Tips for Conducting a Meta-Analysis in Your Statistics Course
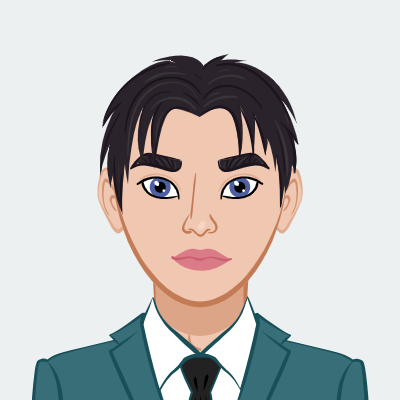
- Understand the Basics
- Define Your Research Question and Inclusion Criteria
- Conduct a Comprehensive Literature Search
- Assess Study Quality
- Extract Data Consistently
- Choose an Effect Size Metric
- Cohen's d: This metric is used to express the standardized mean difference between two groups. It's especially useful when comparing the means of two groups that may have been measured on different scales.
- Odds Ratio: Employed primarily in studies dealing with binary outcomes, the odds ratio quantifies the odds of an event occurring in one group compared to another.
- Correlation Coefficient (r): When your research question revolves around the strength and direction of a linear relationship between two variables, the correlation coefficient becomes an apt choice.
- Risk Ratios: In medical and epidemiological studies, risk ratios are used to assess the relative risk of an outcome between exposed and unexposed groups.
- Calculate Effect Sizes and Variability
- Understand Heterogeneity
- Choose a Meta-Analysis Approach
- Fixed-Effects Model: This assumes that all included studies estimate the same underlying effect size and that any observed variability is due to sampling error. It's suitable when the studies are highly similar in design and methodology.
- Random-Effects Model: This model accounts for both sampling error and true variability between studies. It's more appropriate when there is heterogeneity in effect sizes due to differences in study characteristics.
- Perform Sensitivity Analyses
- Removing Outliers: Excluding studies with extreme effect sizes to assess their influence on the overall result.
- Varying Inclusion Criteria: Testing the impact of including studies with different characteristics.
- Alternative Analytical Approaches: Trying different meta-analysis models or statistical methods to gauge their effect on the results.
- Publication Bias Assessment
- Funnel Plots: Funnel plots are graphical representations that plot the effect sizes of studies against their standard errors. In the absence of publication bias, the points on the plot should resemble a symmetrical funnel shape. Asymmetry or a skewed distribution can indicate potential publication bias.
- Egger's Test: Egger's test quantifies the relationship between the effect size estimates and their corresponding standard errors. If publication bias is present, the test may show a significant correlation between these factors. However, it's important to note that Egger's test can be influenced by other factors as well.
- Interpretation of Results
- Communication and Reporting
- Acknowledge Limitations
- Continuous Learning
Meta-analysis is a powerful statistical technique that involves systematically combining and analyzing data from multiple studies to draw comprehensive conclusions. It's widely used in various fields to synthesize research findings, identify patterns, and provide a more robust understanding of a particular phenomenon. If you're looking to complete your statistics homework on meta-analysis, here are some essential tips to help you navigate the process effectively.
Before embarking on a meta-analysis journey, it's imperative to establish a solid foundation by grasping the basic principles of this statistical technique. A meta-analysis is not just another statistical tool; it's a methodology that enables researchers to aggregate and synthesize findings from multiple individual studies to draw more robust and generalizable conclusions. The primary purpose of a meta-analysis is to provide a quantitative summary of the accumulated evidence, offering a clearer picture of the overall effect size and exploring the potential sources of variability across studies.
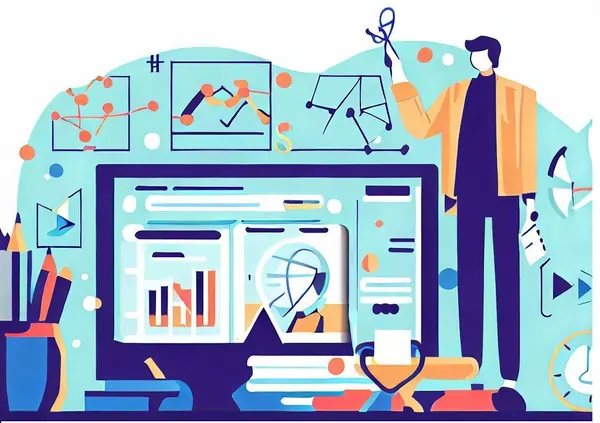
By comprehending the fundamental concepts, you'll be better equipped to appreciate the significance and potential of meta-analysis. It's important to know that meta-analyses are widely used in various fields, including medicine, psychology, education, and social sciences, among others. They serve as a means to distill the noise of individual studies and uncover patterns and trends that might not be evident in single studies.
At the heart of any successful meta-analysis lies a well-defined research question. Before delving into data collection and analysis, it's paramount to articulate a specific, measurable, and relevant research question. This question will act as a guiding beacon throughout the entire meta-analysis process, helping you stay focused on your objectives and ensuring that your efforts lead to meaningful insights.
Equally vital is the establishment of clear inclusion and exclusion criteria. These criteria delineate the characteristics a study must possess to be considered for inclusion in your meta-analysis. These criteria could encompass elements like study design, participant demographics, intervention specifics, and outcome measures. By defining these criteria beforehand, you ensure that your analysis remains consistent and aligned with your research question.
The strength of a meta-analysis lies in its comprehensiveness. To achieve this, conduct an exhaustive literature search across relevant databases, academic journals, conference proceedings, and other reputable sources. Tailor your search strategy to incorporate appropriate keywords, synonyms, and search terms to maximize the breadth of your search.
Remember to document your search strategy meticulously, noting down the databases searched, the keywords used, and the number of identified studies. This documentation not only enhances the transparency of your process but also aids in replication and verification.
The quality of the studies you include significantly impacts the credibility and reliability of your meta-analysis results. As you evaluate potential studies for inclusion, consider factors such as study design, sample size, methodology, and potential sources of bias. A common tool employed for this purpose is the PRISMA checklist, which provides a structured framework for assessing study quality and reporting standards.
Prioritizing high-quality studies contributes to a more accurate and dependable overall effect size estimate. Be prepared to make difficult decisions regarding the inclusion or exclusion of studies based on their quality and relevance to your research question.
Data extraction is a meticulous process that requires attention to detail and consistency. Develop a standardized data extraction protocol to systematically gather relevant information from each study. This protocol should encompass variables such as study characteristics, sample sizes, effect sizes, and measures of variability.
Consistency is paramount during data extraction. Inconsistencies or errors in data collection can undermine the integrity of your meta-analysis. Therefore, ensure that your data extraction process is well-defined, well-documented, and followed rigorously across all studies.
In the realm of meta-analysis, selecting the appropriate effect size metric is pivotal to capturing and quantifying the relationship between variables across multiple studies. The choice of metric hinges on the specific research question at hand and the nature of the data being analyzed. A variety of effect size metrics are at your disposal, each tailored to different types of data and hypotheses.
Some common effect size metrics include:
Picking the most appropriate metric depends on the specific context of your research. Ensure that the chosen metric aligns with your research question, the characteristics of your data, and the statistical assumptions of the chosen effect size measure.
Once the appropriate effect size metric is chosen, the next step is to compute the effect sizes and corresponding measures of variability for each study. The method for calculating effect sizes varies based on the chosen metric and the available data. This might involve extracting raw data (such as means and standard deviations), log odds ratios, or other relevant statistics.
Accuracy and consistency are paramount during this stage. Carefully follow established formulas and double-check your calculations to prevent errors that could compromise the integrity of your meta-analysis.
Heterogeneity is a central consideration in meta-analysis. It refers to the variability in effect sizes across the studies you've included. This variability can stem from differences in study design, populations, interventions, measurement tools, and other factors. Understanding and accounting for heterogeneity is essential to drawing accurate and meaningful conclusions.
There are statistical tests to assess heterogeneity, such as Cochran's Q test and the I² statistic. Cochran's Q test compares the observed differences between study effect sizes and the expected differences under the assumption of homogeneity. The I² statistic quantifies the proportion of total variability that can be attributed to heterogeneity rather than chance.
Based on the level of heterogeneity observed and your research objectives, you'll need to choose between different meta-analysis approaches. Two common models are the fixed-effects model and the random-effects model.
Your choice between these models should be guided by the results of your heterogeneity assessment and the underlying assumptions of each model.
Sensitivity analyses are an essential aspect of a rigorous meta-analysis. They involve testing the robustness of your results by reanalyzing the data under different conditions. Sensitivity analyses can include:
Sensitivity analyses provide insights into the stability of your findings and help identify potential sources of bias or uncertainty.
Publication bias can introduce a significant distortion in the results of a meta-analysis, as studies with non-significant or null findings are less likely to be published. To address this, it's crucial to employ methods to assess and potentially correct for publication bias. Two common approaches are funnel plots and statistical tests like Egger's test.
If you detect significant publication bias, consider adjusting your results using methods like trim-and-fill or fail-safe N analysis to estimate the potential impact of unpublished studies.
Interpreting the results of your meta-analysis requires a comprehensive understanding of the research question, the aggregated evidence, and the potential sources of variability. Begin by discussing the overall effect size estimate and its significance. Is the effect size large enough to be practically meaningful? Is it statistically significant?
Contextualize your findings by considering the accumulated evidence. Are your results consistent with previous research, or do they provide new insights? Address potential sources of heterogeneity that may have influenced the results. Explore variations in study characteristics, methodologies, or participant demographics that could explain differences in effect sizes across studies.
Presenting your meta-analysis findings in a clear and organized manner is vital for communicating your work effectively. Visual aids like forest plots can succinctly illustrate the effect sizes of individual studies and the overall estimate. Ensure that your report includes details about the methods employed, study characteristics, statistical analyses conducted, and any adjustments made for bias or heterogeneity.
Transparent reporting is essential for the reproducibility and credibility of your work. Clearly articulate your research question, the rationale behind your decisions, and the steps you took to address potential biases.
No meta-analysis is without limitations. Engage in a candid discussion of the limitations your study might face. This could include variations in study quality, potential biases introduced by publication bias or study selection criteria, and any assumptions made during the analysis.
Acknowledging limitations showcases your awareness of the potential pitfalls and nuances of your work. It demonstrates a well-rounded understanding of your research and its implications, enhancing the reliability of your conclusions.
Meta-analysis is an evolving field, and staying abreast of the latest methodologies and guidelines is essential. Engage in continuous learning by delving into recent literature, attending workshops, webinars, or seminars focused on meta-analysis, and actively participating in discussions with peers and experts in the field.
By staying curious and open to learning, you'll ensure that your skills and knowledge remain up to date, enabling you to conduct more rigorous and insightful meta-analyses in the future.
Conclusion
Conducting a meta-analysis in your statistics course can be both challenging and rewarding. By understanding the foundational concepts, adhering to rigorous methodologies, and following these essential tips, you can successfully navigate the process. A well-executed meta-analysis can contribute valuable insights to your field of study, offering a synthesized perspective on existing research findings. Remember that practice and continuous learning are key to mastering this advanced statistical technique.