Claim Your Discount Today
Get 10% off on all Statistics homework at statisticshomeworkhelp.com! Whether it’s Probability, Regression Analysis, or Hypothesis Testing, our experts are ready to help you excel. Don’t miss out—grab this offer today! Our dedicated team ensures accurate solutions and timely delivery, boosting your grades and confidence. Hurry, this limited-time discount won’t last forever!
We Accept
- Understanding Factor Analysis
- Steps to Conduct Factor Analysis
- Step 2: Data Collection and Preparation
- Step 3: Choose the Factor Analysis Method
- Step 4: Perform Factor Analysis
- Step 5: Interpret the Results
- Step 6: Make Inferences
- Tips for Accurate Factor Analysis
- 1. Sample Size Matters
- 2. Examine the Correlation Matrix
- 3. Understand the Common Factor Model
- 4. Prioritize Meaningful Interpretation
- 5. Rotation Techniques
- 6. Be Mindful of Multicollinearity
- 7. Consider Cross-Validation
- 8. Replicate the Analysis
- 9. Seek Expert Guidance
- Conclusion
Statistics is a powerful tool used to make sense of complex data, and factor analysis is one of the techniques within its arsenal. Factor analysis is a method used to uncover underlying patterns in a dataset by identifying relationships among variables. It's commonly used in fields such as psychology, social sciences, market research, and more. In this blog, we'll walk you through the process of conducting and interpreting factor analyses for your statistics homework. Whether you need assistance with your factor analysis homework or are simply looking to enhance your understanding of this valuable statistical technique, we've got you covered.
Understanding Factor Analysis
Factor analysis aims to explain the variance between observed variables in terms of a smaller number of latent (unobserved) variables called factors. These factors represent the common underlying dimensions that contribute to the observed data. By reducing the dimensionality of the data, factor analysis simplifies complex relationships and aids in identifying meaningful patterns.
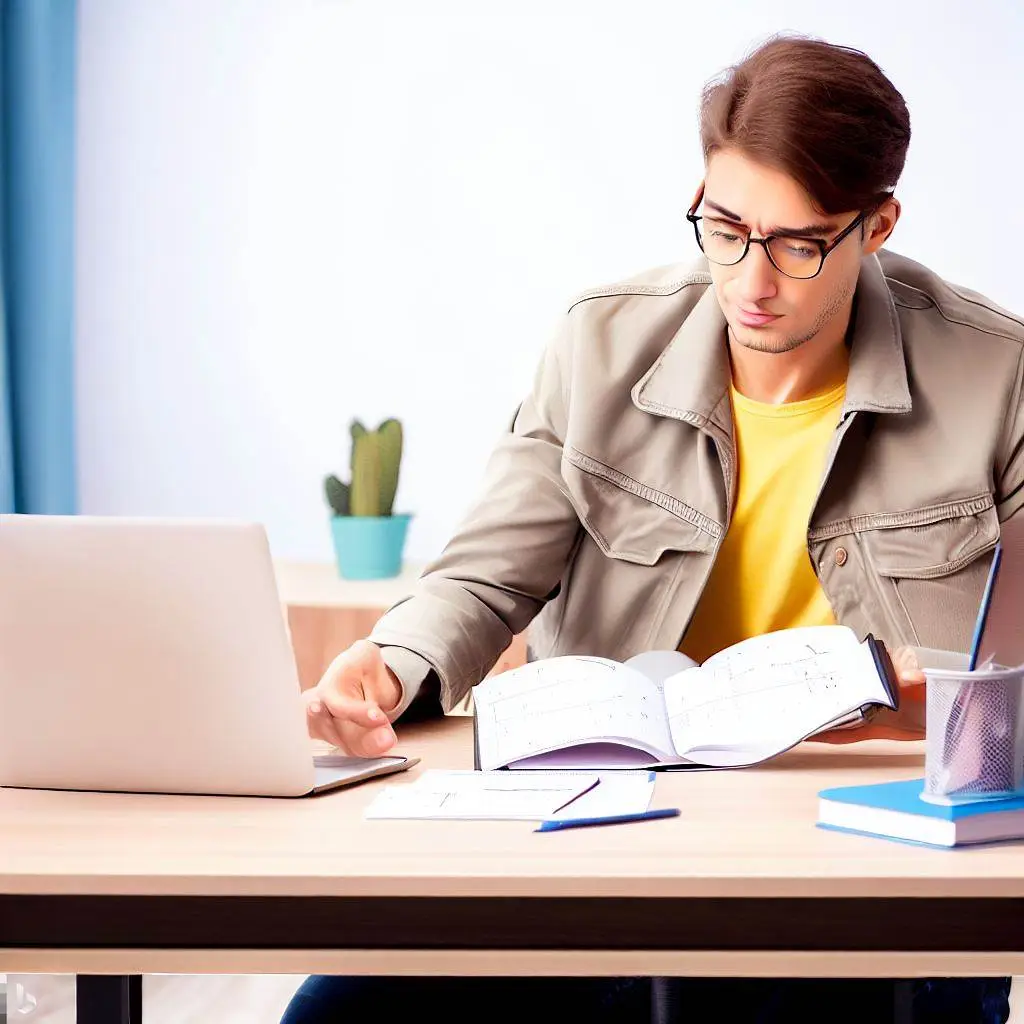
Steps to Conduct Factor Analysis
Factor analysis is a complex statistical technique that involves multiple stages to extract meaningful insights from your data. By following these steps, you can effectively conduct factor analysis and gain a deeper understanding of the underlying patterns in your dataset.
Step 1: Formulate Your Hypothesis
A successful factor analysis begins with a clear research hypothesis or question. Define what relationships or underlying dimensions you aim to uncover among the variables in your dataset. This initial step helps guide your analysis and ensures that the results are relevant to your research objectives.
Step 2: Data Collection and Preparation
The success and accuracy of your factor analysis hinge upon the quality of your data. Proper data collection and thorough preparation are essential to ensure that your results are meaningful, reliable, and aligned with the assumptions of the technique. Here's a detailed exploration of the factors to consider during data collection and preparation:
Variable Types
Factor analysis is most effective when applied to continuous data, particularly data measured on interval or ratio scales. These scales offer meaningful numerical values with equal intervals, allowing for meaningful comparisons between observations. Continuous data ensures that the relationships among variables are captured accurately.
However, if your dataset includes categorical variables, applying standard factor analysis might not be appropriate. Categorical data lacks the numerical properties required by factor analysis algorithms. In such cases, special techniques like categorical factor analysis, which are designed for categorical variables, can be employed. These techniques adapt factor analysis methods to suit the nature of your categorical data.
Missing Data
Addressing missing data is crucial to prevent bias and distortion in your factor analysis results. Missing values can lead to inaccurate estimates of factor loadings and, consequently, flawed interpretations. Several approaches can be taken to handle missing data:
- Imputation: Impute missing values using statistical methods to estimate their plausible values. Common imputation techniques include mean imputation, regression imputation, and k-nearest neighbors imputation.
- Listwise Deletion: Remove entire cases (observations) with missing values from the analysis. However, this approach can lead to reduced sample size and potentially biased results if the missing data are not missing completely at random.
- Multiple Imputation: This advanced technique involves creating multiple imputed datasets to account for uncertainty in missing data. Factor analysis is then performed on each dataset, and results are combined to provide a more robust analysis.
Outliers
Outliers, or extreme values, have the potential to significantly distort the results of factor analysis. These data points can influence factor loadings and contribute to inaccurate interpretations. Therefore, it's essential to identify and appropriately handle outliers before conducting factor analysis:
- Visual Exploration: Visualize your data using scatter plots, box plots, or histograms to identify potential outliers. Outliers often stand out as data points far from the main cluster.
- Winsorization: Winsorization involves capping extreme values at a certain percentile (e.g., 1st and 99th percentiles) to mitigate their impact on the analysis.
- Data Transformation: Transforming your data using mathematical functions like logarithms or square roots can sometimes help reduce the impact of outliers. However, be cautious about the interpretability of transformed data.
Data Normalization
In addition to addressing missing data and outliers, normalizing your data can be beneficial for factor analysis. Normalization ensures that variables with different scales and units are on a comparable basis, preventing larger-scaled variables from disproportionately influencing the analysis.
- Z-Score Normalization: Transforming your variables into z-scores (subtracting the mean and dividing by the standard deviation) standardizes them to have a mean of 0 and a standard deviation of 1.
- Min-Max Scaling: Scaling variables to a specified range (e.g., 0 to 1) ensures they have similar ranges, aiding in meaningful comparison.
Step 3: Choose the Factor Analysis Method
Selecting the appropriate factor analysis method is critical to the success of your analysis. The two common methods are:
- Principal Component Analysis (PCA): PCA aims to explain the maximum variance in the data using linear combinations of variables. It's suitable when you're more interested in explaining variance than in uncovering latent factors.
- Exploratory Factor Analysis (EFA): EFA focuses on identifying the underlying factors that explain correlations between observed variables. This method is more suitable when you want to uncover latent dimensions driving the relationships.
Step 4: Perform Factor Analysis
- Decide on the Number of Factors: Based on your research question and dataset, determine how many factors to extract. Statistical methods like the Kaiser-Guttman criterion or scree plot analysis can help guide this decision.
- Choose Extraction Method: Select an extraction method to identify the initial factors. The Kaiser criterion, for instance, suggests retaining factors with eigenvalues greater than 1. Alternatively, you can use data-driven methods like parallel analysis to determine the number of factors.
- Interpret the Factor Loadings: Factor loadings indicate the strength and direction of the relationships between variables and factors. Higher factor loadings imply stronger associations. A loading of 0.5 or higher is often considered meaningful, but this threshold can vary based on your context.
- Analyze Eigenvalues: Eigenvalues reflect the amount of variance explained by each factor. Higher eigenvalues suggest more important factors. Be cautious of over-extracting factors with eigenvalues close to 1, as they might represent noise rather than meaningful structure.
Step 5: Interpret the Results
Interpreting the results is where the true value of factor analysis lies. Make sense of the extracted factors by:
- Factor Loadings: Examine the factor loadings to identify which variables are strongly associated with each factor. Naming factors based on variables with high loadings can provide insight into their meaning.
- Eigenvalues: Consider eigenvalues to assess the significance of each factor. Factors with eigenvalues well above 1 contribute significantly to explaining variance in the data.
- Scree Plot: Create a scree plot by plotting eigenvalues against factor numbers. Identify the "elbow point," where eigenvalues start to level off. Factors before this point are usually retained.
- Rotation: If you used a rotation method, interpret the rotated factor loadings. Rotation enhances interpretability by minimizing the number of variables with high loadings on each factor.
Step 6: Make Inferences
Based on your interpretation of the factors, draw meaningful inferences that align with your research hypothesis or question. Relate the factors back to the context of your study and consider how they contribute to the understanding of your data.
Factor analysis is a comprehensive process that involves careful planning, data preparation, method selection, and interpretation. By following these steps, you can navigate the complexities of factor analysis and gain valuable insights into the underlying dimensions that drive the relationships among your variables. Remember that factor analysis requires a combination of statistical expertise and domain knowledge to ensure accurate and meaningful results.
Tips for Accurate Factor Analysis
Factor analysis is a powerful statistical technique, but its accuracy and effectiveness can be greatly influenced by several key factors. To ensure that your factor analysis produces meaningful and reliable results, consider the following tips:
1. Sample Size Matters
The size of your dataset plays a crucial role in the accuracy of factor analysis. A common rule of thumb is to have a larger sample size relative to the number of variables you're analyzing. Insufficient sample sizes can lead to unstable factor solutions and unreliable results. With a larger sample size, the patterns in the data are more likely to be consistent and reflective of the underlying population.
2. Examine the Correlation Matrix
Factor analysis assumes that variables are correlated with each other, as it aims to identify underlying shared variance. Before conducting factor analysis, it's essential to assess the correlation matrix among your variables. Variables with low or near-zero correlations may not be suitable for factor analysis, as they might not contribute to the common factor structure. Strong correlations, on the other hand, suggest that the variables could potentially share common underlying dimensions.
3. Understand the Common Factor Model
The common factor model is the foundation of factor analysis. It assumes that each observed variable is influenced by a combination of common factors and unique factors specific to that variable. These unique factors are often referred to as "error" or "unique variance." Understanding this model helps you appreciate that factor analysis aims to separate the common underlying dimensions from the unique variations associated with each variable.
4. Prioritize Meaningful Interpretation
Interpreting the results of factor analysis is both an art and a science. While the mathematical outputs provide information about factor loadings and eigenvalues, the true value of factor analysis lies in the meaningful interpretation of these results. It's important to align the extracted factors with your research question, theoretical framework, or the context of the study. If the factors do not make conceptual sense or do not fit the narrative of your study, re-evaluate the analysis and consider adjusting the number of factors or rotation methods.
5. Rotation Techniques
Factor rotation is a critical step that simplifies the interpretation of the factors. Rotation aims to transform the factor loadings in a way that makes the structure more interpretable. Techniques like Varimax, Promax, and Oblimin are commonly used for this purpose. The choice of rotation method can impact the clarity of the results, so try different methods and assess which one best aligns with the conceptual meaning of your factors.
6. Be Mindful of Multicollinearity
Multicollinearity occurs when two or more variables in your dataset are highly correlated with each other. While correlation is a fundamental assumption of factor analysis, extremely high correlations between variables can lead to challenges in interpreting the results. It can also cause instability in factor extraction. If you encounter severe multicollinearity, consider either removing one of the highly correlated variables or using techniques like Principal Component Analysis (PCA) to address the issue.
7. Consider Cross-Validation
Cross-validation involves splitting your dataset into multiple subsets for analysis. This can help validate the stability of your factor structure across different samples. If your factors are consistent across various subsets of the data, it provides more confidence in the robustness of your findings.
8. Replicate the Analysis
To ensure the reliability of your results, consider replicating the factor analysis using similar datasets or different subsets of your data. Replication adds an extra layer of confidence in the stability and consistency of the extracted factors.
9. Seek Expert Guidance
Factor analysis can be complex, especially for those new to the technique. If you're uncertain about any step of the process or the interpretation of results, seek guidance from a statistician, mentor, or professor. Consulting with experts can help you avoid common pitfalls and ensure that your analysis is conducted correctly.
Conclusion
Factor analysis is a valuable tool for uncovering hidden patterns within complex datasets. By identifying underlying factors, you can simplify your data and extract meaningful information. Remember that conducting factor analysis requires a clear hypothesis, careful data preparation, and a thorough understanding of the results. With these steps and tips in mind, you're well-equipped to tackle factor analyses for your statistics homework and gain deeper insights into your data.