Navigating the Maze: Correlation and Causation in SPSS Homework
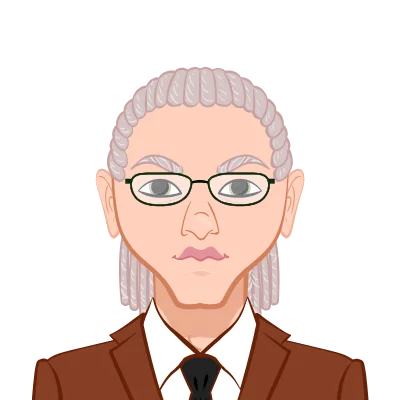
Embarking on the journey of SPSS homework is akin to stepping into a labyrinth of statistical intricacies. As students navigate this maze, they encounter a myriad of statistical tools that can either illuminate the path or cast shadows of confusion. Among the many statistical techniques housed within SPSS, the understanding of correlation and causation emerges as a beacon of paramount importance. These concepts act as the guiding light, helping students make sense of the intricate relationships between variables in their data sets. The complexity of SPSS, with its vast array of functions and options, can be overwhelming for students, especially those new to statistical analysis. It's not merely about inputting data and running tests; it's about deciphering the results, interpreting the relationships, and drawing meaningful conclusions. In this landscape, correlation and causation stand out as fundamental pillars that support the entire structure of statistical reasoning.
Correlation, in the context of SPSS, is the statistical measure of the relationship between two variables. It reveals the degree to which changes in one variable are associated with changes in another. This statistical tool is invaluable for understanding patterns and trends in data. However, it's crucial for students to grasp that correlation does not imply causation. The relationship between variables might be coincidental or influenced by an external factor not considered in the analysis. Acknowledging this nuance is the first step in avoiding common pitfalls in statistical reasoning. Solve your SPSS homework with a clear understanding of correlation, recognizing that correlation alone does not establish a cause-and-effect relationship. Causation, on the other hand, is the holy grail of statistical analysis. Establishing a causal relationship implies that changes in one variable directly lead to changes in another. While SPSS provides tools to explore causation, students must approach this concept with caution. Experimental designs, such as randomized controlled trials, play a pivotal role in establishing causation, offering a structured framework to draw meaningful conclusions from statistical analyses.
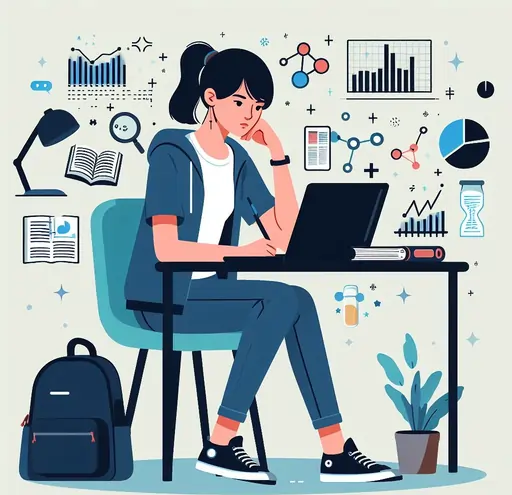
The Basics of Correlation in SPSS
Understanding the basics of correlation in SPSS is akin to unlocking the gateway to deciphering relationships within datasets. In the vast landscape of statistical analysis, correlation stands out as a pivotal technique, shedding light on the strength and direction of linear relationships between two variables. For students delving into SPSS assignments, grasping these fundamentals is not just advisable; it's imperative.
Grasping the Concept of Correlation
Correlation, within the realm of SPSS, is a statistical method designed to quantify the extent of association between two variables. The essence lies in revealing whether, and to what degree, changes in one variable correspond to changes in another. Picture this: you're exploring survey data, and you want to understand if there's a relationship between hours of study and exam scores. Correlation, through its various coefficients, helps you unveil the story hidden within the numbers.
SPSS, being a powerful statistical software, simplifies the calculation of correlation coefficients. Among these, Pearson's correlation coefficient takes center stage. This coefficient, denoted as 'r,' encapsulates the strength and direction of a linear relationship. A positive 'r' indicates a positive correlation, signifying that as one variable increases, the other also tends to increase. Conversely, a negative 'r' implies a negative correlation, suggesting that as one variable increases, the other tends to decrease.
Interpreting Correlation Output in SPSS
Once you've ventured into the labyrinth of SPSS to conduct your correlation analysis, you're met with a deluge of output. Amidst the statistical jargon and numerical values, your focus should zero in on two crucial elements: the correlation coefficient (r) and its significance level, commonly represented as the p-value. The correlation coefficient (r) is your compass in understanding the strength and direction of the relationship. A value close to 1 or -1 indicates a robust correlation, while values approaching 0 signify a weaker connection. However, it's imperative to tread cautiously. Although a high correlation coefficient suggests a strong relationship, it is crucial to remember that correlation does not imply causation.
Accompanying the correlation coefficient is the p-value, a measure of the statistical significance of the correlation. A small p-value (typically less than 0.05) suggests that the observed correlation is unlikely to be a result of random chance. However, statistical significance does not equate to practical significance. Exercise caution and refrain from making causation assumptions solely based on correlation results. Always keep in mind the cardinal rule: correlation is not causation.
Untangling Causation: A Deeper Dive
Navigating the realm of causation in SPSS requires a profound understanding of statistical inference, as causation is often regarded as the holy grail of such analyses. Unlike correlation, which merely establishes relationships between variables, causation delves into the intricate idea that one variable has a direct impact on causing changes in another. This concept forms the core of many research endeavors, aiming to unearth the fundamental drivers behind observed phenomena.
In the context of SPSS assignments, grappling with causation becomes a nuanced task. The first and foremost lesson for students is the indispensable recognition that correlation does not automatically imply causation. This cautionary note is fundamental, as drawing causal conclusions based solely on observed correlations can lead to erroneous interpretations. It's a pitfall that even seasoned researchers can stumble into if not approached with due diligence.
Understanding Causation in SPSS
Understanding causation in SPSS involves acknowledging its complexity and the methodological rigor it demands. Causation, in essence, requires establishing a cause-and-effect relationship between two variables. However, SPSS assignments, often constrained by observational data, make establishing causation particularly intricate.
Students must grasp that while correlation identifies associations, it does not elucidate the direction of influence. Recognizing this limitation is vital, prompting the need for meticulous research design and methodologies. In the labyrinth of SPSS, causation is not a straightforward path; it demands intellectual rigor and a keen eye for potential confounders.
Exploring Experimental Designs in SPSS
In the pursuit of causation within SPSS, students find refuge in the realm of experimental designs. These designs provide a structured framework that allows researchers to manipulate variables and observe the resultant changes systematically. Randomized Controlled Trials (RCTs) and quasi-experimental designs stand out as powerful tools within this landscape. Randomized Controlled Trials, often considered the gold standard, involve randomly assigning participants to different groups and manipulating an independent variable to observe its effect on a dependent variable. Quasi-experimental designs, though lacking the randomization element, still offer valuable insights by carefully selecting and matching groups based on certain criteria.
Mastering SPSS tools tailored for experimental analysis becomes paramount in disentangling causation from correlation. These tools empower students to not only perform the necessary statistical tests but also to understand the intricacies of experimental design, ensuring that their causal claims are grounded in robust methodology.
Common Pitfalls in Correlation and Causation
Navigating the intricate world of correlation and causation in SPSS demands not only a keen understanding of statistical concepts but also a vigilant eye for common pitfalls that can derail your analysis. Let's delve into two significant pitfalls: avoiding spurious correlations and identifying correlation-causation fallacies.
Avoiding Spurious Correlations in SPSS
Spurious correlations, those seemingly meaningful relationships between variables that are actually coincidental, add a layer of complexity and often amusement to statistical analysis. Picture bizarre correlations like the per capita consumption of cheese correlating with the number of people who died by becoming tangled in their bedsheets. While amusing, such correlations underscore the need for caution in drawing conclusions. In SPSS assignments, students must cultivate a heightened sense of skepticism. Careful scrutiny of variables is paramount. It involves questioning the relevance and logical connection between variables that might suggest a correlation. Additionally, students should be mindful of lurking variables that could confound the correlation. Lurking variables are external factors that may impact the relationship between the variables under study, leading to a misleading interpretation. For instance, the correlation between ice cream sales and drowning incidents may be confounded by a lurking variable like temperature.
To steer clear of spurious correlations, meticulous attention to detail is crucial. In SPSS, this involves not only running correlation analyses but also understanding the context of the variables being examined. A robust understanding of the subject matter can help students identify potential lurking variables and differentiate between a meaningful correlation and a coincidental one.
Identifying Correlation-Causation Fallacies
Correlation-causation fallacies represent another minefield in the realm of SPSS assignments. The trap lies in assuming that a correlation between two variables implies a causal relationship. In the context of SPSS, students must be vigilant not to fall into this common misconception. Critical thinking becomes the shield against correlation-causation fallacies. In SPSS assignments, students should adopt a meticulous approach to data analysis, understanding that correlation does not imply causation. For instance, if a study reveals a strong correlation between the number of swimming pool drownings and ice cream sales, it does not mean that buying more ice cream causes more drownings or vice versa. The correlation might be coincidental, or a lurking variable like summer heat could be influencing both variables independently.
To sidestep these fallacies, students must exercise caution when interpreting their SPSS results. Correlation provides a valuable insight into relationships, but establishing causation requires a more rigorous examination, often involving experimental designs and additional statistical techniques. By embracing critical thinking and maintaining a vigilant approach, students can navigate the intricate landscape of correlation and causation in SPSS with confidence and accuracy.
Practical Tips for SPSS Homework Success
Navigating the intricate landscape of SPSS assignments requires more than just theoretical knowledge. Success in SPSS homework is often contingent on a practical and systematic approach. By adopting the following tips, students can enhance their proficiency in correlation and causation analysis, ensuring a smooth journey through the complexities of statistical research.
Embrace a Systematic Approach to SPSS Assignments
When faced with an SPSS assignment, it's crucial to embrace a systematic workflow. The first step is to clearly define your research question. This lays the foundation for your entire analysis, guiding the subsequent stages of your SPSS assignment. Once the question is crystal clear, the next task is to identify the variables involved. Understanding which variables will be analyzed is pivotal, as it shapes the statistical tests you'll employ. After defining the research question and identifying variables, the spotlight turns to selecting the appropriate statistical tests. In correlation and causation analysis, this step is paramount. Different relationships call for different tests, and SPSS offers a plethora of options. Whether it's Pearson's correlation for linear relationships or more advanced tests for causation, a systematic approach ensures you choose the right tool for the job.
By adopting a step-by-step approach, you not only enhance your understanding of the SPSS tools at your disposal but also lay the groundwork for robust results. This systematic method minimizes the risk of oversight, ensuring that each facet of your analysis receives the attention it deserves. In the labyrinth of SPSS, a systematic approach is your compass, guiding you through the intricacies and complexities with confidence.
Leverage SPSS Tutorials and Resources
The expansive functionalities of SPSS can be overwhelming, especially for those new to statistical analysis. To surmount this hurdle, students should actively leverage the plethora of online tutorials, manuals, and forums dedicated to SPSS. Online tutorials serve as virtual mentors, guiding students through the intricacies of SPSS functionalities. These tutorials often break down complex processes into digestible steps, providing clarity on how to execute specific analyses within SPSS. Manuals, whether in the form of official SPSS documentation or supplementary guides, offer in-depth explanations of SPSS features, making them indispensable companions in your learning journey.
Forums dedicated to SPSS create virtual communities where students can seek assistance, share insights, and troubleshoot challenges. Engaging with these communities provides not only solutions to specific problems but also a broader perspective on how others approach and solve similar issues. Real-world examples shared on these platforms enhance practical understanding, bridging the gap between theoretical knowledge and hands-on application.
Conclusion:
In conclusion, mastering correlation and causation in SPSS is pivotal for students navigating the intricate terrain of statistical analysis. This blog serves as a compass, guiding you through the fundamentals, potential pitfalls, and practical strategies to excel in your SPSS homework. Armed with knowledge and a systematic approach, you'll not only decipher the complexities but also unlock the true power of SPSS in your academic journey.