Claim Your Discount Today
Get 10% off on all Statistics homework at statisticshomeworkhelp.com! Whether it’s Probability, Regression Analysis, or Hypothesis Testing, our experts are ready to help you excel. Don’t miss out—grab this offer today! Our dedicated team ensures accurate solutions and timely delivery, boosting your grades and confidence. Hurry, this limited-time discount won’t last forever!
We Accept
- Understanding Statistical Software
- Key Considerations in Choosing Statistical Software
- Nature of Your Homework: Tailoring Tools to Tasks
- Learning Curve: Balancing Familiarity and Complexity
- Features and Capabilities: Meeting Analysis Requirements
- Data Input and Compatibility: Seamless Data Handling
- Cost: Budgeting for Your Software
- Community and Support: Tapping into Resources
- Integration: Streamlining Workflows
- Platform Compatibility: Ensuring Accessibility
- Exploring Popular Statistical Software Options
- R: A Playground for Statisticians and Data Scientists
- Python: Where Versatility Meets Data Analysis
- SPSS: User-Friendly Analysis for Social Sciences and Beyond
- SAS: Advanced Analytics and Data Management
- Excel: Widely Accessible Yet Limited
- Conclusion
In the realm of data analysis, statistical software plays a pivotal role in transforming raw data into meaningful insights, allowing users to complete their statistics homework with ease. From researchers and analysts to business professionals and students, statistical software empowers users to explore data, uncover patterns, make informed decisions, and ultimately contribute to the advancement of knowledge. However, with a plethora of options available in the market, selecting the right statistical software for your homework can be a daunting task. This comprehensive guide aims to walk you through the key considerations and popular choices in the world of statistical software.
Understanding Statistical Software
Statistical software is designed to facilitate data analysis, visualization, and interpretation by providing a suite of tools and algorithms that streamline complex calculations and transform data into understandable formats. Whether you're dealing with surveys, experiments, or observational data, statistical software helps you summarize, model, and draw conclusions from the information at hand.
Key Considerations in Choosing Statistical Software
Selecting the right statistical software is a pivotal decision that can significantly impact the outcome of your data analysis tasks. To ensure you make an informed choice, it's crucial to consider several key factors before diving into the specifics of available software options.
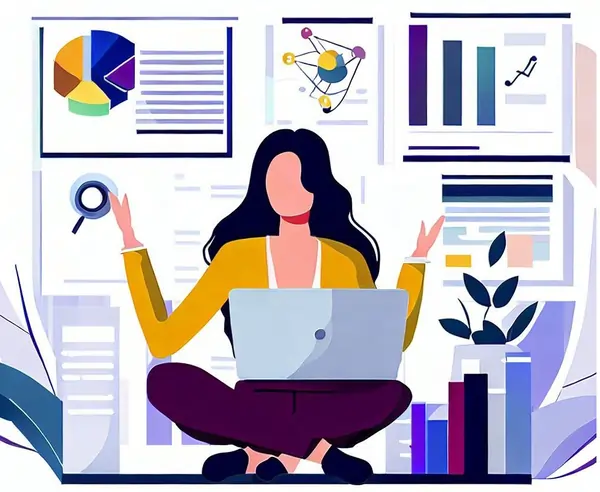
Nature of Your Homework: Tailoring Tools to Tasks
The first and foremost consideration is understanding the nature of your homework. Different tasks demand different types of statistical analyses. Consider whether you're primarily dealing with descriptive statistics to summarize data, inferential statistics to draw conclusions from a sample, regression analysis to model relationships, or data visualization to communicate insights. Aligning the software's capabilities with your homework requirements is paramount to success.
Learning Curve: Balancing Familiarity and Complexity
Your familiarity with statistical software plays a pivotal role in your choice. Some tools are designed with user-friendliness in mind, making them accessible to beginners with minimal statistical or programming knowledge. Others may require a steeper learning curve, demanding a more substantial investment of time and effort. Assess your own proficiency and the resources available for learning to find the right balance between user-friendliness and complexity.
Features and Capabilities: Meeting Analysis Requirements
Evaluate the software's range of features and capabilities to ensure they align with your analytical needs. Consider the following aspects:
- Statistical Techniques: Does the software offer the statistical techniques required for your homework? It should encompass everything from basic tests to advanced modeling.
- Dataset Size: Can the software handle the size of datasets you'll be working with? For large-scale data analysis, robust handling of substantial data volumes is crucial.
- Data Visualization: If visualization is essential, check if the software provides interactive and customizable visualization options, as effective data communication is often as critical as the analysis itself.
Data Input and Compatibility: Seamless Data Handling
Efficient data handling is a cornerstone of statistical analysis. Ensure that the software can smoothly manage the data formats you'll be working with, whether it's spreadsheets, databases, or specialized file formats. Compatibility with your data sources and formats is vital to prevent data import or export issues that can impede your workflow.
Cost: Budgeting for Your Software
Statistical software spans a wide range of pricing models. Some options, like R and Python, are open-source and free of charge, while others require a substantial financial investment. Consider your budget constraints and the long-term cost implications, including potential licensing fees, subscription costs, or the need for additional modules or plugins.
Community and Support: Tapping into Resources
Access to support and a thriving user community can be invaluable, especially when encountering challenges during your analysis. Investigate whether the software has an active user base, online forums, and reliable customer support channels. The availability of documentation, tutorials, and troubleshooting resources can make a significant difference in your experience.
Integration: Streamlining Workflows
Consider whether the software can seamlessly integrate with other tools in your analytical workflow. Integration with programming languages like R or Python can be beneficial, allowing you to leverage the strengths of multiple tools and libraries. Compatibility with other software or platforms you regularly use can enhance efficiency.
Platform Compatibility: Ensuring Accessibility
Before finalizing your choice, verify that the software runs smoothly on your operating system, whether it's Windows, macOS, Linux, or others. Compatibility issues can disrupt your workflow and lead to unnecessary frustrations.
In summary, selecting the right statistical software requires a thoughtful assessment of your homework demands, your own proficiency, the software's capabilities, data compatibility, budget considerations, available support, integration possibilities, and platform compatibility. By carefully considering these factors, you can make an informed choice that empowers you to conduct effective and insightful data analysis.
Exploring Popular Statistical Software Options
When it comes to statistical analysis, having the right tool can make all the difference. Let's take a closer look at some of the most popular statistical software options available today, each with its own unique strengths and weaknesses.
R: A Playground for Statisticians and Data Scientists
R is a robust and versatile programming language and software environment designed specifically for statistical computing and graphics. With its open-source nature, R has gained immense popularity in academia and research communities, as well as among data scientists and statisticians. Here are the pros and cons of using R:
Pros:
- Strong Statistical Capabilities: R boasts a comprehensive library of statistical techniques, from basic descriptive statistics to advanced machine learning algorithms.
- Active Community and Package Ecosystem: The R community is vibrant, contributing to an extensive package ecosystem that covers virtually every statistical analysis imaginable.
- Custom Analyses and Data Visualization: R enables users to create custom analyses and data visualizations tailored to their specific needs, making it a favorite among researchers.
- Cross-Platform Compatibility: R runs on various operating systems, ensuring broad accessibility.
Cons:
- Steeper Learning Curve: R's power comes with a learning curve, especially for non-programmers who might find its syntax challenging at first.
- Limited Documentation for Some Packages: While many packages have thorough documentation, some might lack comprehensive guides, requiring users to explore more to understand their functionality.
Python: Where Versatility Meets Data Analysis
Python, a general-purpose programming language, has become increasingly popular for data analysis and manipulation due to its simplicity and versatility. Libraries like NumPy, pandas, and SciPy provide powerful statistical capabilities. Here's a closer look at Python's strengths and limitations:
Pros:
- General-Purpose Language with Strong Libraries: Python's wide-ranging applications extend to data analysis, and libraries like NumPy (for numerical computations) and pandas (for data manipulation) make it a potent choice.
- Scientific and Engineering Communities: Python is a favorite among scientists and engineers for its rich ecosystem of tools that cater to various disciplines.
- Integration of Analysis with Code and Explanations: Jupyter notebooks enable users to combine code, visualizations, and explanatory text in a single document, facilitating clear communication of findings.
- Cross-Platform Compatibility: Python runs on multiple platforms, enhancing accessibility.
Cons:
- Less Specialized for Statistics: While Python is proficient in handling statistics, it might lack some specialized statistical functionalities that dedicated software provides.
- Additional Libraries for Advanced Analyses: Certain advanced statistical analyses might require additional libraries, potentially increasing the complexity of the analysis process.
SPSS: User-Friendly Analysis for Social Sciences and Beyond
SPSS (Statistical Package for the Social Sciences) stands out as a user-friendly option tailored for statistical analysis in social sciences and business contexts. Its approachable interface and versatile capabilities make it a popular choice:
Pros:
- Intuitive User Interface: SPSS's user-friendly interface is designed to make statistical analysis accessible to those with limited programming or technical expertise.
- Wide Range of Statistical Analyses: SPSS covers a broad spectrum of statistical techniques, making it suitable for a variety of analyses.
- Data Manipulation and Transformation: SPSS facilitates data manipulation and transformation, allowing users to prepare data for analysis efficiently.
Cons:
- Commercial Software: SPSS comes with a cost, which might not be feasible for individuals or organizations with tight budgets.
- Limited Flexibility for Advanced Users: While SPSS is great for standard analyses, it might be less accommodating for advanced users seeking custom or complex analyses.
- Scripting and Automation Limitations: Compared to programming languages, SPSS has limitations when it comes to scripting and automation.
SAS: Advanced Analytics and Data Management
SAS (Statistical Analysis System) is a comprehensive software suite renowned for its capabilities in advanced analytics, multivariate analysis, business intelligence, and data management. It's often utilized in industries that prioritize data security and reliability:
Pros:
- Broad Statistical Capabilities: SAS offers a wide range of statistical and data analysis capabilities, making it suitable for complex analyses.
- Large-Scale Data Analysis: SAS excels in handling large datasets and complex models, a crucial feature for industries dealing with massive amounts of information.
- Emphasis on Data Security and Compliance: SAS places a strong emphasis on data security and compliance, appealing to industries with strict regulations.
Cons:
- Commercial Software with a Price Tag: SAS's advanced features come at a significant cost, which might be a deterrent for individual users or smaller organizations.
- Limited Popularity in Academic and Open-Source Communities: While SAS is widely used in certain industries, it might not enjoy the same popularity in academic or open-source circles.
- Learning Curve: SAS's extensive capabilities might result in a steeper learning curve, especially for newcomers to the software.
Excel: Widely Accessible Yet Limited
Microsoft Excel, although not exclusively designed for statistical analysis, remains a widely accessible tool that many individuals and organizations use for basic data analysis tasks. Let's examine its advantages and drawbacks:
Pros:
- Familiarity and Widespread Usage: Virtually everyone is familiar with Excel, and it's readily available as part of the Microsoft Office suite.
- Beginner-Friendly: Excel's interface is user-friendly, making it a suitable choice for beginners and small-scale analyses.
- Basic Statistical Functions and Charts: Excel includes basic statistical functions and charting options, which can suffice for simple analyses.
Cons:
- Limited Statistical Capabilities: Compared to specialized statistical software, Excel's capabilities are limited, particularly for advanced or specialized analyses.
- Challenges with Large Datasets and Complexity: Excel can become unwieldy when dealing with large datasets or complex analyses, potentially leading to errors.
- Not Ideal for Sophisticated Tasks: While Excel can handle basic statistics, it might fall short when dealing with more sophisticated statistical tasks.
Conclusion
Choosing the right statistical software for your homework depends on a variety of factors, including the nature of your analysis, your level of expertise, the features you require, and your budget. Each software option has its own strengths and weaknesses, so it's important to weigh these against your specific needs. Whether you opt for the open-source power of R, the versatility of Python, the user-friendliness of SPSS, the advanced capabilities of SAS, or the familiarity of Excel, remember that the goal is to effectively analyze data and extract valuable insights to support your research, decision-making, or business objectives. Ultimately, the best statistical software is the one that empowers you to transform data into knowledge.