From Basic Probability to Advanced Analytics: Comprehensive Statistics Homework Help
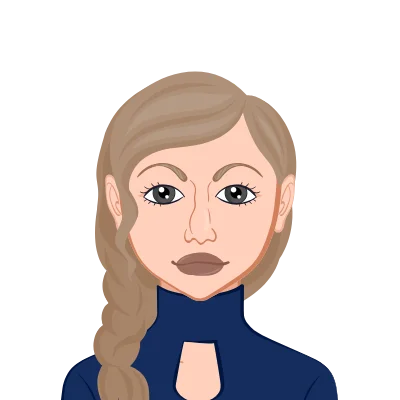
Navigating the intricate landscape of statistics, students embark on a journey that spans from foundational probability concepts to the realms of advanced analytics. The challenges they encounter are diverse, covering a spectrum that extends from understanding the basics of probability theory to applying sophisticated statistical techniques. At its core, statistics is a fundamental field that serves as the bedrock for a myriad of disciplines, permeating fields as diverse as economics, biology, sociology, and beyond. The mastery of statistical principles becomes not just an academic pursuit but a gateway to a vast array of career opportunities. The foundational step in this expedition is often the exploration of basic probability concepts. Probability theory forms the cornerstone upon which more intricate statistical methods are built. It is here that students grapple with the abstraction of uncertainty and randomness, learning to quantify and analyze these phenomena through mathematical models. Concepts such as events, sample spaces, and probability distributions become the building blocks, laying a solid foundation for the statistical odyssey ahead.
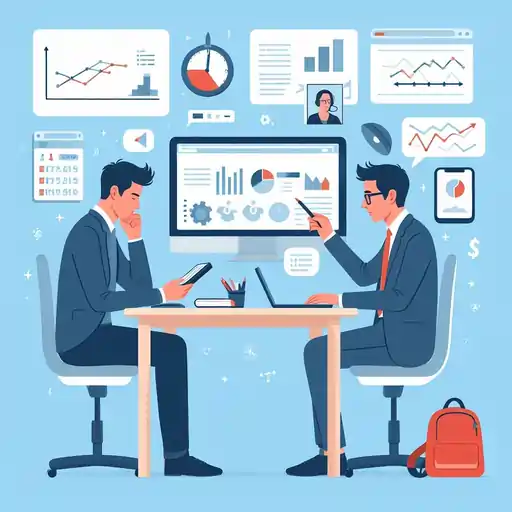
Moving beyond the probabilistic realm, students delve into descriptive statistics. This phase of the journey involves the exploration of measures that characterize and summarize datasets. Mean, median, and mode emerge as tools to unravel central tendencies, providing insights into the core features of data. Simultaneously, variance and standard deviation step forward to quantify the dispersion of data points, essential for a comprehensive understanding. Descriptive statistics, in essence, acts as the lens through which students perceive the intricacies of datasets, an indispensable skill for making informed decisions based on empirical evidence. The transition from descriptive statistics propels students into the domain of inferential statistics. Here, they grapple with hypothesis testing and confidence intervals, techniques designed to draw meaningful conclusions about populations from sample data. This phase requires a shift in perspective, as students move from summarizing observed data to making inferences about broader trends and patterns. Hypothesis testing, with its emphasis on statistical significance, becomes a critical tool for researchers and analysts alike. If you find yourself needing assistance, don't hesitate to seek help with your Statistics homework.
Understanding the Basics of Probability and Statistics
Understanding the basics of probability and statistics is a pivotal step in the journey towards statistical mastery. This foundational knowledge lays the groundwork for more advanced concepts and techniques, serving as the cornerstone for a statistician's toolkit. In this exploration, we delve into the significance of probability and statistical measures, shedding light on their roles in shaping the landscape of statistical analysis.
Probability, at its essence, deals with uncertainty and randomness, providing a systematic way to analyze and predict outcomes. As students embark on their statistical journey, probability emerges as the fundamental concept upon which more advanced methods are built. Early encounters with probability theory occur in the initial stages of statistics coursework, emphasizing its critical role in preparing students for the intricacies of more complex topics.
The Foundation of Probability
The foundation of probability lies in comprehending its core principles. Probability is not merely a mathematical concept; it is a lens through which uncertainty is quantified. This lens becomes indispensable as students progress in their statistical education, offering a structured approach to scenarios ranging from simple coin flips and dice rolls to more intricate statistical problems. Probability theory equips students with essential tools, introducing them to concepts like events, sample spaces, and probability distributions. These concepts form the building blocks for a diverse array of statistical problems, creating a robust foundation for further exploration. As students grapple with the challenges presented by probability, they cultivate the skills needed to navigate the complexities inherent in statistical analysis.
The practical applications of probability are vast and extend beyond academic exercises. From predicting the outcome of a simple coin toss to understanding the likelihood of more complex events, probability becomes a language through which uncertainties are translated into quantifiable measures. Embracing the foundation of probability sets the stage for a more profound understanding of statistical methodologies.
Statistical Measures and Descriptive Statistics
Moving beyond probability, students delve into the realm of descriptive statistics—a set of techniques designed to illuminate the main features of a dataset. Descriptive statistics serve as a lens through which students gain insights into the central tendencies of data. Measures such as mean, median, and mode offer a snapshot of the data's central values, while variance and standard deviation quantify the spread, providing a comprehensive view of the dataset's distribution. Mastery of descriptive statistics is crucial for interpreting data and drawing meaningful conclusions. Whether working with social science data, biological measurements, or economic indicators, a solid grasp of descriptive statistics is indispensable for any statistician. These measures not only summarize the data but also guide the statistician in making informed decisions about the dataset's characteristics.
As students progress in their statistical journey, descriptive statistics become the bridge between basic concepts and more advanced analyses. The ability to succinctly summarize and describe the characteristics of a dataset is a skill that resonates in various domains, from research and academia to industry applications. A thorough understanding of descriptive statistics propels students towards a more nuanced and insightful approach to statistical analysis, setting the stage for further exploration into the intricate realms of inferential statistics and advanced analytics.
Transitioning to Inferential Statistics: Drawing Meaningful Conclusions
The transition to inferential statistics represents a significant milestone in a student's statistical journey, marking a crucial shift from the foundational principles of probability and descriptive statistics to a more complex and nuanced understanding of data analysis. This phase is characterized by the evolution from merely describing data to making educated inferences that extend beyond the confines of a specific dataset, encompassing broader populations.
At the heart of inferential statistics lies the fundamental concept of hypothesis testing—a powerful tool that allows statisticians to draw meaningful conclusions about population parameters using sample data. Hypothesis testing involves formulating a hypothesis about a population parameter, collecting sample data, and then assessing the likelihood of the observed results under the assumption that the null hypothesis is true. This rigorous process enables statisticians to make informed decisions about the characteristics of entire populations based on the analysis of a representative sample.
Hypothesis Testing and Confidence Intervals
At the core of inferential statistics lies the intricate dance of hypothesis testing and confidence intervals. Hypothesis testing is a powerful tool that enables statisticians to draw conclusions about population parameters from sample data. It involves the formulation of null and alternative hypotheses, statistical testing, and the assessment of significance levels. This process allows researchers to make informed decisions and assert the validity of their findings. Complementing hypothesis testing, confidence intervals offer a nuanced perspective on parameter estimation. Rather than providing a single point estimate, confidence intervals supply a range of plausible values for a population parameter. This acknowledgment of variability adds a layer of sophistication to statistical analyses, recognizing the inherent uncertainty in drawing conclusions from limited sample data.
Understanding these concepts is not merely an academic exercise; it is the gateway to conducting meaningful research and making informed decisions in real-world scenarios. Hypothesis testing and confidence intervals act as bridges, spanning the gap between the specific data under examination and the broader trends and patterns present in the entire population. Students are tasked with applying these statistical methods to navigate the complexities of diverse fields, from social sciences to economics.
Regression Analysis and Correlation
As students venture further into inferential statistics, they encounter the dynamic duo of regression analysis and correlation. These techniques propel statisticians into the realm of predictive modeling, allowing them to explore and quantify relationships between variables. Regression analysis, in particular, enables the creation of models that can predict outcomes based on observed data. The applications of regression analysis and correlation are vast and diverse. From predicting stock prices and studying the impact of variables on disease outcomes to analyzing marketing data, these tools become indispensable in a statistician's arsenal. However, mastering these advanced techniques demands more than a grasp of mathematical foundations; it necessitates the development of critical thinking skills.
Students find themselves challenged by the intricacies of model building, the art of variable selection, and the nuanced task of interpreting results. These challenges are not obstacles but opportunities for growth, pushing students to not only understand statistical methodologies but also to apply them effectively in solving real-world problems. The transition to regression analysis and correlation marks a significant step in a student's statistical journey, transforming them from passive learners to active contributors in the field of statistics.
Embracing Advanced Analytics: A Technological Paradigm Shift
The advent of advanced analytics in the realm of statistics heralds a monumental paradigm shift, catapulting the discipline into a new era of data-driven decision-making. This transformation is not merely a linear progression but a dynamic evolution, shaped by the rapid advancements in technology. As statisticians navigate this ever-evolving landscape, the integration of advanced analytics stands out as a pivotal turning point, redefining the methodologies and strategies employed in unraveling the complexities of data.
In the past, statistics primarily relied on traditional methods to glean insights from data. Descriptive and inferential statistics served as the backbone, providing a systematic framework for analyzing and interpreting information. However, as technology took center stage, the synergy between statistics and advanced analytics emerged as a catalyst for innovation. This integration goes beyond mere augmentation; it signifies a profound shift in the very approach to statistical analysis.
Machine Learning and Predictive Analytics
At the forefront of this technological convergence are machine learning and predictive analytics, powerful tools that empower statisticians to unlock insights from complex datasets. Machine learning algorithms, ranging from traditional methods like classification and clustering to more sophisticated deep learning techniques, are transforming the way statistical analyses are conducted. As statisticians delve into the realm of machine learning, they acquire a deeper understanding of essential concepts such as model training, validation, and evaluation. These skills provide a competitive edge in various industries, including finance and healthcare, where informed decision-making is critical. The ability to harness advanced algorithms enhances a statistician's capability to extract meaningful patterns and predictions from data, paving the way for more accurate and impactful analyses.
The integration of machine learning into statistical practices not only enhances the precision of predictions but also allows for a more nuanced exploration of relationships within datasets. Whether predicting consumer behavior, optimizing manufacturing processes, or identifying potential health risks, machine learning empowers statisticians to navigate the complexities of real-world data with unprecedented accuracy.
Big Data and Analytics in the Real World
In the contemporary data-driven world, statisticians are faced with the monumental task of analyzing vast datasets to extract actionable insights. This brings us to the realm of big data analytics, where the scale and complexity of information require a specialized skill set. Students venturing into this domain are exposed to a diverse toolkit that goes beyond traditional statistical methods. Big data analytics involves not only the processing and interpretation of massive amounts of information but also proficiency in specialized tools and techniques. Platforms like Hadoop and Spark enable statisticians to handle large datasets efficiently, facilitating the extraction of valuable insights. Furthermore, data visualization tools like Tableau empower students to communicate complex findings in a comprehensible manner, bridging the gap between raw data and actionable intelligence.
Understanding big data goes beyond technical proficiency; students must grapple with issues of scalability, data cleaning, and ethical considerations. The implications of working with massive datasets necessitate a holistic approach, prompting statisticians to consider the social, ethical, and privacy dimensions of their analyses. As students navigate this terrain, they not only develop technical skills but also the critical thinking necessary to thrive in a data-centric workforce.
Conclusion
The conclusion encapsulates the profound transformation that students undergo as they navigate the trajectory from basic probability to advanced analytics within the realm of statistics. This journey is not merely an academic exercise but a transformative experience that shapes the way students perceive and interact with data. At its core, this evolution is marked by a continuous accumulation of skills and knowledge, with each step in the progression serving as a building block for the next. The foundational phase of this journey begins with a grasp of the basics of probability. Probability is not merely a theoretical concept; it is the language through which uncertainty and randomness are quantified. As students engage with coin flips, dice rolls, and simple probability distributions, they lay the groundwork for understanding the inherent unpredictability in various real-world scenarios.
This initial exposure instills in them a statistical mindset, a fundamental tool that will serve them well throughout their academic and professional endeavors. Moving beyond the realms of probability, students delve into the intricacies of descriptive and inferential statistics. Descriptive statistics, with measures like mean, median, and standard deviation, become their compass in navigating the vast sea of data. These statistical measures allow students to distill complex datasets into meaningful summaries, providing a foundation for drawing insights and making informed decisions. Inferential statistics, on the other hand, empowers students to extrapolate from sample data to make broader assertions about populations, a crucial skill for anyone engaging in empirical research.