Claim Your Discount Today
Get 10% off on all Statistics homework at statisticshomeworkhelp.com! Whether it’s Probability, Regression Analysis, or Hypothesis Testing, our experts are ready to help you excel. Don’t miss out—grab this offer today! Our dedicated team ensures accurate solutions and timely delivery, boosting your grades and confidence. Hurry, this limited-time discount won’t last forever!
We Accept
- Descriptive Statistics:
- Theoretical Probability:
- Sampling Methods:
- Hypothesis Testing:
- Analysis of Regression:
- Experimental Design
- Analysis of Time Series:
- Statistical Analysis Software:
- Conclusion:
Applied statistics is an intriguing field that involves the application of statistical techniques to real-world problems. Having a solid foundation in the fundamental topics of applied statistics is critical whether you are a student studying statistics or a professional working in a data-driven industry. In this blog from statistics homework helper, we will look at the most important topics to learn and understand before tackling your applied statistics homework. You will be better equipped to analyze data, draw meaningful conclusions, and make informed decisions if you master these concepts.
Descriptive Statistics:
Descriptive statistics employ a variety of measures to summarize and describe data. The mean, also known as the average, is a central tendency measure that represents the average value of a dataset. It is computed by adding all of the values together and dividing by the total number of observations. Another measure of central tendency is the median, which represents the middle value in a dataset when arranged in ascending or descending order. The median, unlike the mean, is unaffected by extreme values, making it a reliable measure for skewed distributions.
In contrast, mode represents the most frequently occurring value in a dataset. It can be used to determine the dominant category or value in categorical data. The difference between the maximum and minimum values in a dataset is represented by a range, which is a measure of variability. The spread or dispersion of data points around the mean is indicated by variance and standard deviation. Standard deviation is the square root of the variance and is a more interpretable measure of dispersion than variance.
Descriptive statistics also includes graphical techniques such as histograms, box plots, and scatter plots in addition to these measures. Histograms depict the distribution of numerical data by segmenting the range and displaying the frequency or relative frequency of observations within each segment. Box plots show the minimum, first quartile, median, third quartile, and maximum values to provide a visual summary of the distribution. Scatter plots are useful for identifying patterns or trends by visualizing the relationship between two continuous variables.
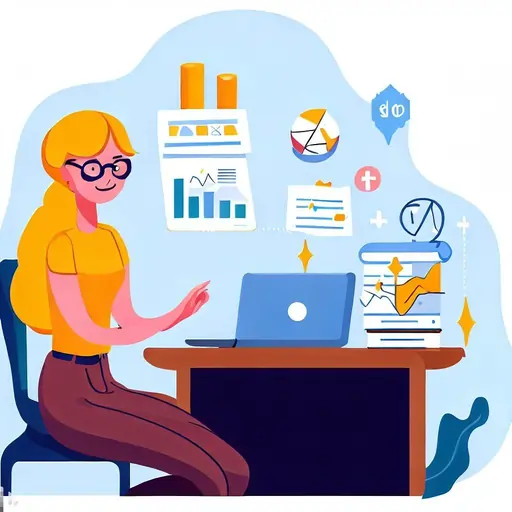
Theoretical Probability:
Probability theory, which deals with the quantification of uncertainty, is the foundation of statistical inference. It is concerned with the investigation of random events and the likelihood of their occurrence. A probability distribution describes the probabilities of various outcomes or events. The normal distribution, binomial distribution, Poisson distribution, and exponential distribution are all examples of common probability distributions.
One of the most important probability distributions in statistics is the normal distribution, also known as the bell curve. Its symmetric shape and the fact that the mean, median, and mode are all equal distinguish it. A normal distribution is used to describe many natural phenomena, making it a fundamental concept in applied statistics. In contrast, the binomial distribution is used to model the number of successes in a fixed number of independent Bernoulli trials. It's especially useful when dealing with binary outcomes like success/failure or yes/no questions.
Understanding probability laws is essential in probability theory. The probability of the union of two mutually exclusive events is equal to the sum of their individual probabilities, according to the addition rule. The multiplication rule, on the other hand, states that the likelihood of two independent events intersecting is equal to the product of their individual probabilities. In various statistical scenarios, these rules serve as the foundation for calculating probabilities and making predictions.
Sampling Methods:
Sampling techniques are used to select a subset of people or observations from a larger group. The goal is to obtain a representative sample that accurately reflects the population's characteristics. The most basic sampling technique is simple random sampling, in which each member of the population has an equal chance of being chosen. This method ensures that every person has an equal chance of being included in the sample.
Another common technique is stratified sampling, which involves dividing the population into distinct groups or strata based on certain characteristics. To ensure representation, a proportional or disproportionate sample is drawn from each stratum. Stratified sampling is especially useful when there are different subgroups in the population and we want to ensure that each subgroup is adequately represented in the sample.
Cluster sampling entails dividing the population into clusters or groups and selecting entire clusters at random for inclusion in the sample. When it is impractical or expensive to sample individuals directly, this method is useful. Cluster sampling is commonly used in surveys where the clusters are geographical regions or institutions.
Systematic sampling is a technique in which individuals are drawn at random from an ordered list at regular intervals. If a population list is sorted alphabetically, for example, every nth individual can be chosen to form the sample. If the list is randomly ordered or does not exhibit any patterns, this method is simple to implement and can provide a representative sample.
Hypothesis Testing:
A statistical procedure that allows us to make inferences about a population based on sample data is hypothesis testing. Formulating a null hypothesis (H0) and an alternative hypothesis (Ha), selecting an appropriate test statistic, determining the significance level, collecting data, calculating the test statistic, and interpreting the results are all part of the process.
The null hypothesis is the default assumption or claim that no significant difference or relationship exists between variables in the population. In contrast, the alternative hypothesis asserts that there is a significant difference or relationship. The significance level, commonly abbreviated as, determines the cutoff for rejecting the null hypothesis. Common significance levels are 0.05 and 0.01, which correspond to a 5% and 1% chance of rejecting the null hypothesis, respectively, when it is true.
The test statistic is calculated based on the data collected to assess the likelihood of obtaining the observed result if the null hypothesis is true. The test statistic chosen is determined by the type of data and the research question. The t-test, chi-square test, ANOVA (analysis of variance), and correlation coefficient are all examples of test statistics.
The results are interpreted by comparing the calculated test statistic to the critical value or p-value. The critical value is determined by the significance level chosen as well as the distribution of the test statistic. If the calculated test statistic is greater than the critical value, the null hypothesis is rejected in favor of the alternative hypothesis. Alternatively, if the p-value is less than the significance level, the null hypothesis is rejected.
Based on statistical evidence, hypothesis testing allows us to draw conclusions, evaluate claims, and make informed decisions. It's widely used in research, quality control, medical trials, and a variety of other applications.
Analysis of Regression:
Regression analysis is a statistical technique for modeling the relationship between one or more independent variables and a dependent variable. It seeks to comprehend how changes in the independent variables influence the dependent variable and to make predictions based on the observed data.
One of the most common regression techniques is linear regression. It is based on the assumption of a linear relationship between the dependent and independent variables. The goal is to estimate the linear equation coefficients that best fit the data. The coefficients represent the dependent variable's slope or rate of change with respect to the independent variables.
Multiple regression incorporates multiple independent variables into linear regression. It enables us to evaluate each independent variable's unique contribution while controlling for the effects of other variables. Multiple regression models are commonly used to study complex relationships in the social sciences, economics, and business.
When the dependent variable is binary or categorical, another type of regression analysis is used. Based on the independent variables, it models the likelihood of the dependent variable belonging to a specific category. In medical research, social sciences, and marketing, logistic regression is widely used to predict binary outcomes such as disease presence/absence or customer churn.
The interpretation of regression coefficients is a critical aspect of regression analysis. The coefficients represent the magnitude and direction of the relationship between the variables. A positive relationship is indicated by positive coefficients, while a negative relationship is indicated by negative coefficients. While holding other variables constant, the magnitude of the coefficient represents the change in the dependent variable associated with a one-unit change in the independent variable.
The goodness of fit of a regression model is critical in determining its effectiveness. R-squared, also known as the coefficient of determination, calculates how much of the variance in the dependent variable can be explained by the independent variables. A higher R-squared value indicates that the model fits the data better.
Understanding regression analysis allows you to discover relationships, predict outcomes, and make data-driven decisions in a variety of fields such as economics, finance, social sciences, and marketing.
Experimental Design
When conducting experiments or studies to test hypotheses and make causal inferences, experimental design is critical. The validity and reliability of the results are ensured by proper design. Experiment design involves a number of key concepts and techniques.
In experimental design, randomization is a fundamental principle. It entails randomly assigning participants or subjects to different treatment groups in order to minimize bias and ensure that the groups are comparable. Randomization aids in the control of confounding variables and increases the likelihood that any differences observed between groups are due to the treatment or intervention.
In experimental design, control groups are critical. They act as a control group that does not receive the treatment or intervention under investigation. Researchers can assess the effectiveness or impact of a treatment by comparing the outcomes of the treatment group to those of the control group.
When studying the effects of multiple independent variables on a dependent variable, a factorial design is used. A factorial design combines different levels of each independent variable to create various treatment combinations. This enables researchers to investigate the primary effects of each variable as well as any interactions between them.
Blocking is a technique used in experiments to reduce the impact of nuisance variables or sources of variability. It entails grouping similar subjects or units together and assigning treatments at random within each block. Blocking reduces the variability associated with the nuisance variables, allowing the treatment effects to be more visible.
Understanding experimental design principles aids researchers in effectively planning studies, controlling for potential biases, and drawing reliable conclusions. When designing experiments, it is critical to consider factors such as sample size, randomization, control groups, factorial designs, and blocking.
Analysis of Time Series:
Time series analysis is the process of analyzing data over time to identify patterns, trends, and relationships. Time series data are observations made at regular intervals, such as daily, monthly, or yearly data points. Time series analysis employs a variety of statistical techniques to comprehend and forecast future values.
Time series analysis relies heavily on autocorrelation. It calculates the relationship between an observation and its lag values. Positive autocorrelation denotes a pattern of increasing or decreasing values over time, whereas negative autocorrelation denotes an alternating pattern of values.
In time series analysis, moving averages are commonly used to smooth out fluctuations and identify underlying trends. A moving average computes the average of a fixed number of consecutive observations, providing a more complete picture of the data's overall pattern.
The technique of exponential smoothing is used to forecast future values in time series data. It weights past observations differently, with more recent observations receiving higher weights. To make accurate predictions, exponential smoothing methods consider both the trend and the level of the time series.
ARIMA (autoregressive integrated moving average) models, which capture both the autoregressive and moving average components of the data, and seasonal decomposition, which separates the time series into trend, seasonal, and residual components, are also used in time series analysis.
Time series analysis is widely used in a variety of fields such as finance, economics, meteorology, and sales forecasting. You can uncover temporal patterns, forecast future values, and make informed decisions based on historical data if you understand the techniques and concepts involved.
Statistical Analysis Software:
The ability to use statistical software is required for applied statistics. Several powerful statistical packages that provide tools for data manipulation, analysis, and visualization are widely used in the field.
R is a popular open-source statistical programming language with a large number of statistical analysis packages. It includes a wide range of functions for data cleaning, exploration, hypothesis testing, regression analysis, and other tasks. R also has powerful visualization tools, allowing users to create high-quality graphs and charts.
Python has gained popularity in data analysis and machine learning thanks to libraries such as NumPy and Pandas. Python is a popular choice for data manipulation, statistical modeling, and algorithm development due to its versatility and simplicity. NumPy offers efficient numerical computing, whereas Pandas provides high-performance data structures and data analysis tools.
SAS (Statistical Analysis System) is a commercial software suite that is widely used for advanced analytics and statistical modeling. It offers a comprehensive set of statistical procedures as well as data management tools. SAS is especially common in industries like healthcare, finance, and market research.
Statistical software packages have user-friendly interfaces and a wide range of functions that make complex statistical analyses easier to understand. They enable users to import and manipulate data, perform statistical analysis, generate reports, and create visualizations. Expertise in statistical software allows researchers and analysts to work efficiently with large datasets, perform advanced analyses, and effectively communicate results.
Conclusion:
Mastering the top topics in applied statistics is critical for completing your homework and building a solid foundation in the field. You will have the tools to analyze data, draw meaningful conclusions, and make informed decisions if you understand descriptive statistics, probability theory, sampling techniques, hypothesis testing, regression analysis, experimental design, time series analysis, and statistical software.
Remember that these topics serve as the foundation for more advanced statistical methods and applications. Continuously practicing and applying these concepts will improve your statistical skills and allow you to tackle more complex problems in the field of applied statistics with confidence.