Claim Your Discount Today
Get 10% off on all Statistics homework at statisticshomeworkhelp.com! Whether it’s Probability, Regression Analysis, or Hypothesis Testing, our experts are ready to help you excel. Don’t miss out—grab this offer today! Our dedicated team ensures accurate solutions and timely delivery, boosting your grades and confidence. Hurry, this limited-time discount won’t last forever!
We Accept
Check Out Our Collection of Mathematical Statistics Homework Samples
Check out our collection of mathematical statistics homework samples at statisticshomeworkhelper.com. These samples showcase the quality of our help with mathematical statistics homework and highlight the expertise of our mathematical statistics homework experts. Whether you need statistics homework help or detailed solutions, our samples illustrate our commitment to excellence in providing top-notch mathematical statistics homework help. Explore our examples to see how we can assist with your assignments.
Statistics
Statistics
Statistics
Statistics
Statistics
Statistics
Statistics
Statistics
Statistics
Statistics
Statistics
Statistics
Statistics
Statistics
Statistics
Statistics
Statistics
Statistics
Time Series Analysis
Statistical Models
View Our Budget-Friendly Pricing for Exceptional Mathematical Statistics Homework Help
View our budget-friendly pricing for exceptional mathematical statistics homework help at statisticshomeworkhelper.com. Our rates offer excellent value for help with mathematical statistics homework, provided by experienced mathematical statistics homework experts. Whether you need statistics homework help or specialized assistance, our affordable pricing ensures you receive top-quality support without breaking the bank. Explore our pricing options and see how we can assist with your homework effectively and economically.
Description | Price Range |
---|---|
Basic Mathematical Statistics Homework Concepts | $20 - $40 |
Intermediate Mathematical Statistics Homework Techniques | $45 - $70 |
Advanced Mathematical Statistics Homework Methods | $75 - $110 |
Complex Mathematical Statistics Homework Solutions | $130 - $220+ |
Rapid Service for Urgent Mathematical Statistics Homework | Additional 50% |
Personalized Consultation and Assistance | $25 - $60 per hour |
- Mathematical Statistics Homework Help
- Top Reasons for Students to Investing in Online Mathematical Statistics Homework Help
- Experience the Advantages of Our Outstanding Mathematical Statistics Homework Help Service
- Discover the Range of Topics Addressed by Our Mathematical Statistics Homework Help Service
- Simple Steps to Connect with Our Experienced Mathematical Statistics Homework Specialists
- We Guarantee On-Time Delivery for All Your Mathematical Statistics Homework
Mathematical Statistics Homework Help
At statisticshomeworkhelper.com, we offer comprehensive mathematical statistics homework help to support students at all levels. Our dedicated mathematical statistics homework experts are here to provide tailored help with mathematical statistics homework.
Whether you need assistance with complex statistical theories, data analysis, or specific mathematical problems, our team is equipped to offer effective solutions. Our statistics homework help covers a wide range of topics, including probability, regression analysis, and hypothesis testing, ensuring you get the precise support you need for your assignments.
With years of experience, our experts deliver high-quality, original work that meets academic standards and helps you understand difficult concepts. Choose statisticshomeworkhelper.com for reliable and efficient mathematical statistics homework help.
Our commitment to excellence and personalized assistance ensures that you receive the best support for your mathematical statistics homework challenges. If you are worried about who will do my mathematical statistics homework? Trust us to guide you through every step of your mathematical statistics homework with professional expertise and dedicated care.
Top Reasons for Students to Investing in Online Mathematical Statistics Homework Help
Investing in online mathematical statistics homework help offers significant advantages for students facing complex assignments and tight deadlines. With high academic expectations and the need for precise results, having access to a mathematical statistics homework expert ensures you receive accurate and reliable solutions. Our team provides expert help with mathematical statistics homework to address challenges and simplify complex topics, relieving pressure and improving your performance.
At statisticshomeworkhelper.com, our statistics homework help is designed to meet your academic needs, ensuring you achieve the best results even under deadline constraints. If you are worried about who will do my mathematical statistics homework? You can choose our online service to manage your mathematical statistics homework demands effectively.
- Complexity of Topics: Mathematical statistics homework often involves intricate concepts. Expert help simplifies these topics, making them more accessible and manageable, thus enhancing overall comprehension and assignment quality.
- Deadline Pressure: Tight deadlines can stress students. Online mathematical statistics homework help provides timely assistance, ensuring assignments are completed on schedule without sacrificing quality or accuracy.
- High Academic Expectations: Students face high expectations from their academic programs. Professional help with mathematical statistics homework ensures adherence to rigorous standards, meeting and exceeding academic requirements effectively.
- Accuracy Requirements: Precision is critical in mathematical statistics homework. Professional help ensures all calculations and analyses are accurate, preventing errors that could negatively impact grades and understanding.
- Desire for a Good Grade: Achieving a high grade in mathematical statistics homework is crucial. Online help ensures accuracy and thorough understanding, contributing to improved academic performance and desirable grades.
- Fear of Failure: Students fear poor grades due to challenging mathematical statistics homework. Professional help mitigates this fear by providing expert guidance and boosting confidence in their work.
Experience the Advantages of Our Outstanding Mathematical Statistics Homework Help Service
Experience the advantages of our exceptional mathematical statistics homework help service. At statisticshomeworkhelper.com, we provide top-notch mathematical statistics assignment writing service, ensuring you receive accurate and comprehensive support. Our support team offers professional statistics homework help online, addressing your specific needs and challenges. Benefit from tailored and plagiarism free solutions, timely assistance, and a commitment to academic excellence.
With our expert guidance, you’ll achieve better understanding and improved grades, all while managing your workload efficiently. Discover how our dedicated support can make a difference in your academic journey. If you are worried about who will do my mathematical statistics homework? Rest assured, our team is ready to provide exceptional mathematical statistics homework help for your academic success!
- Expert Assistance: Access to highly qualified mathematical statistics homework experts with extensive experience and advanced degrees, ensuring top-quality support and accurate solutions.
- Personalized Solutions: Tailored help with mathematical statistics homework that addresses your specific needs and academic challenges, providing customized guidance and support.
- Timely Delivery: Prompt service with guaranteed on-time delivery, ensuring you meet deadlines without compromising the quality of your mathematical statistics homework.
- Comprehensive Support: Extensive statistics homework help covering a wide range of topics, from basic concepts to complex analyses, ensuring thorough assistance across all areas of mathematical statistics.
- High-Quality Work: Commitment to producing original, well-researched, and meticulously checked assignments, enhancing your understanding and performance in mathematical statistics homework.
- 24/7 Availability: Round-the-clock support to assist you whenever you need, providing flexibility and convenience for managing your mathematical statistics homework requirements.
Discover the Range of Topics Addressed by Our Mathematical Statistics Homework Help Service
Discover the broad range of topics covered by our Mathematical Statistics Homework Help service. At statistics homework helper, our mathematical statistics homework experts provide expert help with mathematical statistics homework across various subjects. From probability theory and hypothesis testing to regression analysis and data interpretation, we offer professional statistics assignment help tailored to your needs. Whether you're tackling basic concepts or advanced statistical models, our dedicated team ensures you receive accurate and insightful support for stats homework, addressing complex statistics problems with effective problem solving strategies.
Explore different types of statistics, improve your data collection techniques, and get assistance with statistical software and statistical courses. Explore how our service can assist you with any aspect of your mathematical statistics homework and enhance your academic performance. If you are worried about who will do my mathematical statistics homework? Don’t worry, our team is ready to help you with any level of your mathematical statistics homework for your academic excellence!
- Probability Theory: Understand the fundamentals of probability, including distributions, random variables, and expected values. Our mathematical statistics homework experts clarify complex concepts for precise application.
- Hypothesis Testing: Learn about formulating and testing hypotheses, including p-values, confidence intervals, and error types. We provide detailed help with mathematical statistics homework for accurate statistical inference.
- Regression Analysis: Explore linear and non-linear regression models, including multiple regression and correlation techniques. Our reliable statistics homework help ensures you grasp complex relationships in data effectively.
- Statistical Inference: Master methods for making predictions and generalizations about populations from sample data. Our experts offer thorough mathematical statistics homework help for reliable statistical conclusions.
- Descriptive Statistics: Learn to summarize and interpret data using measures like mean, median, mode, and standard deviation. Our service provides clear explanations for effective mathematical statistics homework solutions.
- Analysis of Variance (ANOVA): Understand ANOVA techniques to compare means across multiple groups. Our mathematical statistics homework experts guide you through complex analyses for accurate results and interpretations.
Simple Steps to Connect with Our Experienced Mathematical Statistics Homework Specialists
Connecting with our experienced mathematical statistics homework specialists is easy and straightforward. At statisticshomeworkhelper.com, simply submit your homework requirements to receive top-notch mathematical statistics homework help. Our team of mathematical statistics homework experts is ready to provide help with mathematical statistics homework, ensuring your needs are met efficiently.
We offer comprehensive statistics homework help tailored to your specific challenges, delivering high-quality and timely solutions. Engage with our experts to streamline your homework process and enhance your academic performance. If you are worried about who will do my mathematical statistics homework? Contact us today to get expert mathematical statistics homework assistance!
- Submit Your Requirements: Provide detailed information about your mathematical statistics homework needs. Include specific topics and deadlines to ensure our experts deliver precise and timely help with mathematical statistics homework.
- Receive a Quote: Get a personalized quote based on the complexity and scope of your assignment. Our mathematical statistics homework experts offer competitive pricing for quality statistics homework help.
- Review the Proposal: Examine the proposed plan and confirm the details. Our team will outline how they will address your needs for mathematical statistics homework help, ensuring clarity before starting.
- Make the Payment: Complete the payment process securely. Once payment is received, our mathematical statistics homework experts will begin working on your assignment promptly.
- Get Regular Updates: Stay informed with progress updates. Our experts provide ongoing communication to keep you updated on the status of your mathematical statistics homework and any developments.
- Receive Your Completed Homework: Get your finished assignment delivered on time. Review the high-quality solutions provided by our statistics homework help team and ensure everything meets your expectations.
We Guarantee On-Time Delivery for All Your Mathematical Statistics Homework
At statisticshomeworkhelper.com, we guarantee on-time delivery for all your mathematical statistics homework. Our commitment is to provide prompt and reliable mathematical statistics homework help, ensuring you meet every deadline without stress.
We understand the importance of timely submissions, and our mathematical statistics homework experts are dedicated to delivering high-quality solutions within your specified timeframe. Our process is designed to ensure that you receive the best help with mathematical statistics homework while adhering to strict deadlines.
From the moment you submit your requirements, our team works efficiently to provide statistics homework help that meets your needs and exceeds your expectations. We prioritize punctuality and reliability in every aspect of our service. By choosing our expert assistance, you benefit from our proven track record of delivering assignments on schedule.
Trust statisticshomeworkhelper.com for consistent, on-time delivery of your mathematical statistics homework and experience the ease of having your homework handled by professionals who value your deadlines as much as you do.
Read Our Blogs for Expert Guidance on Mathematical Statistics Homework
Read our blogs for expert guidance on mathematical statistics homework. Our posts offer valuable insights and tips to enhance your understanding and improve your assignments. Whether you need help with mathematical statistics homework or are seeking advice from a mathematical statistics homework helper, our content is designed to assist you in mastering complex topics. Explore our resources to get the best mathematical statistics homework help available.
Positive Reviews from Students Who Used Our Mathematical Statistics Homework Help
Read positive reviews from students who benefited from our mathematical statistics homework help. They praised the exceptional help with mathematical statistics homework and appreciated the expertise of our mathematical statistics homework helpers. Many highlighted how our tailored assistance made complex topics easier to understand and improve their grades. Discover how our mathematical statistics homework help has made a difference for students like you.
Learn More About Our Capable Mathematical Statistics Homework Helpers
Learn more about our capable mathematical statistics homework helpers who are dedicated to providing top-notch mathematical statistics homework help. Our experts offer personalized help with mathematical statistics homework, ensuring you receive accurate and detailed support. With their extensive knowledge, our mathematical statistics homework helpers are committed to helping you excel in your assignments and understanding complex concepts. Explore their expertise to see how they can assist you.
Dr. Clara Mitchell
Ph.D. in Quantitative Methods
🇦🇺 Australia
Dr. Clara Mitchell earned her Ph.D. in Quantitative Methods from the University of Guelph. With 760 homework completed and over 10 years of experience, she specializes in delivering precise and actionable statistical analyses. Dr. Mitchell is known for her ability to handle both basic and advanced topics with efficiency and clarity.
MR. Aakash Patel
Master’s in Applied Statistics
🇮🇳 India
MR. Aakash Patel obtained his Master’s in Applied Statistics from the University of Delhi. With over 500 homework completed and 7 years of experience, he is highly regarded for his ability to simplify complex topics and provide structured solutions. His consistent track record makes him a top choice for social science statistics homework help.
Professor Fisher Griffiths
Master’s degree in Statistics
🇦🇺 Australia
Professor Fisher Griffiths holds a Master’s degree in Statistics from Aberystwyth University. With over 420 homework completed and 8 years of experience, he is known for his practical and hands-on approach. His focus on delivering thorough and detailed solutions makes him a trusted resource for students in need of reliable assistance.
Dr. Lila Monroe
Ph.D. in Statistics
🇦🇪 United Arab Emirates
Dr. Lila Monroe earned her Ph.D. in Statistics from the College of William & Mary. She has completed over 350 homework during her 9 years of experience, helping students master complex statistical concepts. Dr. Monroe’s expertise lies in delivering clear and concise solutions to intricate problems across various domains of social science statistics.
Mr. Dylan Reese
Master’s degree in Statistics
🇸🇬 Singapore
Mr. Dylan Reese holds a Master’s degree in Statistics from Cardiff University. With 930+ homework on his belt, Mr. Dylan brings 6 years of experience to the table. His professional background includes contributions at Aberystwyth University and the University of South Wales, specializing in statistical applications and data interpretation.
Dr. Amelia Hayes
Ph.D. in Statistics
🇸🇬 Singapore
Dr. Amelia Hayes completed her Ph.D. in Statistics at Simon Fraser University. Over her 7-year career, she has tackled 930+ homework, drawing on her expertise gained at Trent University and Mount Royal University. Her work involves advanced statistical techniques and practical applications for diverse data sets.
Professor Oliver Langley
MSc in Statistics
🇬🇧 United Kingdom
Professor Oliver Langley obtained his MSc in Statistics from the University of Leicester. With more than 810+ homework completed, he has 10 years of academic and practical experience. He has contributed significantly to the field through his work at the University of Essex and Keele University, focusing on various statistical methodologies.
Dr. Evelyn Hawthorne
Ph.D. in Statistics
🇺🇸 United States
Dr. Evelyn Hawthorne holds a Ph.D. in Statistics from the University of California, Irvine. She has completed over 620 homework and brings over 8 years of experience in the field. Her career includes roles at Claremont McKenna College and Occidental College, where she refined her skills in statistical analysis and data interpretation.
Professor James Sullivan
Ph.D. in Statistics
🇺🇸 United States
Professor James Sullivan, with a Ph.D. from Cardiff University, has completed more than 770 statistical consulting projects. His extensive background in statistical methods and his commitment to high-quality work make him a trusted expert in the field. Professor Sullivan’s experience spans various statistical domains, ensuring that students receive well-rounded and effective assistance.
MR. Lin Wei
Master’s in Statistics
🇨🇦 Canada
MR. Lin Wei holds a Master’s in Statistics from the University of Toronto. With over 420 homework completed, he brings a practical, solution-oriented approach to statistical consulting. His commitment to accuracy and his ability to handle complex datasets make him an excellent choice for students seeking reliable and insightful help with their statistical homework.
Professor Ravi Patel
M.Sc. in Applied Statistics
🇬🇧 United Kingdom
Professor Ravi Patel earned his M.Sc. in Applied Statistics from the University of Alberta. With a track record of over 400 completed homework, Professor Patel is renowned for his methodical approach and in-depth understanding of statistical techniques. His extensive experience enables him to tackle diverse and challenging statistical problems with confidence and skill.
Dr. Isla Montgomery
Ph.D. in Statistics
🇨🇦 Canada
Dr. Isla Montgomery holds a Ph.D. in Statistics from the University of Wales Trinity Saint David. With over 350 homework completed, Dr. Montgomery combines deep theoretical knowledge with practical experience. Her expertise ensures precise, data-driven solutions for complex statistical problems. Her dedication and attention to detail have earned her a reputation for excellence in the field.
Professor Elwood Tiller
Master’s degree in Industrial Statistics
🇸🇬 Singapore
Professor Elwood Tiller holds a Master’s degree in Industrial Statistics from Williams College. He has completed more than 1,900 homework and brings a decade of experience to his role. Professor Tiller’s expertise lies in applying advanced statistical techniques to real-world problems, ensuring students receive thorough, actionable insights that enhance their understanding.
Dr. Rosalind Glynne
Ph.D. in Industrial Statistics
🇬🇧 United Kingdom
Dr. Rosalind Glynne earned her Ph.D. in Industrial Statistics from the University of Bristol. With over 1,450 homework completed and 8 years of experience, Dr. Glynne offers expert solutions tailored to complex statistical problems. Her approach combines rigorous analysis with a clear, methodical presentation to meet the highest academic standards.
MR. Liam Connolly
Master’s in Industrial Statistics
🇺🇸 United States
MR. Liam Connolly, with a Master’s in Industrial Statistics from Lakehead University, has managed over 1,300 homework. His 6 years of professional experience are characterized by a commitment to accuracy and clarity in every solution he provides. MR. Connolly’s skill set includes a range of statistical methods tailored to meet diverse academic needs.
Dr. Catrin Evans
Ph.D. in Industrial Statistics
🇦🇪 United Arab Emirates
Dr. Catrin Evans received her Ph.D. from Aberystwyth University in Industrial Statistics. With over 860 homework completed and 7 years of expertise, Dr. Evans specializes in providing detailed, reliable solutions for intricate statistical challenges. Her work is known for its precision and the ability to convey complex concepts in an accessible manner.
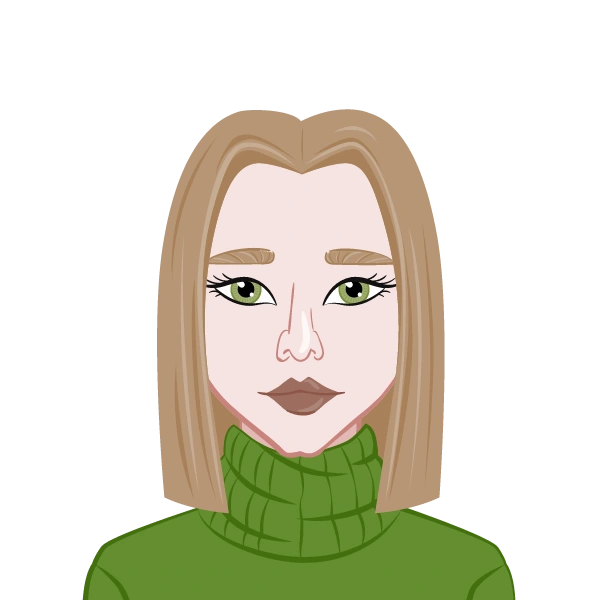
Dr. Lena Zhao
Ph.D. in Economics
🇨🇦 Canada
Dr. Lena Zhao received her Ph.D. in Quantitative Methods from the University of Toronto. With over 630 homework under her belt, she possesses extensive experience and a thorough understanding of business statistics. Dr. Zhao is celebrated for her ability to simplify complex topics and her dedication to providing precise, high-quality solutions. Her work ensures that students not only complete their homework but also improve their grasp of the subject. Specialization Areas:
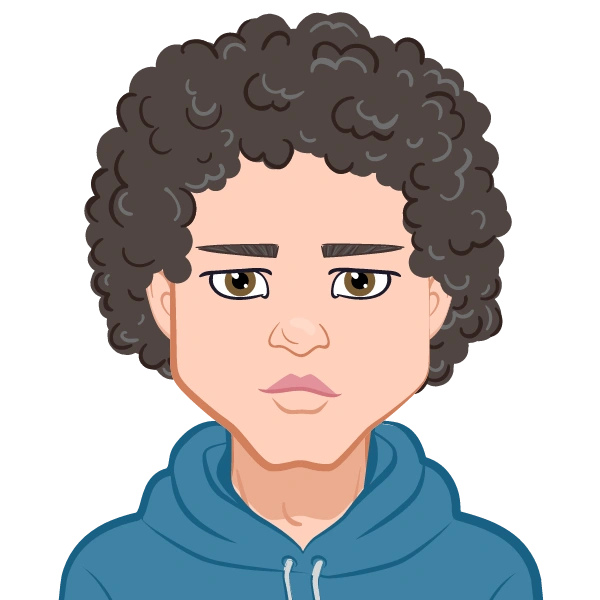
MR. Rohan Patel
Master’s degree in Applied Statistics
🇺🇸 United States
MR. Rohan Patel holds a Master’s degree in Applied Statistics from the University of Chicago. He has completed over 1,310 homework, bringing a wealth of experience in business data analysis and statistical modeling. His focus is on delivering practical and understandable solutions, guiding students through their homework effectively. MR. Patel’s expertise encompasses both academic research and applied data techniques.
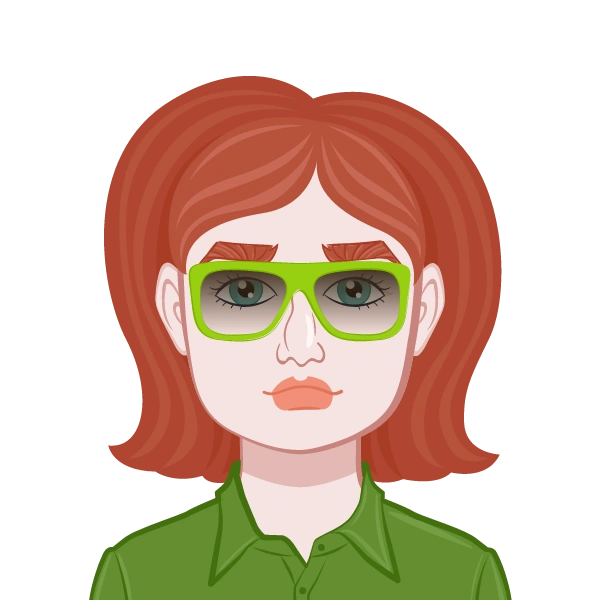
Professor Emily Chen
Ph.D. in Economics
🇺🇸 United States
Professor Emily Chen earned her Ph.D. in Statistics from the University of Chicago and has completed over 1,160 homework throughout her career. With extensive teaching and research experience, Professor Chen brings in-depth knowledge in business statistics and data analysis. Her approach merges rigorous theoretical understanding with hands-on problem-solving techniques, helping students master complex concepts and their applications.
.webp)
Dr. Alex Morgan
Ph.D. in Business Analytics
🇺🇸 United States
Dr. Alex Morgan holds a Ph.D. in Business Analytics from Stanford University. With over 940 homework completed, Dr. Morgan has more than a decade of experience assisting students with complex statistical concepts and analyses. His expertise extends beyond theoretical applications into real-world business scenarios, ensuring that students gain both knowledge and practical skills. Dr. Morgan is renowned for his clear explanations and commitment to fostering student success.
Related Topics
Frequently Asked Questions (FAQs)
Our FAQ section provides answers to common queries about our services. Find out how our mathematical statistics homework helpers deliver exceptional help with mathematical statistics homework and what you can expect from their support. For additional information on getting the best mathematical statistics homework help, browse through our frequently asked questions to get all the details you need.