
- 24/7 Availability: Access our services any time of day with our round-the-clock support, providing continuous multivariate analysis homework help for all your urgent needs.
- Affordable Pricing: Receive high-quality multivariate analysis homework assistance at competitive rates, making expert help accessible without breaking your budget.
Different Topics Handled by Our Expert Multivariate Analysis Homework Help Service
Our multivariate analysis homework help service at statistics homework helper covers a wide range of topics to support your academic needs. Our multivariate analysis homework experts provide detailed help with data analysis and statistical techniques on subjects such as factor analysis, cluster analysis, and canonical correlation. We also offer expert guidance on complex multivariate statistics techniques and methods, including multiple regression and regression analysis, ensuring comprehensive statistics homework help.
Whether you need assistance with statistical softwares or expert advice from experienced data analysts, our service is designed to meet all your academic requirements. Whether you’re dealing with advanced concepts or basic applications, our professional statistics homework help service is designed to deliver accurate and tailored solutions to enhance your performance in multivariate analysis. If you are concerned about who will do my multivariate analysis homework? We are ready to provide expert assistance with any level of your multivariate analysis homework!
- Factor Analysis: We provide expert assistance in factor analysis, helping you understand how to identify underlying variables and reduce data dimensions for comprehensive results.
- Cluster Analysis: Our service covers cluster analysis, guiding you through techniques to group similar data points, identify patterns, and derive meaningful insights from your data sets.
- Canonical Correlation: Get help with canonical correlation analysis to explore the relationships between two sets of variables and understand their interdependencies effectively.
- Principal Component Analysis (PCA): We offer support for principal component analysis (PCA), aiding in dimensionality reduction and simplifying complex data while retaining essential features.
- Discriminant Analysis: Our experts assist with discriminant analysis, which helps classify data into predefined categories and understand the differences between groups based on multiple variables.
- Structural Equation Modeling (SEM): Receive guidance on structural equation modeling (SEM), allowing you to test theoretical models and understand complex relationships among variables in your data.
Step-by-Step Process for Using Our Multivariate Analysis Homework Help Service
To access our multivariate analysis homework help service at statisticshomeworkhelper.com, follow these simple steps. First, provide your homework requirements, detailing the specific challenges you face. Next, our multivariate analysis homework experts will review your needs and offer a tailored solution. You’ll receive expert help with multivariate analysis homework and guidance throughout the process.
For additional statistics homework help, just indicate your needs. Our streamlined process ensures that you get accurate and timely assistance to excel in your assignments. If you are concerned about who will do my multivariate analysis homework? Contact us today to get expert assistance with your multivariate analysis homework!
- Submit Your Requirements: Provide detailed information about your multivariate analysis homework needs, including specific topics and challenges, to help our experts tailor the assistance.
- Receive Expert Review: Our multivariate analysis homework experts will evaluate your requirements and create a customized plan to address your help with multivariate analysis homework needs.
- Get a Quote: Obtain a detailed quote based on the complexity and scope of your assignment. This ensures transparency in pricing for our statistics homework help.
- Approve and Proceed: Review the proposed plan and quote. Once approved, our team will start working on your multivariate analysis homework with expert precision.
- Receive Your Solution: Get the completed assignment within the agreed timeframe, ensuring it meets all academic requirements and addresses your specific challenges.
- Request Revisions if Needed: If any adjustments are required, request revisions. Our multivariate analysis homework experts will make necessary changes to ensure your satisfaction and academic success.
We Provide Unlimited Free Revisions to Ensure Your Homework Meets Expectations
At statisticshomeworkhelper.com, we are committed to ensuring that your assignments meet your expectations through our policy of providing unlimited free revisions. Our primary goal is to offer the best possible multivariate analysis homework help tailored to your specific needs.
When you seek help with multivariate analysis homework, you can trust that our dedicated multivariate analysis homework experts will deliver high-quality solutions. However, if you require adjustments or refinements, we are here to make any necessary changes without additional cost.
Our statistics homework help service is designed to provide comprehensive and accurate assistance, and our unlimited revision policy ensures that every detail of your homework meets the required standards. This approach guarantees that you receive the most precise and effective help possible, aligning perfectly with your academic requirements.
By offering unlimited revisions, we aim to eliminate any concerns about the quality of your work, allowing you to focus on achieving the best results. At statisticshomeworkhelper.com, we stand by our commitment to your satisfaction, providing flexible support until your multivariate analysis homework is completed to your satisfaction.
Read Our Detailed Multivariate Analysis Homework Blogs
Explore our detailed blogs for multivariate analysis homework help at statisticshomeworkhelper.com. Our articles provide valuable insights and tips for tackling complex topics. Whether you need help with multivariate analysis homework or guidance from a multivariate analysis homework helper, our blogs are designed to support your academic success.
Hear from Our Clients: Real Reviews about Our Multivariate Analysis Homework Help
Hear directly from our clients about their experiences with our multivariate analysis homework help. Discover how they benefited from our expert help with multivariate analysis homework and the effectiveness of our multivariate analysis homework helpers. Their reviews reflect the quality and reliability of our services, showcasing how we assist students in mastering their assignments.
Connect with Our Multivariate Analysis Homework Help Experts for Personalized Guidance
Connect with our multivariate analysis homework help experts for personalized guidance. Whether you need help with multivariate analysis homework or are looking for a skilled multivariate analysis homework helper, our team is here to provide tailored support. Get expert advice and solutions customized to your needs for successful academic results.
Dr. Clara Mitchell
Ph.D. in Quantitative Methods
🇦🇺 Australia
Dr. Clara Mitchell earned her Ph.D. in Quantitative Methods from the University of Guelph. With 760 homework completed and over 10 years of experience, she specializes in delivering precise and actionable statistical analyses. Dr. Mitchell is known for her ability to handle both basic and advanced topics with efficiency and clarity.
MR. Aakash Patel
Master’s in Applied Statistics
🇮🇳 India
MR. Aakash Patel obtained his Master’s in Applied Statistics from the University of Delhi. With over 500 homework completed and 7 years of experience, he is highly regarded for his ability to simplify complex topics and provide structured solutions. His consistent track record makes him a top choice for social science statistics homework help.
Professor Fisher Griffiths
Master’s degree in Statistics
🇦🇺 Australia
Professor Fisher Griffiths holds a Master’s degree in Statistics from Aberystwyth University. With over 420 homework completed and 8 years of experience, he is known for his practical and hands-on approach. His focus on delivering thorough and detailed solutions makes him a trusted resource for students in need of reliable assistance.
Dr. Lila Monroe
Ph.D. in Statistics
🇦🇪 United Arab Emirates
Dr. Lila Monroe earned her Ph.D. in Statistics from the College of William & Mary. She has completed over 350 homework during her 9 years of experience, helping students master complex statistical concepts. Dr. Monroe’s expertise lies in delivering clear and concise solutions to intricate problems across various domains of social science statistics.
Mr. Dylan Reese
Master’s degree in Statistics
🇸🇬 Singapore
Mr. Dylan Reese holds a Master’s degree in Statistics from Cardiff University. With 930+ homework on his belt, Mr. Dylan brings 6 years of experience to the table. His professional background includes contributions at Aberystwyth University and the University of South Wales, specializing in statistical applications and data interpretation.
Dr. Amelia Hayes
Ph.D. in Statistics
🇸🇬 Singapore
Dr. Amelia Hayes completed her Ph.D. in Statistics at Simon Fraser University. Over her 7-year career, she has tackled 930+ homework, drawing on her expertise gained at Trent University and Mount Royal University. Her work involves advanced statistical techniques and practical applications for diverse data sets.
Professor Oliver Langley
MSc in Statistics
🇬🇧 United Kingdom
Professor Oliver Langley obtained his MSc in Statistics from the University of Leicester. With more than 810+ homework completed, he has 10 years of academic and practical experience. He has contributed significantly to the field through his work at the University of Essex and Keele University, focusing on various statistical methodologies.
Dr. Evelyn Hawthorne
Ph.D. in Statistics
🇺🇸 United States
Dr. Evelyn Hawthorne holds a Ph.D. in Statistics from the University of California, Irvine. She has completed over 620 homework and brings over 8 years of experience in the field. Her career includes roles at Claremont McKenna College and Occidental College, where she refined her skills in statistical analysis and data interpretation.
Professor James Sullivan
Ph.D. in Statistics
🇺🇸 United States
Professor James Sullivan, with a Ph.D. from Cardiff University, has completed more than 770 statistical consulting projects. His extensive background in statistical methods and his commitment to high-quality work make him a trusted expert in the field. Professor Sullivan’s experience spans various statistical domains, ensuring that students receive well-rounded and effective assistance.
MR. Lin Wei
Master’s in Statistics
🇨🇦 Canada
MR. Lin Wei holds a Master’s in Statistics from the University of Toronto. With over 420 homework completed, he brings a practical, solution-oriented approach to statistical consulting. His commitment to accuracy and his ability to handle complex datasets make him an excellent choice for students seeking reliable and insightful help with their statistical homework.
Professor Ravi Patel
M.Sc. in Applied Statistics
🇬🇧 United Kingdom
Professor Ravi Patel earned his M.Sc. in Applied Statistics from the University of Alberta. With a track record of over 400 completed homework, Professor Patel is renowned for his methodical approach and in-depth understanding of statistical techniques. His extensive experience enables him to tackle diverse and challenging statistical problems with confidence and skill.
Dr. Isla Montgomery
Ph.D. in Statistics
🇨🇦 Canada
Dr. Isla Montgomery holds a Ph.D. in Statistics from the University of Wales Trinity Saint David. With over 350 homework completed, Dr. Montgomery combines deep theoretical knowledge with practical experience. Her expertise ensures precise, data-driven solutions for complex statistical problems. Her dedication and attention to detail have earned her a reputation for excellence in the field.
Professor Elwood Tiller
Master’s degree in Industrial Statistics
🇸🇬 Singapore
Professor Elwood Tiller holds a Master’s degree in Industrial Statistics from Williams College. He has completed more than 1,900 homework and brings a decade of experience to his role. Professor Tiller’s expertise lies in applying advanced statistical techniques to real-world problems, ensuring students receive thorough, actionable insights that enhance their understanding.
Dr. Rosalind Glynne
Ph.D. in Industrial Statistics
🇬🇧 United Kingdom
Dr. Rosalind Glynne earned her Ph.D. in Industrial Statistics from the University of Bristol. With over 1,450 homework completed and 8 years of experience, Dr. Glynne offers expert solutions tailored to complex statistical problems. Her approach combines rigorous analysis with a clear, methodical presentation to meet the highest academic standards.
MR. Liam Connolly
Master’s in Industrial Statistics
🇺🇸 United States
MR. Liam Connolly, with a Master’s in Industrial Statistics from Lakehead University, has managed over 1,300 homework. His 6 years of professional experience are characterized by a commitment to accuracy and clarity in every solution he provides. MR. Connolly’s skill set includes a range of statistical methods tailored to meet diverse academic needs.
Dr. Catrin Evans
Ph.D. in Industrial Statistics
🇦🇪 United Arab Emirates
Dr. Catrin Evans received her Ph.D. from Aberystwyth University in Industrial Statistics. With over 860 homework completed and 7 years of expertise, Dr. Evans specializes in providing detailed, reliable solutions for intricate statistical challenges. Her work is known for its precision and the ability to convey complex concepts in an accessible manner.
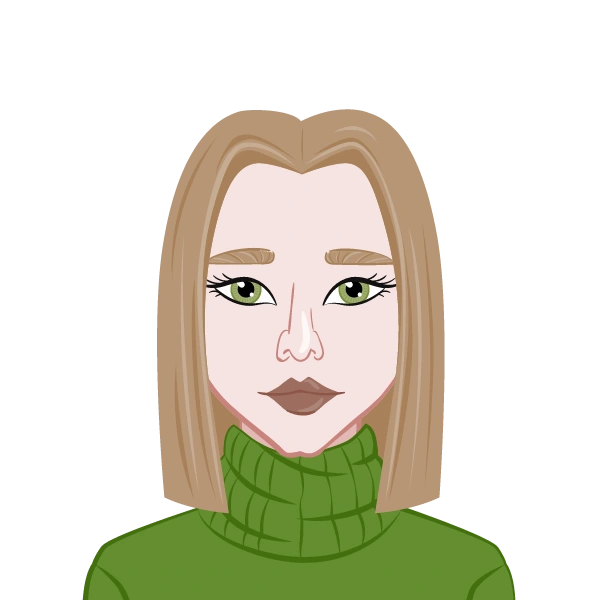
Dr. Lena Zhao
Ph.D. in Economics
🇨🇦 Canada
Dr. Lena Zhao received her Ph.D. in Quantitative Methods from the University of Toronto. With over 630 homework under her belt, she possesses extensive experience and a thorough understanding of business statistics. Dr. Zhao is celebrated for her ability to simplify complex topics and her dedication to providing precise, high-quality solutions. Her work ensures that students not only complete their homework but also improve their grasp of the subject. Specialization Areas:
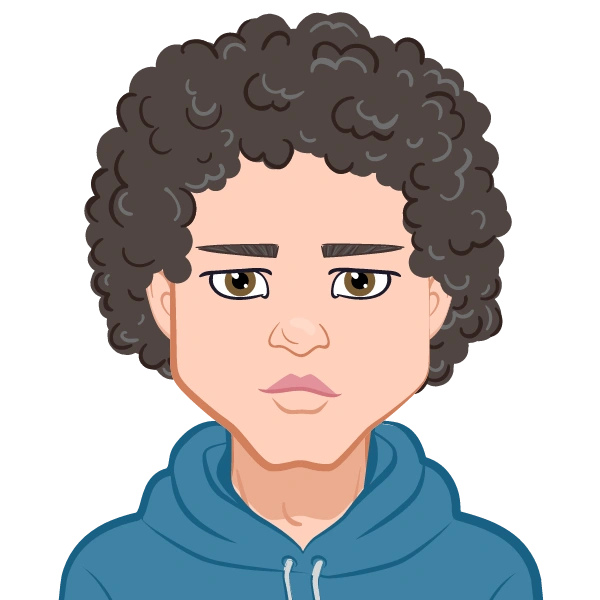
MR. Rohan Patel
Master’s degree in Applied Statistics
🇺🇸 United States
MR. Rohan Patel holds a Master’s degree in Applied Statistics from the University of Chicago. He has completed over 1,310 homework, bringing a wealth of experience in business data analysis and statistical modeling. His focus is on delivering practical and understandable solutions, guiding students through their homework effectively. MR. Patel’s expertise encompasses both academic research and applied data techniques.
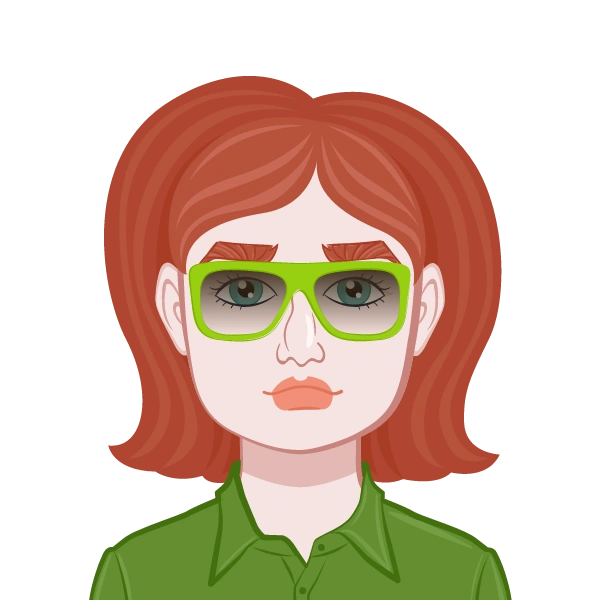
Professor Emily Chen
Ph.D. in Economics
🇺🇸 United States
Professor Emily Chen earned her Ph.D. in Statistics from the University of Chicago and has completed over 1,160 homework throughout her career. With extensive teaching and research experience, Professor Chen brings in-depth knowledge in business statistics and data analysis. Her approach merges rigorous theoretical understanding with hands-on problem-solving techniques, helping students master complex concepts and their applications.
.webp)
Dr. Alex Morgan
Ph.D. in Business Analytics
🇺🇸 United States
Dr. Alex Morgan holds a Ph.D. in Business Analytics from Stanford University. With over 940 homework completed, Dr. Morgan has more than a decade of experience assisting students with complex statistical concepts and analyses. His expertise extends beyond theoretical applications into real-world business scenarios, ensuring that students gain both knowledge and practical skills. Dr. Morgan is renowned for his clear explanations and commitment to fostering student success.
Frequently Asked Questions (FAQs)
Check out our Frequently Asked Questions (FAQs) for insights into our multivariate analysis homework help services. Find answers to common queries about how we provide help with multivariate analysis homework and what to expect from our multivariate analysis homework helpers. Our FAQs offer valuable information to ensure you get the support you need.