The Ultimate Guide to Excelling in Generalized Linear Modeling Homework

When delving into the world of statistics and data analysis, one topic that often arises is Generalized Linear Modeling (GLM). GLM is a powerful tool used to model relationships between variables, with applications spanning from biology to finance. As you embark on your journey to conquer GLM homework, it's essential to equip yourself with a solid understanding of various topics. In this comprehensive guide, we'll explore the fundamental topics you should know before tackling GLM homework and provide a step-by-step approach to solving Generalized Linear Modeling Homework effectively.
Basics of Linear Regression and Probability
Before diving into GLM, it's crucial to have a solid grasp of linear regression and probability concepts. Linear regression serves as the foundation for GLM, as both models aim to uncover relationships between variables. Understanding concepts like regression coefficients, residuals, and least squares estimation will set the stage for comprehending GLM's extensions.
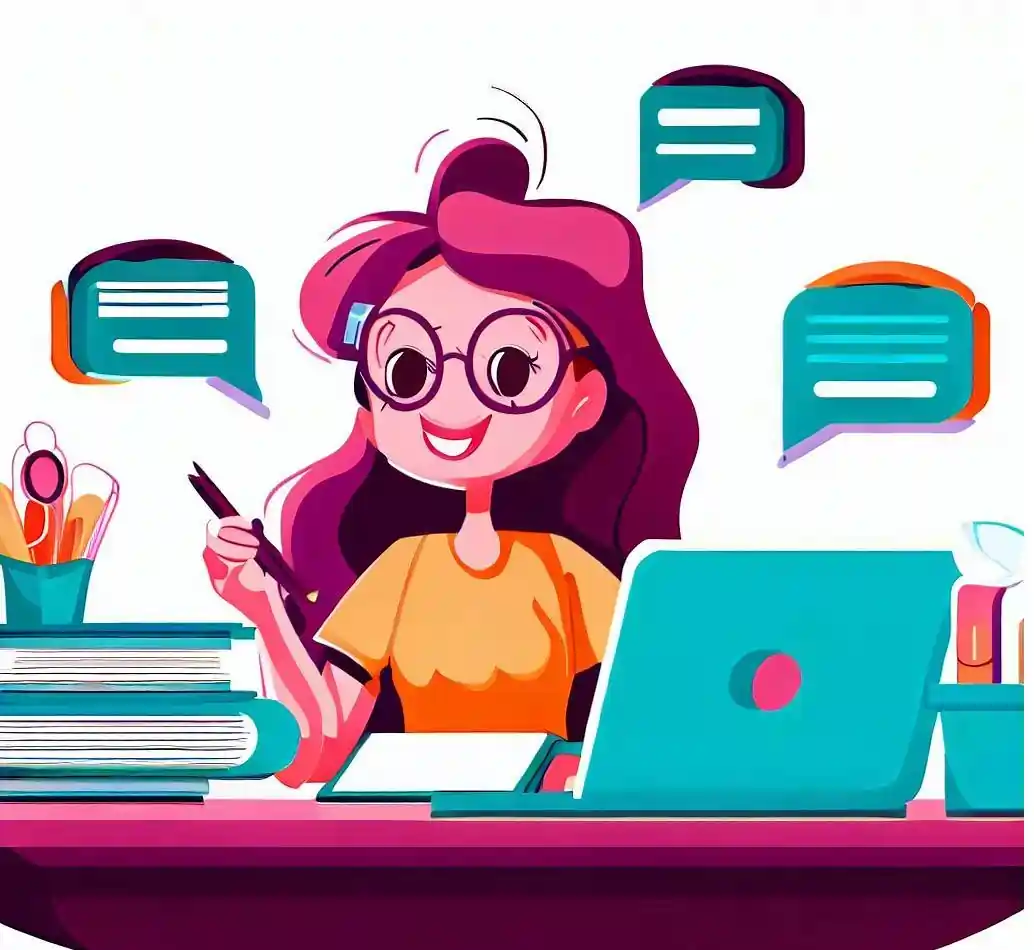
Probability concepts are also essential, particularly when dealing with the likelihood function in GLM. Brush up on concepts such as probability distributions, cumulative distribution functions, and probability density functions. A strong foundation in these areas will make navigating the intricacies of GLM's likelihood function much smoother.
Exponential Family of Distributions
The Exponential Family of Distributions lies at the core of GLM. GLM is designed to handle a wide range of response variables, and the exponential family provides a framework for understanding the underlying distribution of these variables. Familiarize yourself with the exponential family and its various distributions, including the normal, binomial, Poisson, and gamma distributions. Understanding the characteristics and parameters of these distributions will be invaluable when specifying the appropriate model for your GLM homework.
Link Functions and Linear Predictors
In GLM, the relationship between the response variable's mean and the predictors is established through link functions and linear predictors. Different GLMs employ different link functions to connect these components. Common link functions include identity, logit, and log link. Understanding the rationale behind choosing specific link functions and their impact on the model's interpretation is crucial. Dive deep into how to link functions to transform the linear predictor to suit the range and properties of the response variable.
Deviance and Model Goodness of Fit
Assessing the goodness of fit for a GLM involves understanding deviance. Deviance measures the difference between the observed data and the data predicted by the model. A lower deviance indicates a better fit. Delve into the concept of deviance and learn how it's used to evaluate the performance of different GLMs. Familiarize yourself with residual deviance and null deviance and understand how they contribute to the overall assessment of model fit.
Overdispersion and Underdispersion
In some cases, GLMs may encounter overdispersion or underdispersion. Overdispersion occurs when the variance of the response variable is greater than predicted by the model, while underdispersion is the opposite scenario. Both situations can lead to biased parameter estimates and inaccurate inference. Learn to recognize the signs of overdispersion and underdispersion and explore techniques to address these issues. Methods like quasi-likelihood and negative binomial regression can help mitigate the effects of overdispersion.
Model Estimation and Inference
Estimating parameters in GLM involves maximizing the likelihood function. Many software packages offer built-in functions to perform this task, but understanding the underlying optimization process is essential for interpreting results accurately. Additionally, grasp the concept of Wald tests, Likelihood Ratio tests, and Score tests for hypothesis testing. These tools will empower you to draw meaningful conclusions from your GLM homework.
Step-by-Step Approach to Solving GLM Homework
Navigating the realm of Generalized Linear Modeling (GLM) homework requires a systematic approach that combines statistical understanding and practical implementation. In this section, we'll guide you through a concise yet comprehensive step-by-step process, empowering you to confidently tackle GLM problems. By following these steps, you'll not only solve your homework effectively but also develop a deeper grasp of GLM's intricacies.
Mastering the intricacies of Generalized Linear Modeling (GLM) requires a methodical approach that combines theoretical understanding with practical implementation. Let's delve into the step-by-step process that will guide you through solving GLM homework effectively:
- Problem Understanding
- Data Exploration
- Model Specification
- Model Fitting
- Model Assessment
- Interpretation
- Hypothesis Testing
- Reporting Results
At the outset, immerse yourself in the problem statement. Understand the context and objectives of the analysis. Identify the response variable, which represents the outcome you're trying to predict. Pinpoint the predictor variables, often referred to as features or independent variables, which might influence the response. Additionally, pay attention to any specific requirements or constraints outlined in the problem. This comprehensive grasp of the problem will form the foundation for your subsequent steps.
Once you have a clear grasp of the problem, shift your focus to the data itself. Dive into the dataset to gain insights into its characteristics. Explore the distribution of the response variable and predictor variables. Detect potential outliers or anomalies that might impact the modeling process. Identify missing values and consider how to handle them effectively. This exploration serves a dual purpose: it aids in understanding the data's suitability for GLM and informs decisions about preprocessing steps that might be necessary.
With a solid understanding of the data, move on to the heart of GLM: model specification. Based on the nature of the response variable and the predictor variables, select an appropriate distribution from the exponential family. This choice will determine the likelihood function used for estimation. Additionally, choose a suitable link function that establishes the relationship between the linear predictor and the response variable's mean. Define the linear predictor as a combination of predictor variables and their corresponding coefficients. This step is pivotal in laying the groundwork for the modeling process.
Armed with a well-defined model, it's time to fit it into the data. Utilize statistical software such as R or Python to perform the estimation process. The software will iteratively adjust the model's coefficients to maximize the likelihood function, effectively finding the best-fit parameters. As you execute this step, closely examine the output generated by the software. This output includes coefficient estimates, standard errors, and p-values, providing crucial information about the significance of predictor variables and their impact on the response.
Evaluating the model's performance is a critical aspect of the GLM process. One key metric for assessment is deviance, which measures the discrepancy between observed and predicted outcomes. Compare the residual deviance with the null deviance to gauge the model's fit to the data. Furthermore, be vigilant for signs of overdispersion or underdispersion, as they can influence the accuracy of the model's estimates. The robust assessment ensures the model's validity and aids in making informed decisions about model refinement.
With a well-fitted model and a thorough assessment, turn your attention to interpretation. Interpretation involves comprehending the coefficients of the predictor variables in the context of the problem. How does each coefficient influence the response variable based on the chosen link function? Consider the direction and magnitude of these effects. Crafting meaningful interpretations provides insights into the relationships you've uncovered and their practical implications.
In some cases, you may need to substantiate your findings through hypothesis testing. This involves testing the significance of individual coefficients. Utilize statistical tests like Wald tests, Likelihood Ratio tests, or Score tests to evaluate the null hypothesis that a coefficient has no effect. Understand the assumptions underlying these tests and their implications for your conclusions. Hypothesis testing adds a layer of statistical rigour to your analysis.
Having gone through the stages of problem understanding, data exploration, model specification, fitting, assessment, interpretation, and hypothesis testing, it's time to consolidate your findings. Summarize your results in a clear and concise manner. Craft a report that includes information about the chosen model, parameter estimates, goodness of fit metrics, and any hypothesis test results. Effective reporting ensures that your analysis is accessible to others and reinforces your understanding of the GLM process.
Conclusion
Embarking on the journey of mastering Generalized Linear Modeling for your homework might seem challenging, but with a solid foundation in linear regression, probability, and the exponential family of distributions, you'll be well-equipped to tackle the complexities of GLM. Remember the importance of link functions, deviance, and model estimation techniques. By following a structured approach to solving GLM problems, you'll not only complete your homework effectively but also gain a deeper understanding of this powerful statistical tool.